A machine learning-based approach for generating high-resolution soil moisture from SMAP products
GEOCARTO INTERNATIONAL(2022)
摘要
The coarse resolutions of passive microwave surface soil moisture (SSM) products often hamper the applications of such products in regional or local studies. This study developed a machine learning-based approach for the downscaling of Soil Moisture Active and Passive (SMAP) SSM products at both ascending and descending passes based on the relationships between SSM and environmental variables, such as the land surface temperature (LST), enhanced vegetation index (EVI), surface albedo, cumulative precipitation, digital elevation model (DEM), and soil texture. Two machine learning algorithms-random forest (RF) and long short-term memory network (LSTM)-were applied to downscale the SMAP product from a coarse resolution (36 km) to a fine resolution (1 km). The downscaled SSM results were validated against ground observations. The contributions of environmental variables to the established model were also discussed. The results showed that both RF and LSTM models demonstrated satisfactory performance in SMAP SSM prediction, indicating that the downscaling models were able to learn the relationships between the environmental variables and SMAP SSM well at coarse resolution. The RF and LSTM-based downscaled SSM maps not only realized full spatial coverage but also reasonably maintained the spatiotemporal evolution trends of the original SMAP SSM products. Both downscaled results correlated well with the ground observations and indicated good temporal consistency with the daily precipitation. In addition, the variable importance analysis reveals that the DEM, precipitation, EVI, and surface albedo were dominant to establishing SSM regression models, particularly for DEM in regions with large height differences. Overall, RF and LSTM-based models are promising downscaling approaches for generating full spatial coverage and fine-resolution SSM data from passive microwave SSM.
更多查看译文
关键词
Soil moisture, random forest, long short-term memory, spatial downscaling, SMAP
AI 理解论文
溯源树
样例
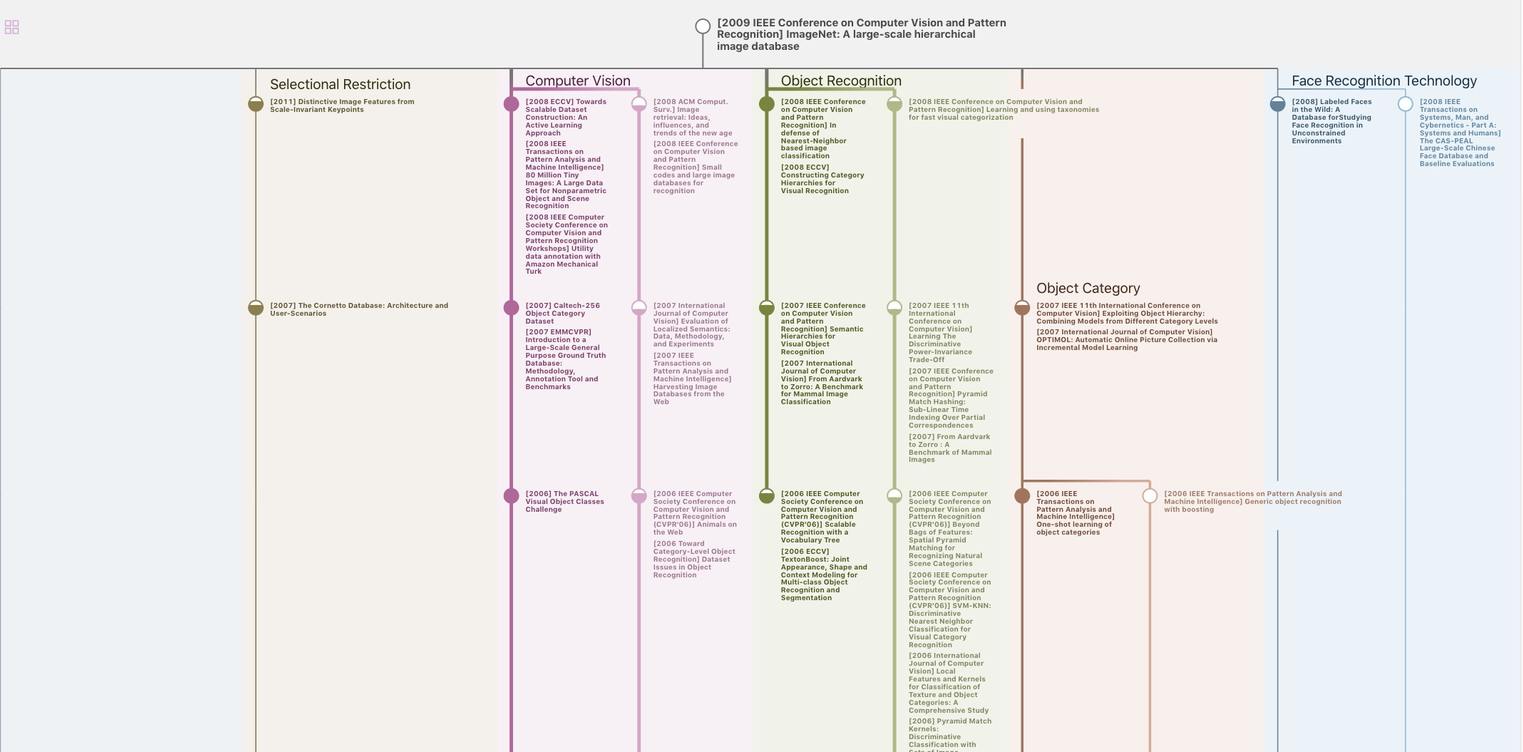
生成溯源树,研究论文发展脉络
Chat Paper
正在生成论文摘要