A Computer-Aided Diagnostic System for Diabetic Retinopathy Based on Local and Global Extracted Features
APPLIED SCIENCES-BASEL(2022)
摘要
Featured Application This paper presents a novel deep learning system for the detection and diagnosis of diabetic retinopathy using optical coherence tomography images. Diabetic retinopathy (DR) is a major public health problem and the leading cause of vision loss in the working age population. This paper presents a novel deep learning system for the detection and diagnosis of DR using optical coherence tomography (OCT) images. The input for this system is three-channel local and global information from OCT images. The local high-level information is represented by the thickness channel and the reflectivity channel. The global low-level information is represented by the grey-level OCT original image. The deep learning system processes the three-channel input to produce the final DR diagnoses. Experimental results on 200 OCT images, augmented to 800 images, which are collected by the University of Louisville, show high system performance related to other competing methods. Moreover, 10-fold and leave-one-subject-out (LOSO) experiments are performed to confirm how significant using the fused images is in improving the performance of the diagnoses, by investigating four different CNN architectures. All of the four architectures achieve acceptable performance and confirm a significant performance improvement using the fused images. Using LOSO, the best network performance has improved from 90.1 +/- 2% using only the grey level dataset to 97.7 +/- 0.5% using the proposed fused dataset. These results confirm the promise of using the proposed system for the detection of DR using OCT images.
更多查看译文
关键词
diabetic retinopathy, deep learning, low level information, high level information
AI 理解论文
溯源树
样例
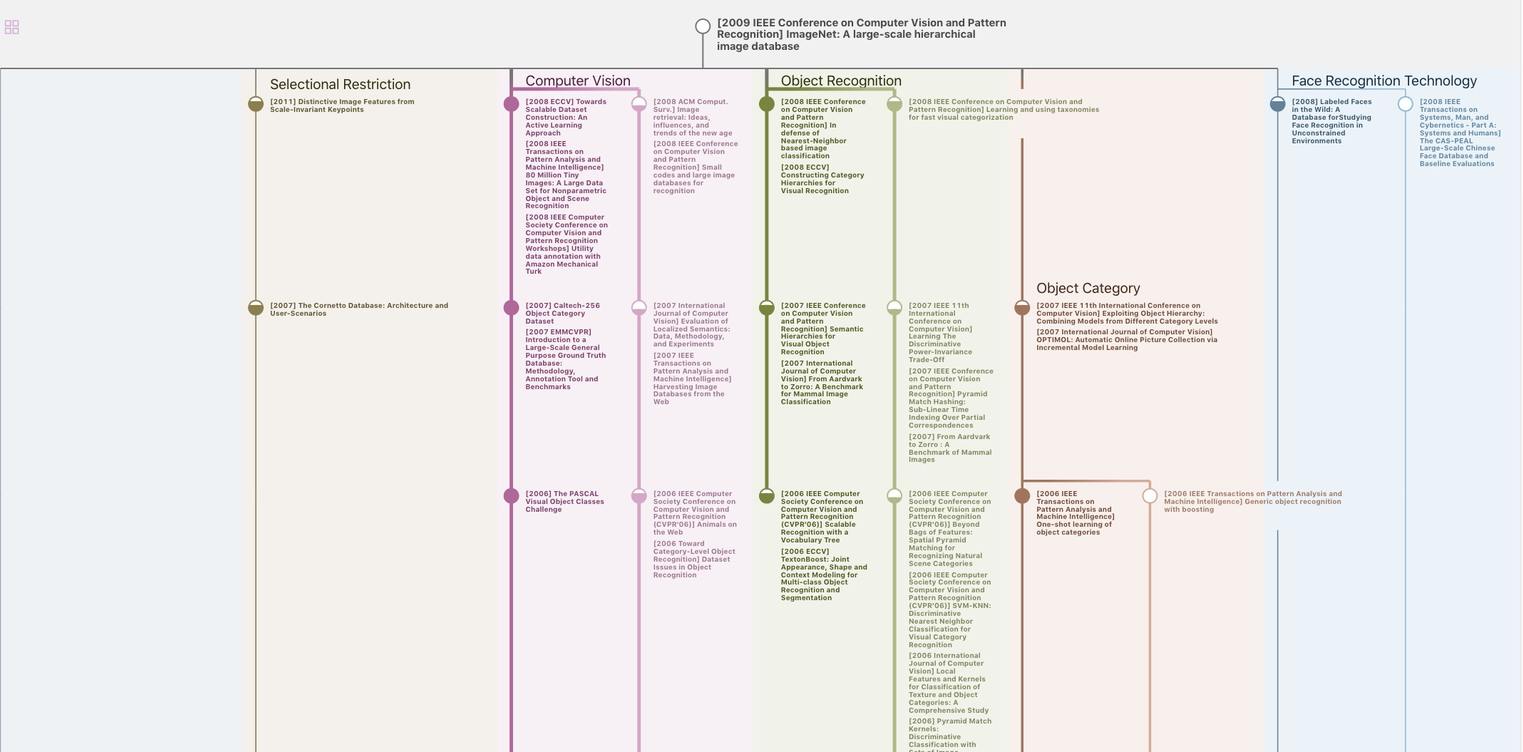
生成溯源树,研究论文发展脉络
Chat Paper
正在生成论文摘要