Short-term load forecasting using time series clustering
OPTIMIZATION AND ENGINEERING(2022)
Abstract
Short-term load forecasting plays a major role in energy planning. Its accuracy has a direct impact on the way power systems are operated and managed. We propose a new Clustering-based Similar Pattern Forecasting algorithm (CSPF) for short-term load forecasting. It resorts to a K-Medoids clustering algorithm to identify load patterns and to the COMB distance to capture differences between time series. Clusters’ labels are then used to identify similar sequences of days. Temperature information is also considered in the day-ahead load forecasting, resorting to the K-Nearest Neighbor approach. CSPF algorithm is intended to provide the aggregate forecast of Portugal's national load, for the next day, with a 15-min discretization, based on data from the Portuguese Transport Network Operator (TSO). CSPF forecasting performance, as evaluated by RMSE, MAE and MAPE metrics, outperforms three alternative/baseline methods, suggesting that the proposed approach is promising in similar applications.
MoreTranslated text
Key words
Clustering time series,Distance measures,Load pattern,Sequence Pattern,Similar Pattern Method,Short-term load forecasting
AI Read Science
Must-Reading Tree
Example
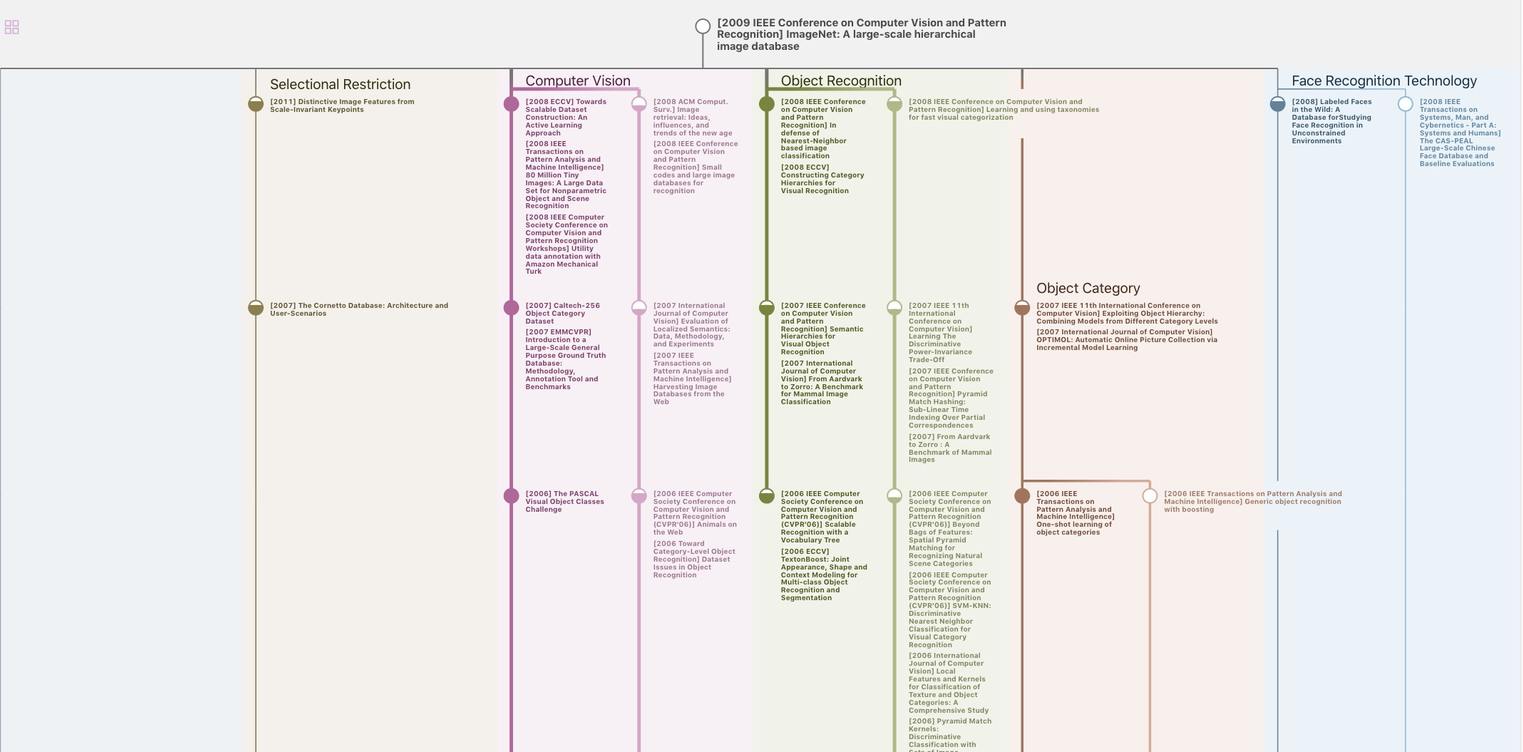
Generate MRT to find the research sequence of this paper
Chat Paper
Summary is being generated by the instructions you defined