SCA-Net: A Multiscale Building Segmentation Network Incorporating a Dual-Attention Mechanism
IEEE ACCESS(2022)
Abstract
The traditional deep learning networks in remote sensing image building segmentation have problems such as incomplete internal extraction, low accuracy of edge segmentation, and difficult prediction of irregular targets. Based on this, this paper proposes the network SCA-Net, which fuses the dense connection feature pyramid (DCFP) module and the spatial and channel attention mechanisms (SCA) module, for high precision automatic extraction of building contours. First, the DCFP module is used to obtain a large range of multi-scale contextual information without sacrificing the spatial resolution of features, thus improving the recognition rate of small-sized buildings. Second, SCA module is used to enhance feature learning ability of building pixels and suppress irrelevant feature information input. Finally, a fully convolutional asymmetric encoder-decoder structure is designed to obtain the final building segmentation results by gradually fusing the features and decoding them. The results on the WHU dataset as well as the CHN dataset show that the extraction of buildings using this method has good extraction results and high accuracy performance, outperforming several comparable advanced networks.
MoreTranslated text
Key words
Feature extraction, Buildings, Data mining, Convolution, Semantics, Remote sensing, Radio frequency, Building extraction, SCA-Net, deep learning, attention mechanism, high-resolution aerial images
AI Read Science
Must-Reading Tree
Example
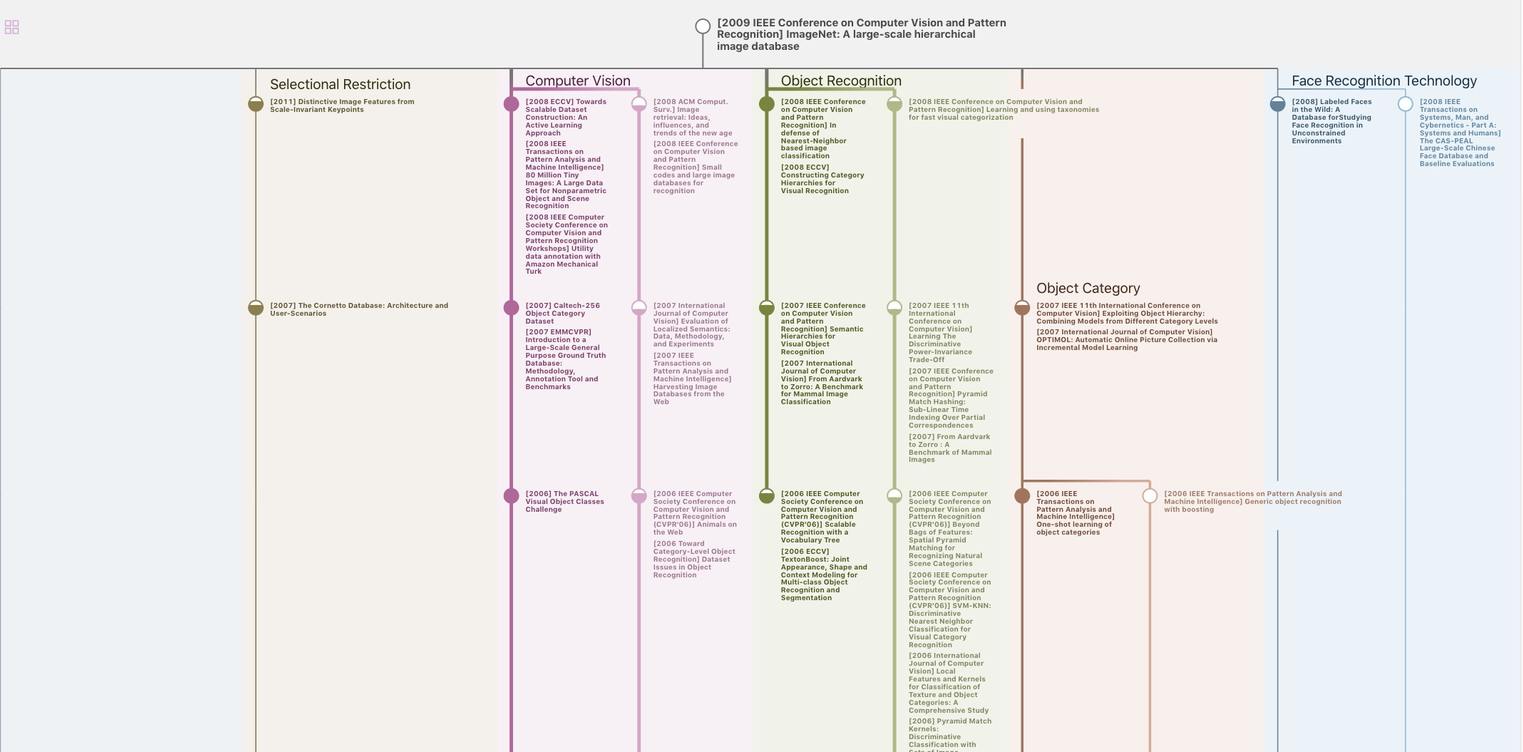
Generate MRT to find the research sequence of this paper
Chat Paper
Summary is being generated by the instructions you defined