Feature decomposition and enhancement for unsupervised medical ultrasound image denoising and instance segmentation
Applied Intelligence(2022)
摘要
In this paper, we study how to denoise medical ultrasound images and improve the performance of instance segmentation using deep learning technology. Since medical ultrasound images usually contain a lot of noises, we first propose a novel unsupervised learning approach called Dual Image (DI) for denoising of medical ultrasound images. DI consists of three main features. Firstly, unlike many existing supervised denoising methods, it does not need clean medical ultrasound images for denoising. Instead, it uses Computed Tomography (CT) images and the noise patches extracted from medical ultrasound images for denoising. Secondly, to effectively select noise patches from medical ultrasound images, a patch selection algorithm based on entropy is formulated. Thirdly, to minimize structure variation of denoised medical ultrasound images, a new reconstruction block is designed for combining the structural information from the structural enhancement block. After denoising, since medical ultrasound images are usually poor in features, to further improve the instance segmentation performance, we extend SOLOv2 to Segmenting on Ultrasound Image (SOUI) by proposing the Double Feature Pyramid Network (D-FPN) and mask fusion branch to strengthen the communication and fusion of different feature layers. Extensive experiments have been performed to study the performance of DI and SOUI using practical medical ultrasound images. We demonstrate that DI can greatly improve the quality of medical ultrasound images and minimize structure variation of denoised medical ultrasound images. SOUI gives 53.7% AP(Average Precision) on practical medical ultrasound images, and outperforms most the state-of-the-art instance segmentation methods including SOLOv2. Code is available at: https://github.com/ztt0821/SOUI .
更多查看译文
关键词
Medical ultrasound imaging, Image denoising, Instance segmentation, Convolution neural network (CNN)
AI 理解论文
溯源树
样例
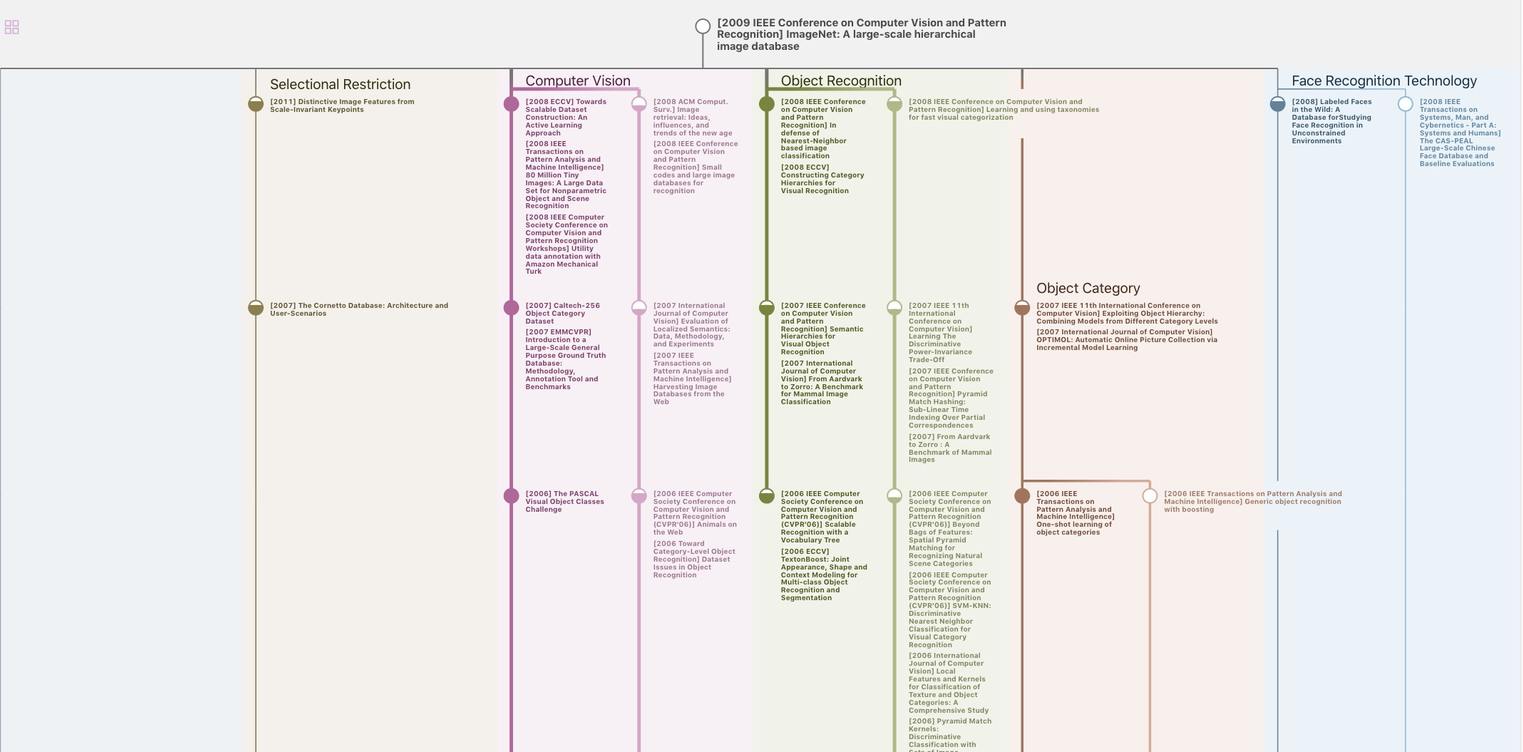
生成溯源树,研究论文发展脉络
Chat Paper
正在生成论文摘要