Localization of Thermal Wellbore Defects Using Machine Learning
JOURNAL OF ENERGY RESOURCES TECHNOLOGY-TRANSACTIONS OF THE ASME(2022)
Abstract
Defect detection and localization are key to preventing environmentally damaging wellbore leakages in both geothermal and oil/gas applications. In this study, a multistep, machine learning approach is used to localize two types of thermal defects within a wellbore model. This approach includes a comsol heat transfer simulation to generate base data, a neural network to classify defect orientations, and a localization algorithm to synthesize sensor estimations into a predicted location. A small-scale physical wellbore test bed was created to verify the approach using experimental data. The classification and localization results were quantified using these experimental data. The classification predicted all experimental defect orientations correctly. The localization algorithm predicted the defect location with an average root-mean-square error of 1.49 in. The core contributions of this study are as follows: (1) the overall localization architecture, (2) the use of centroid-guided mean-shift clustering for localization, and (3) the experimental validation and quantification of performance.
MoreTranslated text
Key words
geothermal energy, wells-injection, oil, gas, geothermal
AI Read Science
Must-Reading Tree
Example
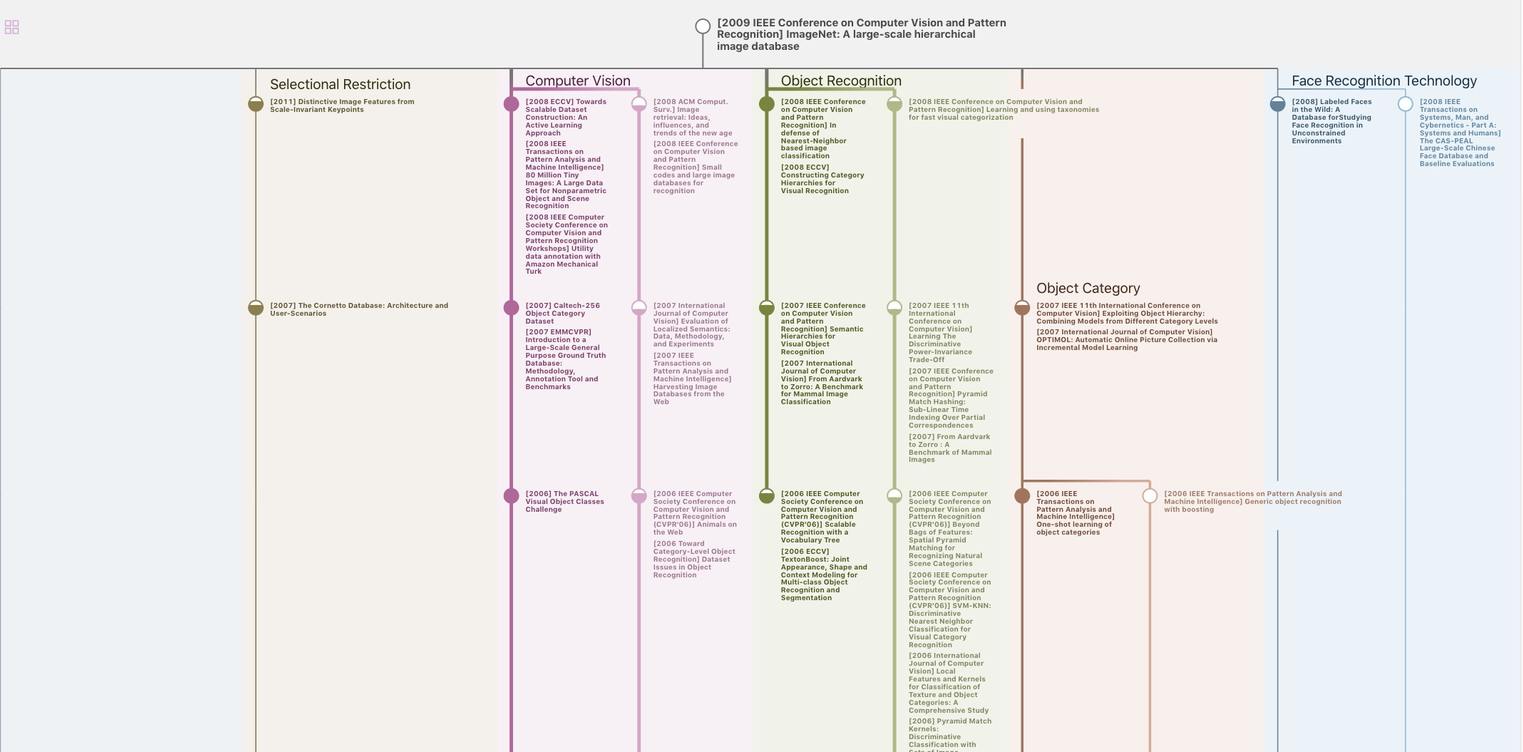
Generate MRT to find the research sequence of this paper
Chat Paper
Summary is being generated by the instructions you defined