Active Deep Feature Extraction for Hyperspectral Image Classification Based on Adversarial Learning
IEEE GEOSCIENCE AND REMOTE SENSING LETTERS(2022)
Abstract
The issues of spectral redundancy and limited training samples hinder the widespread application and development of hyperspectral images. In this letter, a novel active deep feature extraction scheme is proposed by incorporating both representative and informative measurement. First, an adversarial autoencoder (AAE) is modified to suit the classification task with deep feature extraction. Dictionary learning and a multivariance and distributional distance (MVDD) measure are then introduced to choose the most valuable candidate training samples, where we use the limited labeled samples to obtain a high classification accuracy. Comparative experiments with the proposed querying strategy were carried out with two hyperspectral datasets. The experimental results obtained with the two datasets demonstrate that the proposed scheme is superior to the others. With this method, the unstable increase in accuracy is eliminated by incorporating both informative and representative measurement.
MoreTranslated text
Key words
Training, Hyperspectral imaging, Feature extraction, Dictionaries, Uncertainty, Image classification, Deep learning, Active learning, adversarial autoencoder (AAE), hyperspectral image classification, sparse representation
AI Read Science
Must-Reading Tree
Example
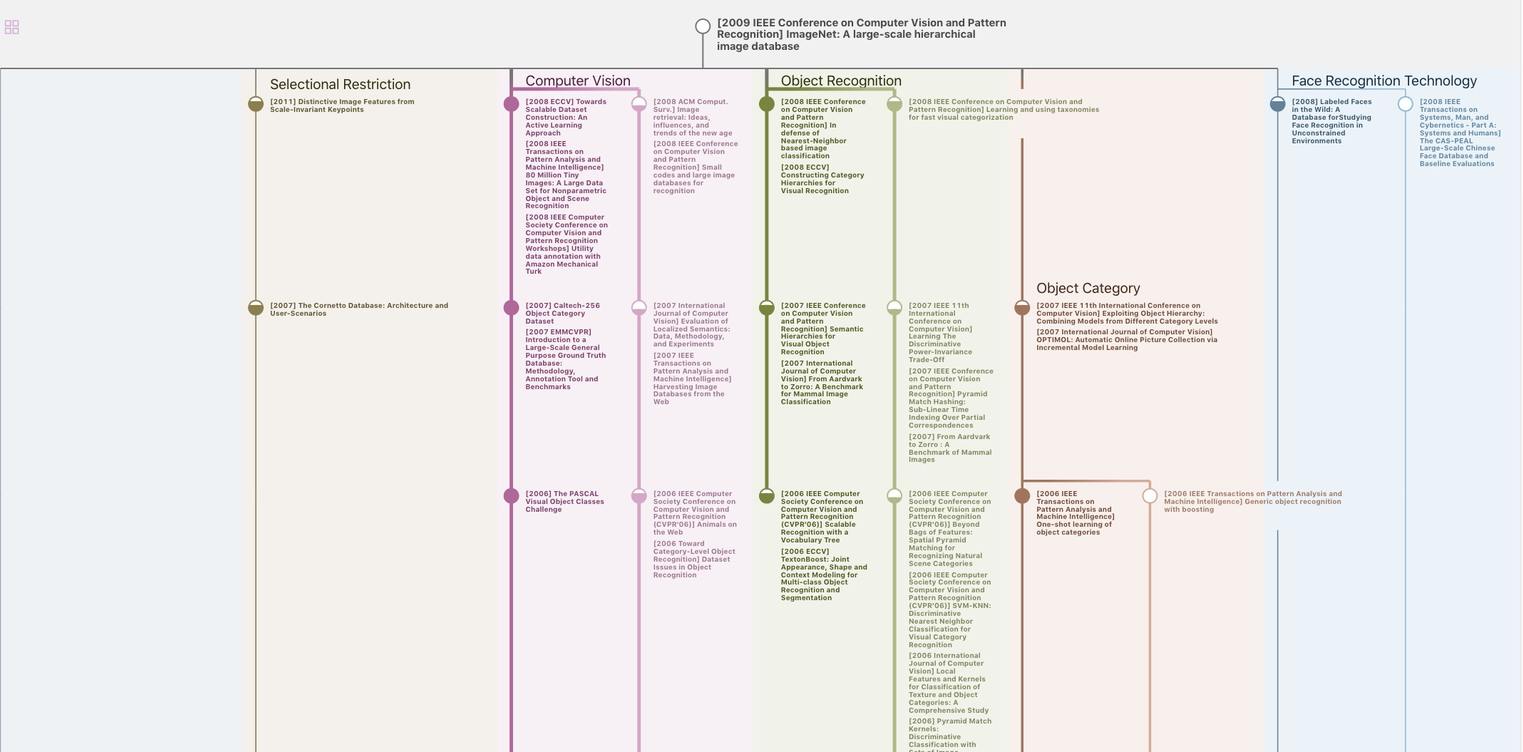
Generate MRT to find the research sequence of this paper
Chat Paper
Summary is being generated by the instructions you defined