A comparison of models for inferring longitudinal reciprocal relationships between constructs: A case example with internalizing and externalizing problems
SOCIAL DEVELOPMENT(2023)
Abstract
Developmental researchers commonly utilize longitudinal data to decompose reciprocal and dynamic associations between repeatedly measured constructs to better understand the temporal precedence between constructs. Although the cross-lagged panel model (CLPM) is commonly used in developmental research, it has been criticized for its potential to produce biased estimates due to the fact of ignoring the trait-like, time-invariant nature of stability in constructs across people and aggregating the between- and within-person effects together as a single estimate. Recently, a growing set of alternatives have emerged, but the estimates across CLPM and alternatives have rarely been compared in developmental research. The primary purpose of this article is to (a) provide a three-component framework to help developmental researchers to select and specify the most appropriate model, and (b) illustrate how models differ in estimates with an empirical example investigating the reciprocal associations between internalizing and externalizing problems in school-aged children. Methods: We specified CLPM, Random-Intercept Cross-lagged Panel Model (RI-CLPM), Latent Curve Model with Structured Residuals (LCM-SR), Latent Change Score (LCS), and a Random-Mean LCS using four waves of data from ECLS-K: 2011 (N = 8779). Results: The CLPM provided the most evidence of significant cross-lagged paths but the poorest fit to the data compared to other models. Alternative models had excellent fit and found either only negative temporal precedence from internalizing to externalizing problems or simply no evidence of prospective within-person relations between internalizing and externalizing problems.
MoreTranslated text
Key words
cross-lagged panel models,internalizing and externalizing problems,reciprocal model comparison
AI Read Science
Must-Reading Tree
Example
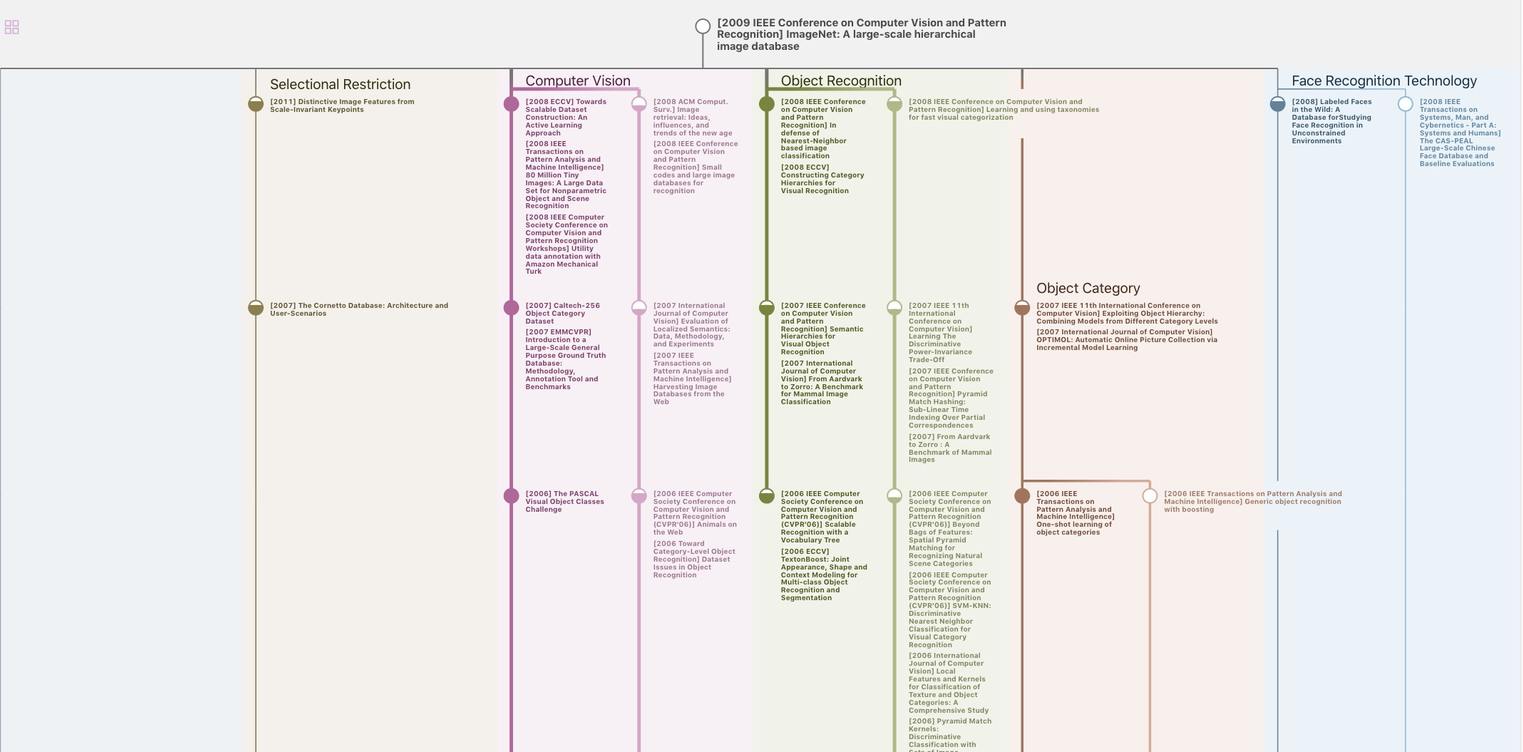
Generate MRT to find the research sequence of this paper
Chat Paper
Summary is being generated by the instructions you defined