Regularized Spectral Spike Response Model: A Neuron Model for Robust Parameter Reduction
BRAIN SCIENCES(2022)
摘要
The modeling procedure of current biological neuron models is hindered by either hyperparameter optimization or overparameterization, which limits their application to a variety of biologically realistic tasks. This article proposes a novel neuron model called the Regularized Spectral Spike Response Model (RSSRM) to address these issues. The selection of hyperparameters is avoided by the model structure and fitting strategy, while the number of parameters is constrained by regularization techniques. Twenty firing simulation experiments indicate the superiority of RSSRM. In particular, after pruning more than 99% of its parameters, RSSRM with 100 parameters achieves an RMSE of 5.632 in membrane potential prediction, a VRD of 47.219, and an Fl-score of 0.95 in spike train forecasting with correct timing (+/- 1.4 ms), which are 25%, 99%, 55%, and 24% better than the average of other neuron models with the same number of parameters in RMSE, VRD, F1-score, and correct timing, respectively. Moreover, RSSRM with 100 parameters achieves a memory use of 10 KB and a runtime of 1 ms during inference, which is more efficient than the Izhikevich model.
更多查看译文
关键词
spike response model, neuron models, Izhikevich neuron model
AI 理解论文
溯源树
样例
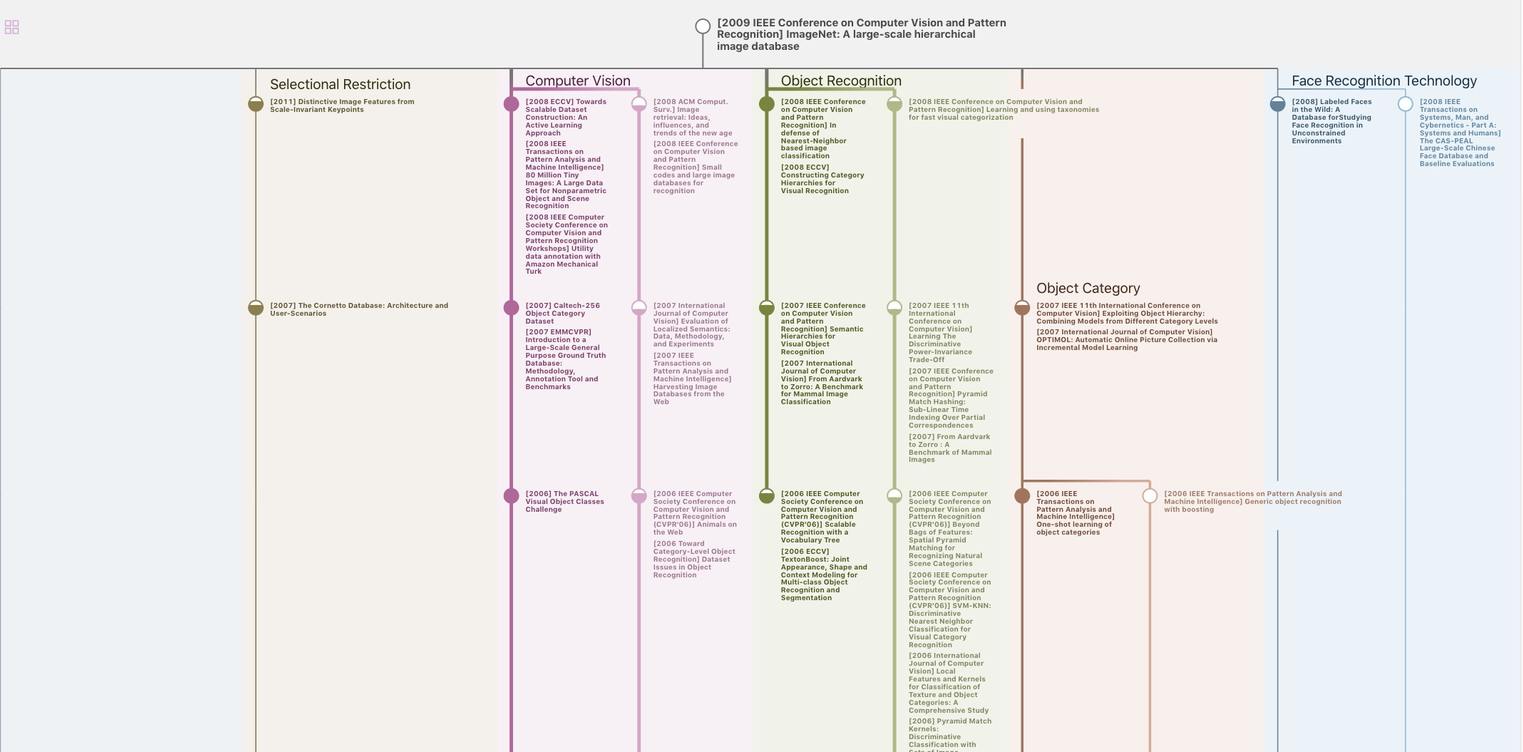
生成溯源树,研究论文发展脉络
Chat Paper
正在生成论文摘要