Transfer learning using small-sized dataset for concentrate ash content prediction of coal flotation
INTERNATIONAL JOURNAL OF COAL PREPARATION AND UTILIZATION(2023)
摘要
Real-time and accurate acquisition of ash content is crucial for coal flotation monitoring but existing modeling approaches have been hindered from a large dataset labeled by the target task. The method proposed in this paper relies on a transfer learning approach that is able to exploit the knowledge of a source domain (ImageNet and S1) to improve the result of a task performed on the target domain (S2). The ResNet 101 is selected as the main Convolutional neural network (CNN) framework of transfer learning among six state-of-the-art CNN modules in this study. Freeze and fine-tune are used to transfer the weights of the CNN layers to the industry ash content classification task. The tuning results of neurons and fully connected layers show that the classification results based on the weights knowledge of S1 obtained through the proposed transfer method outperform the training direct method by achieving an accuracy of 86.26% and F1-score of 85.19% over the small-sized industry dataset. The 3-D discriminant feature score and ROC curves demonstrate satisfactory prediction performance in coal flotation industrial ash content prediction. Generally, this work provides new options for flotation monitoring in tasks of training ash content labels shortage.
更多查看译文
关键词
Coal flotation,ash content,convolutional neural network,transfer learning,machine learning
AI 理解论文
溯源树
样例
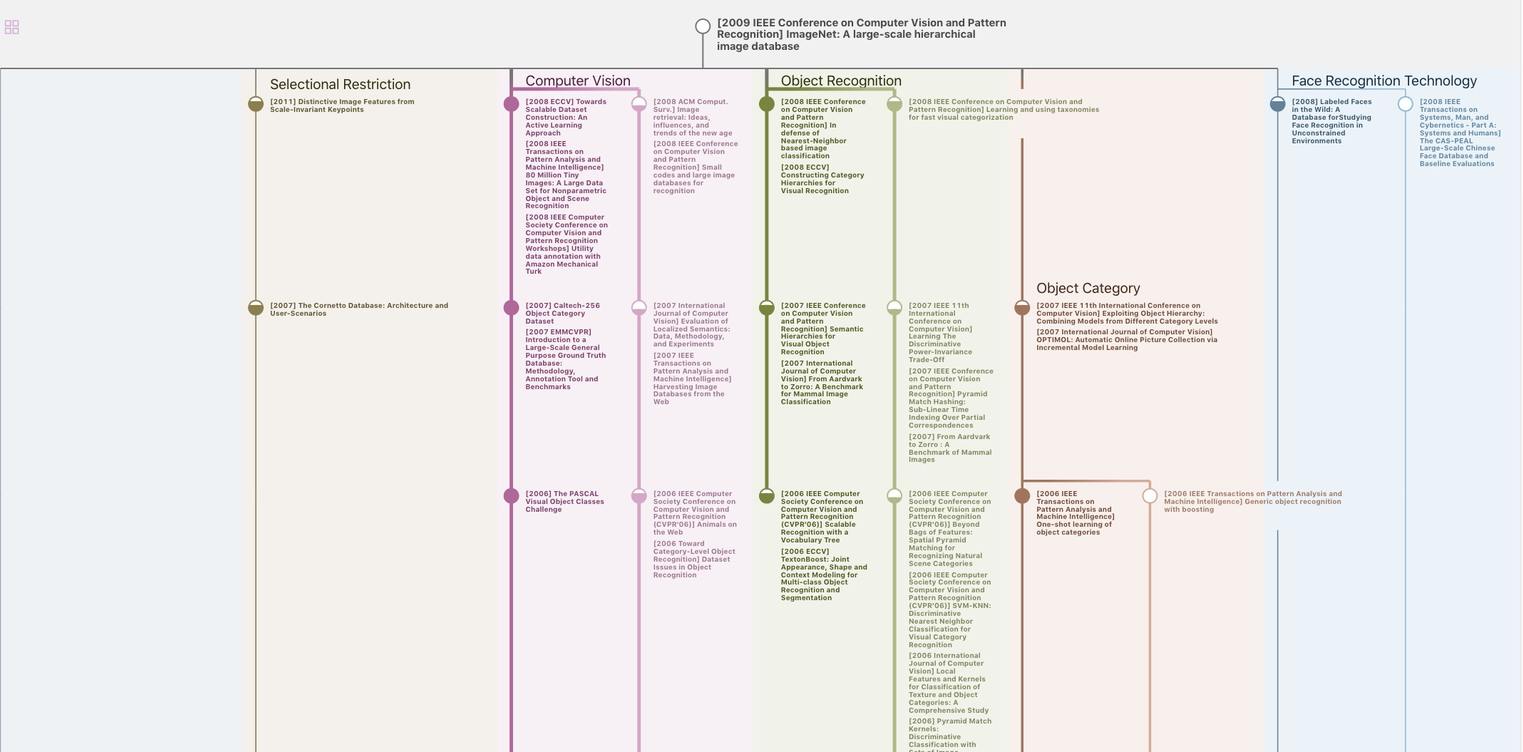
生成溯源树,研究论文发展脉络
Chat Paper
正在生成论文摘要