Bayesian Aerosol Retrieval-Based PM2.5 Estimation through Hierarchical Gaussian Process Models
MATHEMATICS(2022)
摘要
Satellite-based aerosol optical depth (AOD) data are widely used to estimate land surface PM2.5 concentrations in areas not covered by ground PM2.5 monitoring stations. However, AOD data obtained from satellites are typically at coarse spatial resolutions, limiting their applications on small or medium scales. In this paper, we propose a new two-step approach to estimate 1-km-resolution PM2.5 concentrations in Shanghai using high spatial resolution AOD retrievals from MODIS. In the first step, AOD data are refined to a 1 x 1 km(2) resolution via a Bayesian AOD retrieval method. In the second step, a hierarchical Gaussian process model is used to estimate PM2.5 concentrations. We evaluate our approach by model fitting and out-of-sample cross-validation. Our results show that the proposed approach enjoys accurate predictive performance in estimating PM2.5 concentrations.
更多查看译文
关键词
Bayesian retrieval algorithm, PM2.5, hierarchical Gaussian process model, MAIAC
AI 理解论文
溯源树
样例
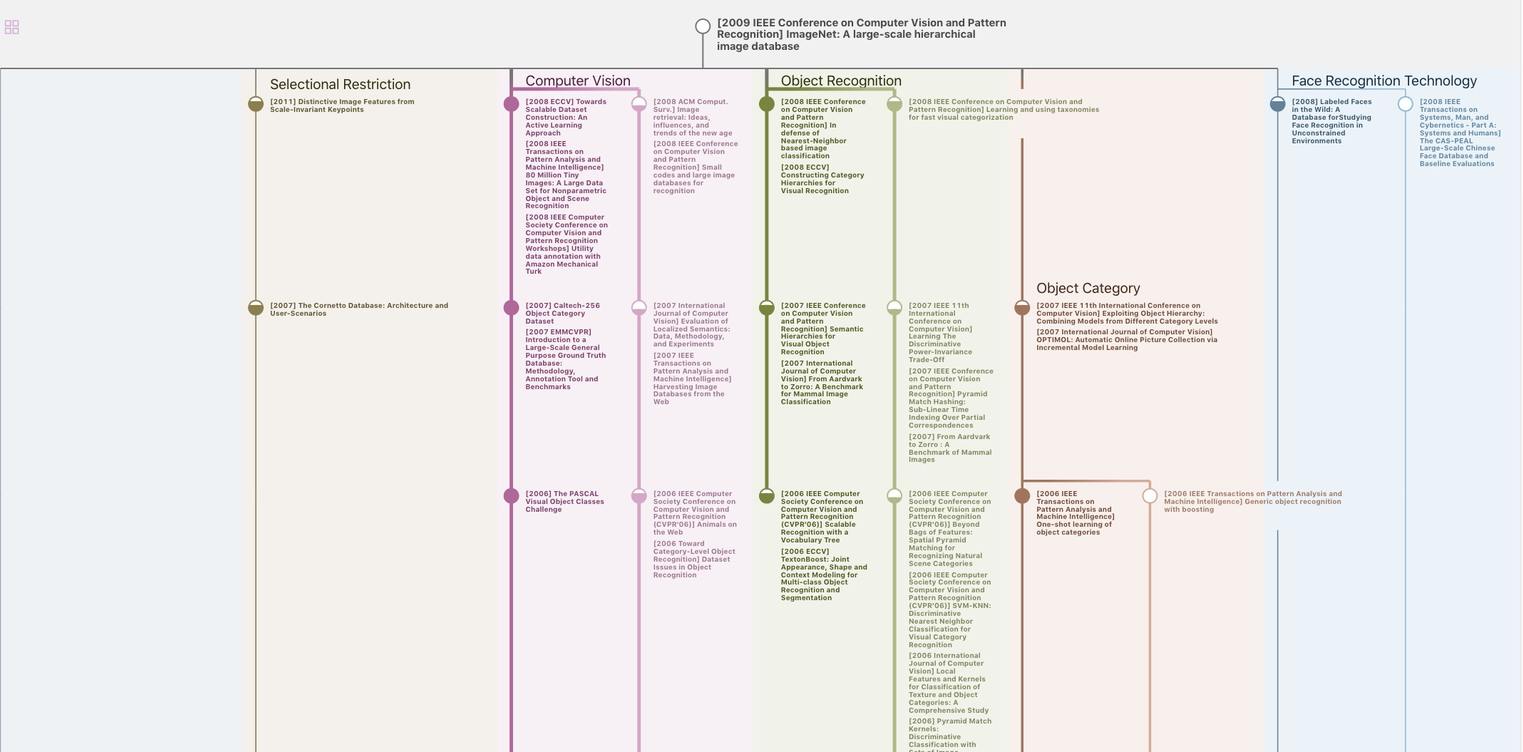
生成溯源树,研究论文发展脉络
Chat Paper
正在生成论文摘要