Federated Learning for Indoor Localization via Model Reliability With Dropout
IEEE Communications Letters(2022)
摘要
In this letter, we propose a novel model weight update method that accounts for the reliability of the local clients in FL-based indoor localization. FL shows degraded localization performance than centralized learning because of the non-independent and identically distributed (non-IID) data configuration. Thus, we aim to improve the localization performance by applying the reliability of the local clients, which is quantified by the model uncertainty of the local models. Bayesian models provide a framework for capturing model uncertainty but usually requires a substantial computational cost as well, particularly for high-dimensional learning problems. In order to resolve this computational issue, the proposed scheme applies Monte Carlo (MC) dropout to approximate the Bayesian uncertainty quantification with enhanced computational efficiency. Our simulation results show that the proposed learning method improves localization performance compared to the existing model, federated averaging (FedAvg), and close to the centralized learning performance.
更多查看译文
关键词
Federated learning (FL),indoor localization,model uncertainty,Bayesian approximation
AI 理解论文
溯源树
样例
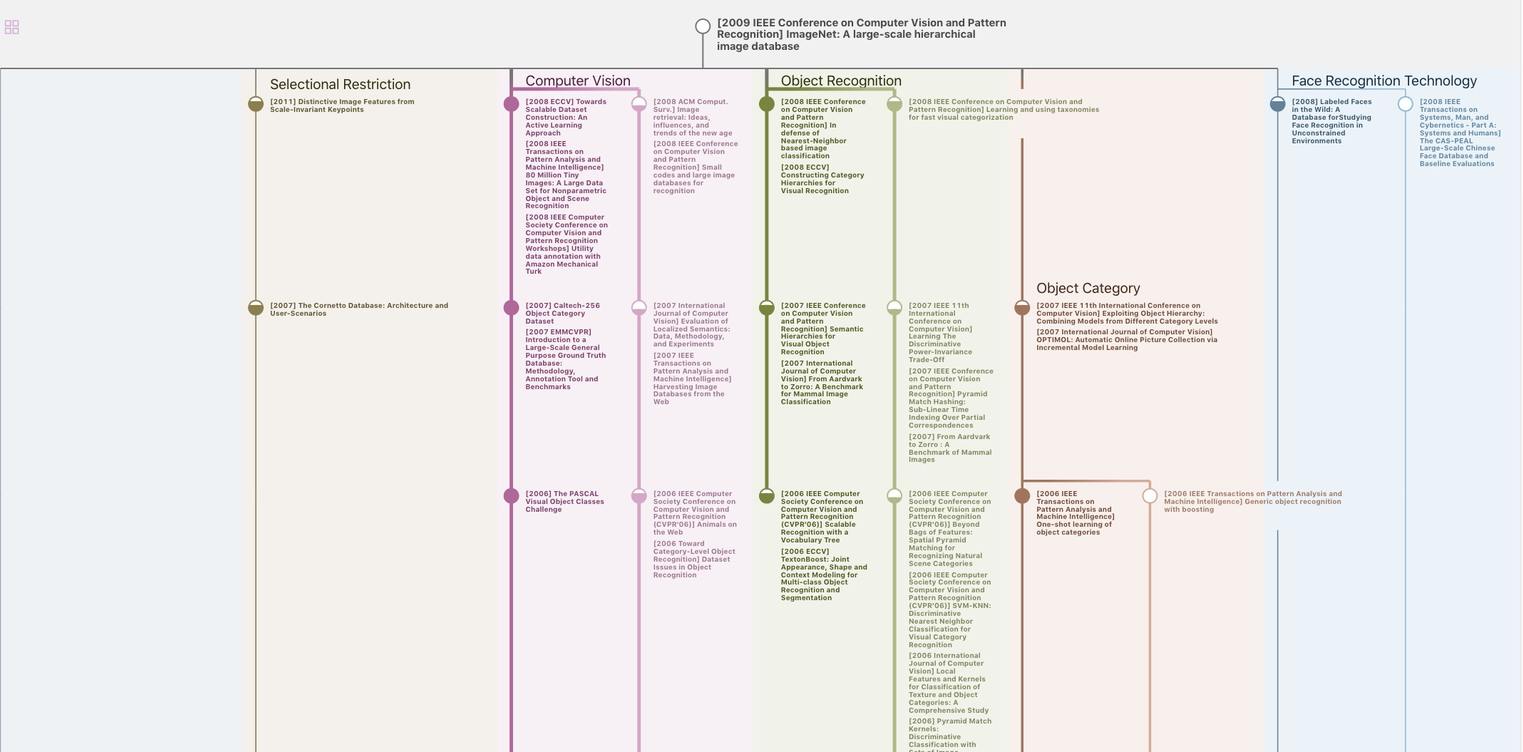
生成溯源树,研究论文发展脉络
Chat Paper
正在生成论文摘要