Classification and Total Carbon Determination of the Soils Using RGB Digital Images Combined with Machine Learning
COMMUNICATIONS IN SOIL SCIENCE AND PLANT ANALYSIS(2023)
摘要
An alternative approach for soil characterization is proposed, using digital images and machine learning. The present work investigated the Red, Green, and Blue (RGB) pattern with Artificial Neural Network (ANN) and Random Forest (RF) in the classification of Oxisol and Inceptisol collected in South Brazil. Furthermore, we also built ANN, RF, Partial Least Squares (PLS), and Support Vector Machine (SVM) models from digital images to quantify Total Carbon (TC) content in soil samples. Preliminary evaluations considered the hypothesis that alternative methods of classification and quantification of soil properties might provide results comparable to traditional methods. The TC content of 91 soil samples, 40 from Oxisol and 51 from Inceptisol were determined by dry combustion. ANN and RF as machine learning algorithms were applied to classify the two kinds of soils. The classification quality was assessed by the percentage of correctly classified instances. The results suggest using digital images and machine learning algorithms as a new methodology to soil classification. The best machine learning method was RF which can be used to classify soil types by the RGB channel colors of the soil images. The use of digital images and RF is a clean (eco-friendly), rapid, and affordable strategy analysis for TC quantification in soils. Therefore, the proposed methods represent an advancement in soil classification, such as the determination of the TC content of soils.
更多查看译文
关键词
Artificial neural network, organic matter, partial least squares, Random forest, support vector machine
AI 理解论文
溯源树
样例
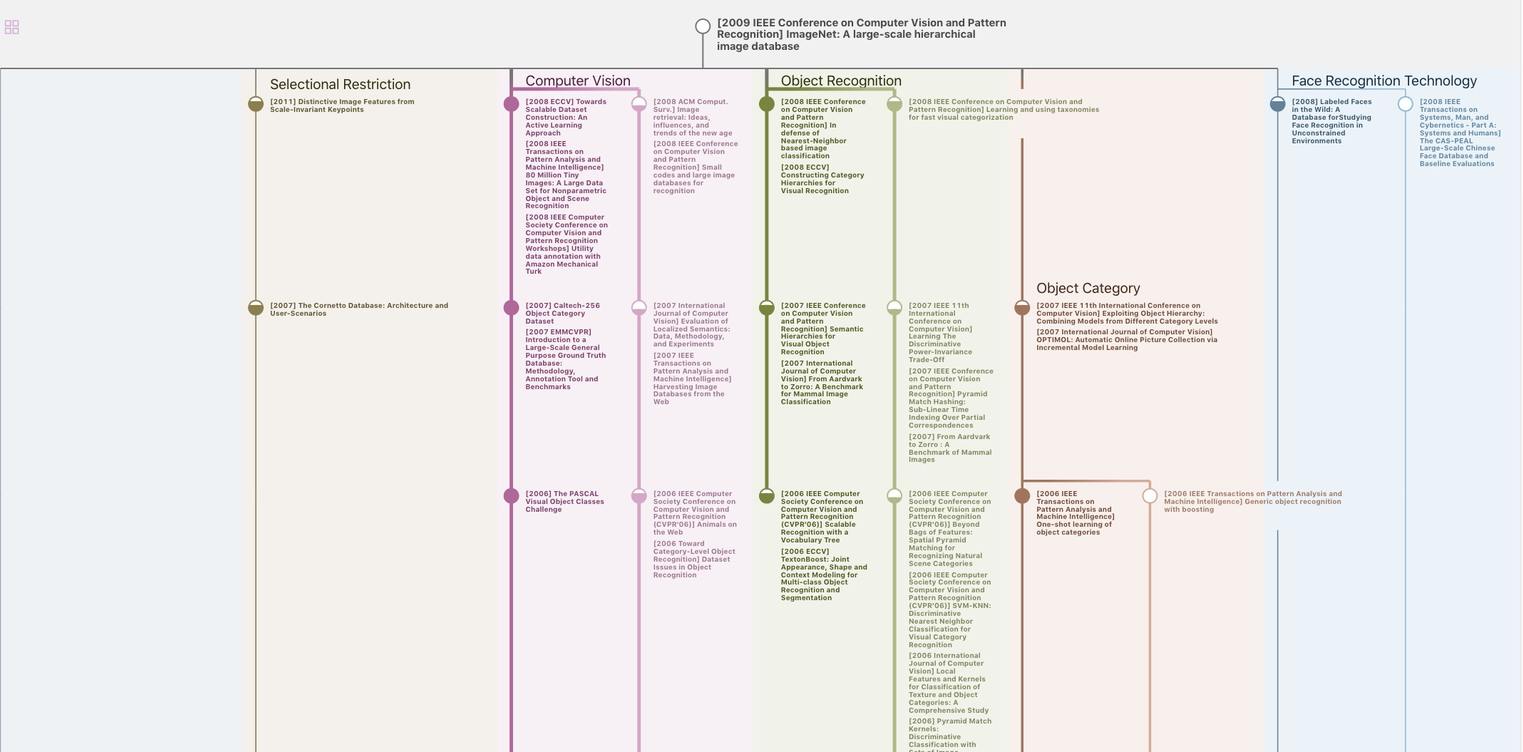
生成溯源树,研究论文发展脉络
Chat Paper
正在生成论文摘要