Water quality classification using machine learning algorithms
Journal of Water Process Engineering(2022)
摘要
Monitoring water quality is essential for protecting human health and the environment and controlling water quality. Artificial Intelligence (AI) offers significant opportunities to help improve the classification and prediction of water quality (WQ). In this study, various AI algorithms are assessed to handle WQ data collected over an extended period and develop a dependable approach for forecasting water quality as accurately as possible. Specifically, various machine learning classifiers and their stacking ensemble models were used to classify the WQ data via the Water Quality Index (WQI). The studied classifiers included Support Vector Machine (SVM), Random Forest (RF), Logistic Regression (LR), Decision Tree (DT), CATBoost, XGBoost, and Multilayer Perceptron (MLP). The dataset used in the study included 1679 samples and their meta-data collected over nine years. In addition, precision-recall curves and Receiver Operating Characteristic curves (ROC) were used to assess the performance of the various classifiers. The findings revealed that the CATBoost model offered the most accurate classifier with a percentage of 94.51. Moreover, after applying stacking ensemble models with all classifiers, accuracy reached 100% in various Meta-classifiers. Furthermore, the CATBoost achieved the highest accuracy as a primary gradient boosting algorithm and a meta classifier. Therefore, the boosting algorithm is proposed as a reliable approach for the WQ classification. The analysis presented in this article presents a framework that can support the efforts of researchers working toward water quality improvement using artificial intelligence.
更多查看译文
关键词
Water quality,Water quality index,Machine learning,Stack modelling,Meta classifier,Ensemble models
AI 理解论文
溯源树
样例
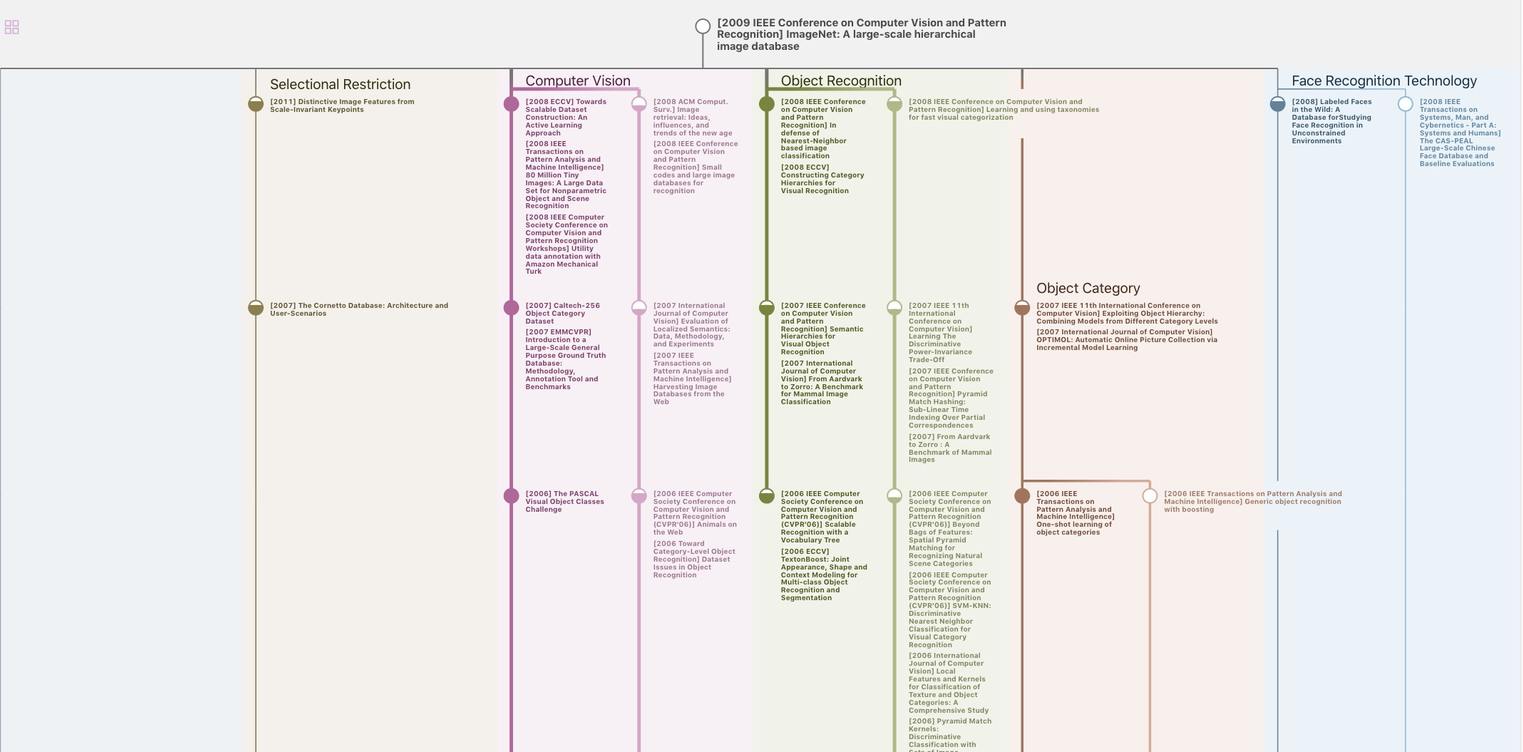
生成溯源树,研究论文发展脉络
Chat Paper
正在生成论文摘要