Optimal sparse polynomial chaos expansion for arbitrary probability distribution and its application on global sensitivity analysis
Computer Methods in Applied Mechanics and Engineering(2022)
摘要
Polynomial chaos expansion has received considerable attention in uncertainty quantification since its great modeling capability for complex systems. However, considering the different variable distribution types and the ‘curse of dimensionality’ of the expansion coefficients, the polynomial chaos expansion has some limitations in the practical engineering application. In this paper, an optimal sparse polynomial chaos expansion is proposed to effectively realize uncertainty quantification with arbitrary probability distribution. This polynomial chaos expansion adopts the derivative λ-PDFs and the corresponding Gegenbauer polynomials to implement stochastic characterization for system model. Because of the excellent approximation ability of derivative λ-PDFs for arbitrary mono-peak distribution, the proposed polynomial chaos expansion has a wide adaptability to arbitrary distribution. Further, through the structure-selection technique based on error reduction ratio to screen the significant basis polynomials, the optimal sparse polynomial chaos expansion can be built with much fewer model evaluations than unknown coefficients. In view of that, the optimal sparse polynomial chaos expansion is applied to global sensitivity analysis, and the analytical solution of Sobol’ sensitivity indices is derived from the coefficients. Because of the advantages of simple structure, high precision and anti-noise ability, this optimal sparse polynomial chaos can improve the efficiency and accuracy of sensitivity analysis and has better adaptability for complex practical engineering problems. One numerical example and two engineering applications are studied to illustrate the practicability and effectiveness of the proposed method.
更多查看译文
关键词
Polynomial chaos expansion,λ-PDF,Structure-selection,Gegenbauer polynomials,Global sensitivity analysis
AI 理解论文
溯源树
样例
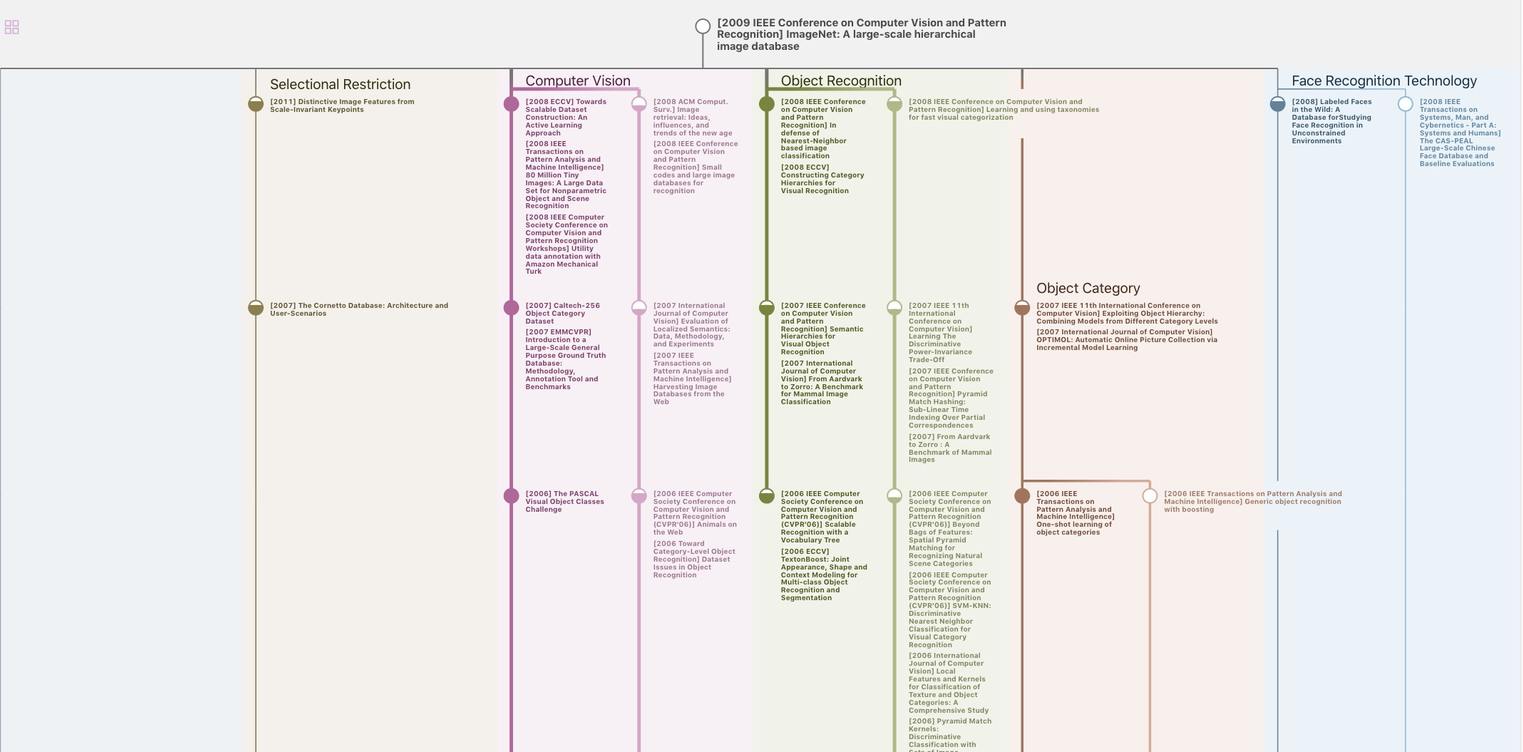
生成溯源树,研究论文发展脉络
Chat Paper
正在生成论文摘要