KGNER: Improving Chinese Named Entity Recognition by BERT Infused with the Knowledge Graph
APPLIED SCIENCES-BASEL(2022)
摘要
Recently, the lexicon method has been proven to be effective for named entity recognition (NER). However, most existing lexicon-based methods cannot fully utilize common-sense knowledge in the knowledge graph. For example, the word embeddings pretrained by Word2vector or Glove lack better contextual semantic information usage. Hence, how to make the best of knowledge for the NER task has become a challenging and hot research topic. We propose a knowledge graph-inspired named-entity recognition (KGNER) featuring a masking and encoding method to incorporate common sense into bidirectional encoder representations from transformers (BERT). The proposed method not only preserves the original sentence semantic information but also takes advantage of the knowledge information in a more reasonable way. Subsequently, we model the temporal dependencies by taking the conditional random field (CRF) as the backend, and improve the overall performance. Experiments on four dominant datasets demonstrate that the KGNER outperforms other lexicon-based models in terms of performance.
更多查看译文
关键词
named-entity recognition,knowledge graph,conditional random field
AI 理解论文
溯源树
样例
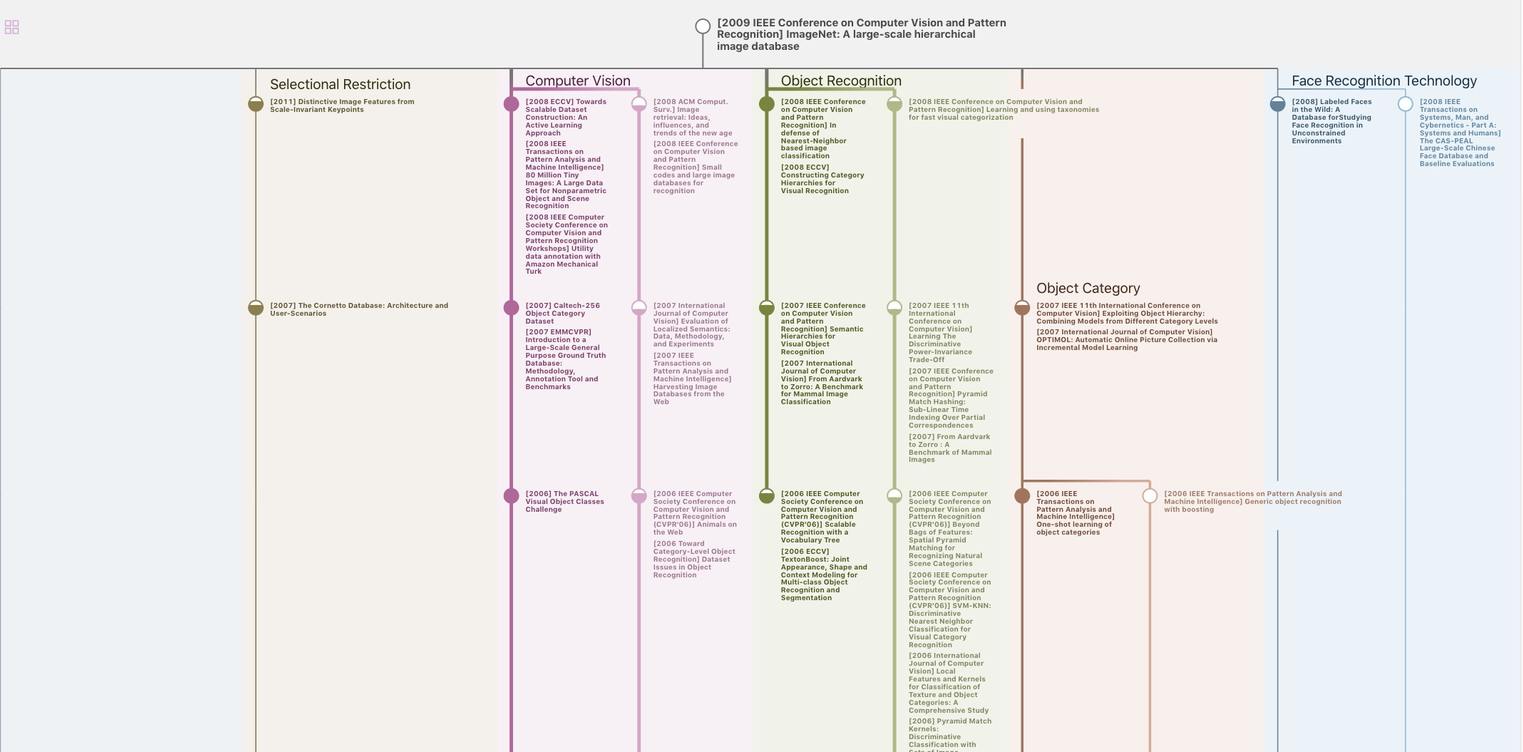
生成溯源树,研究论文发展脉络
Chat Paper
正在生成论文摘要