Prediction of coal spontaneous combustion temperature based on improved grey wolf optimizer algorithm and support vector regression
FUEL(2022)
Abstract
The effective prediction of coal spontaneous combustion temperature is of great importance to the monitoring and prevention of coal mine fires. Aiming at the problem of insufficient prediction accuracy of traditional coal spontaneous combustion temperature prediction model, and considering the characteristics of prediction data samples and the timeliness of applicable models, an improved grey wolf optimized support vector regression coal spontaneous combustion temperature prediction model based on nonlinear parameter control, dynamic inertia weights and grey wolf social hierarchy is proposed, and the effectiveness of the improved grey wolf optimizer algorithm is verified by numerical experiments. The O-2 concentration, CO concentration, C2H4 concentration, CO/AO(2), and C2H4/C2H6 selected from the coal spontaneous combustion procedure warming experiment were used as the input indexes of the prediction model, and the coal body temperature was used as the output index, and the prediction model was compared and analyzed with the particle swarm optimization support vector regression and grey wolf optimized support vector regression models through the experimental data. The results show that the improved grey wolf algorithm has stronger global search ability, faster convergence speed and better stability, and the proposed prediction model has strong advantages in accuracy and stability, which can provide better decision reference for coal spontaneous combustion fire prediction and warning in coal mines.
MoreTranslated text
Key words
Prediction, Support vector regression, Coal spontaneous combustion, Grey wolf optimizer, Dynamic inertia weights
AI Read Science
Must-Reading Tree
Example
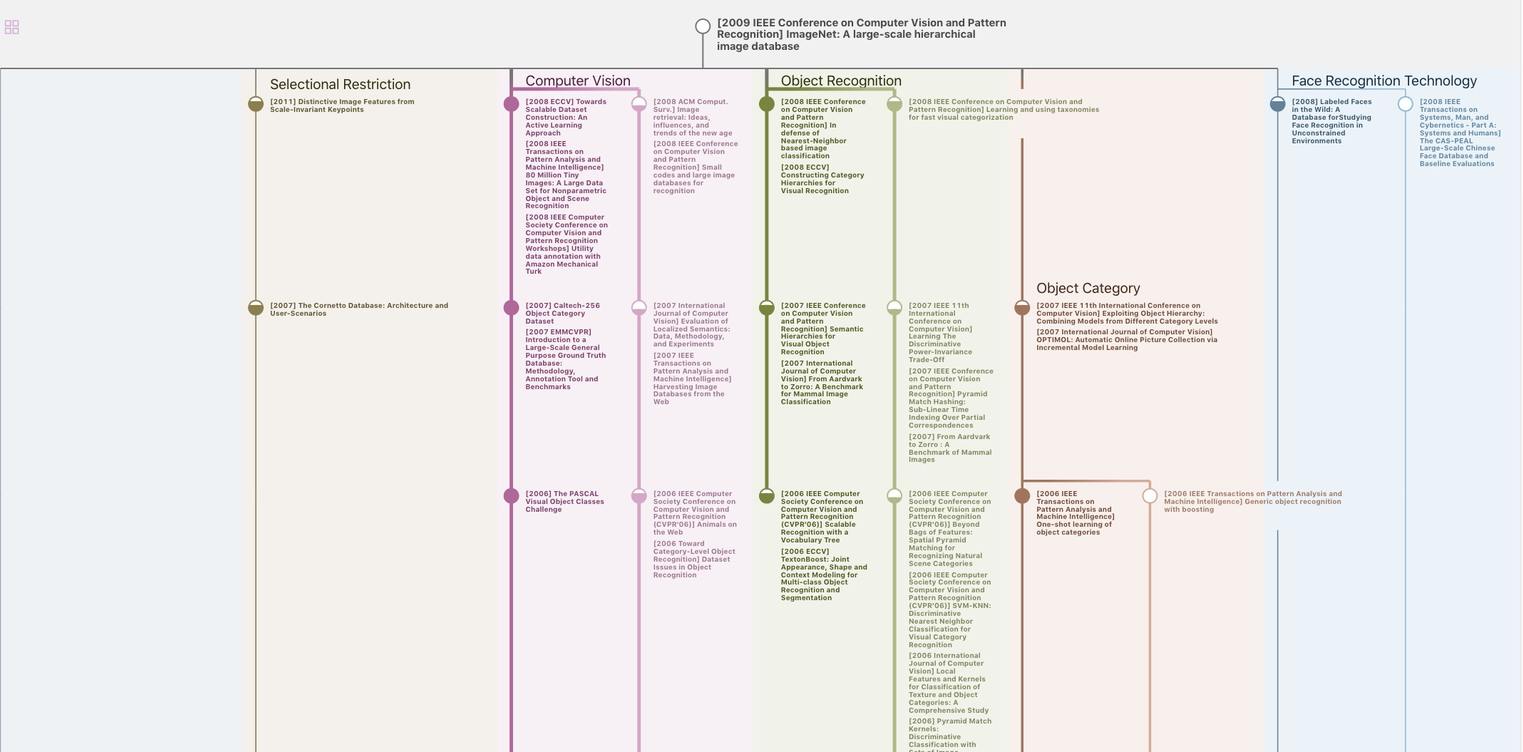
Generate MRT to find the research sequence of this paper
Chat Paper
Summary is being generated by the instructions you defined