A Hybrid Forecast Model for Household Electric Power by Fusing Landmark-Based Spectral Clustering and Deep Learning
SUSTAINABILITY(2022)
Abstract
Household power load forecasting plays an important role in the operation and planning of power grids. To address the prediction issue of household power consumption in power grids, this paper chooses a time series of historical power consumption as the feature variables and uses landmark-based spectral clustering (LSC) and a deep learning model to cluster and predict the power consumption dataset, respectively. Firstly, the investigated data are reshaped into a matrix and all missing entries are recovered by matrix completion. Secondly, the data samples are divided into three clusters by the LSC method according to the periodicity and regularity of power consumption. Then, all samples in each cluster are expanded via bootstrap aggregating technique. Subsequently, a combination of a convolutional neural network (CNN) and a long short-term memory (LSTM) is employed to predict power consumption. The goal of CNN is to extract the features from input data in sequence learning, and LSTM aims to train and predict the power consumption. Finally, the forecasting performance of the LSC-CNN-LSTM is compared with several other deep learning models to verify its reliability and effectiveness in the field of household power load. The experimental results show that the proposed hybrid method is superior to other state-of-the-art deep learning techniques in forecasting performance.
MoreTranslated text
Key words
household power load forecasting,deep learning,matrix completion,landmark-based spectral clustering,convolutional neural network,long short-term memory
AI Read Science
Must-Reading Tree
Example
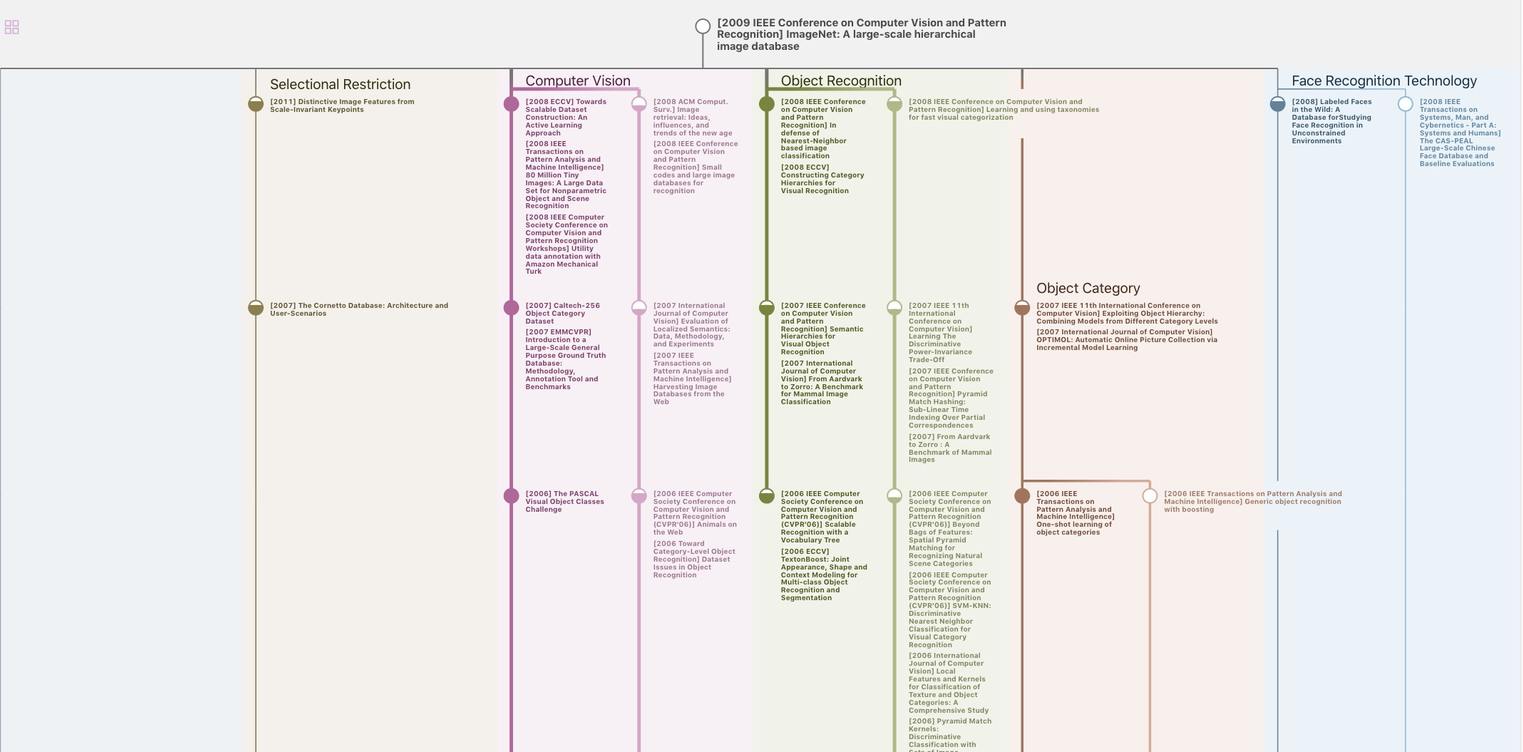
Generate MRT to find the research sequence of this paper
Chat Paper
Summary is being generated by the instructions you defined