Symptom and performance validity in samples of adults at clinical evaluation of ADHD: a replication study using machine learning algorithms
JOURNAL OF CLINICAL AND EXPERIMENTAL NEUROPSYCHOLOGY(2022)
摘要
Introduction Research has shown non-trivial base rates of noncredible symptom report and performance in the clinical evaluation of attention-deficit/hyperactivity disorder (ADHD) in adulthood. The goal of this study is to estimate and replicate base rates of symptom and performance validity test failure in the clinical evaluation of adult ADHD and derive prediction models based on routine clinical measures. Methods This study reuses data of a previous publication of 196 adults seeking ADHD assessment and replicates the findings on an independent sample of 700 adults recruited in the same referral context. Measures of symptom and performance validity (one SVT, two PVTs) were applied to estimate base rates. Prediction models were developed using machine learning. Results Both samples showed substantial rates of noncredible symptom report (one SVT failure: 35.7% - 36.6%), noncredible test performance (one PVT failure: 32.1% - 49.3%; two PVT failures: 18.9% - 27.3%), or both (each one SVT and PVT failure: 13.3% - 22.4%; one SVT and two PVT failures: 9.7% - 13.7%). Machine learning algorithms resulted in generally moderate to weak prediction models, with advantages of the reused sample compared to the independent replication sample. Associations between measures of symptom and performance validity were negligible to small. Conclusions This study highlights the necessity to include measures of symptom and performance validity in the clinical evaluation of adult ADHD. Further, this study demonstrates the difficulty to characterize the group failing symptom or performance validity assessment.
更多查看译文
关键词
Machine learning,symptom validity,attention deficit disorder with hyperactivity,methodology,neuropsychological testing
AI 理解论文
溯源树
样例
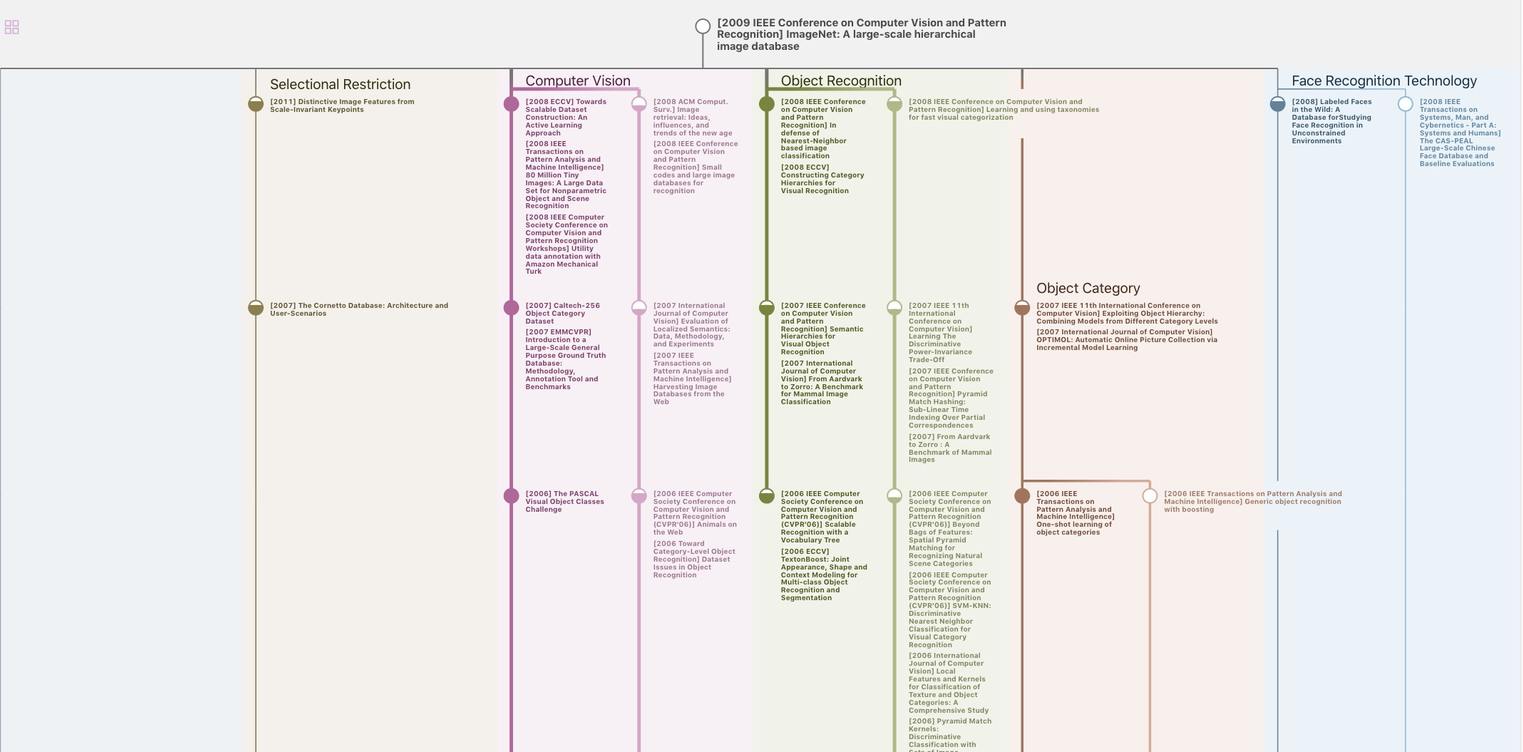
生成溯源树,研究论文发展脉络
Chat Paper
正在生成论文摘要