FAWNet: two-phase attention based street view image classification for urban land use analysis
REMOTE SENSING LETTERS(2022)
摘要
Street view image (SVI) is becoming one of the most essential proximity sensing data for urban land-use study. Because of the highly abstract nature of their labels (e.g., commercial area), straight usage of end-to-end visual models often perform poorly. Recently proposed 'bottom-up and top-down' framework has achieved remarkable performance, which transforms visual classification task into text sequence classification task. However, in the 'top-down' phase, the long-distance dependence of text information still exists. On the other hand, in the 'bottom-up' phase, better detectors are also needed to further extract visual features. In this letter, the idea of 'feature adaptive weighting' (FAW), which was derived from the attention mechanism, is used in both phases to improve the overall performance. 'Self-correlation guided feature adaptive weighting' (S-FAW) is introduced in the first phase to improve building detection. In the second phase, 'cross-correlation guided feature adaptive weighting' (C-FAW) is used to enhance the connections between detected individual buildings. Experimental results show that the proposed FAWNet can effectively improve the performance of the two-phase framework in both phases and surpass the mainstream end-to-end models.
更多查看译文
AI 理解论文
溯源树
样例
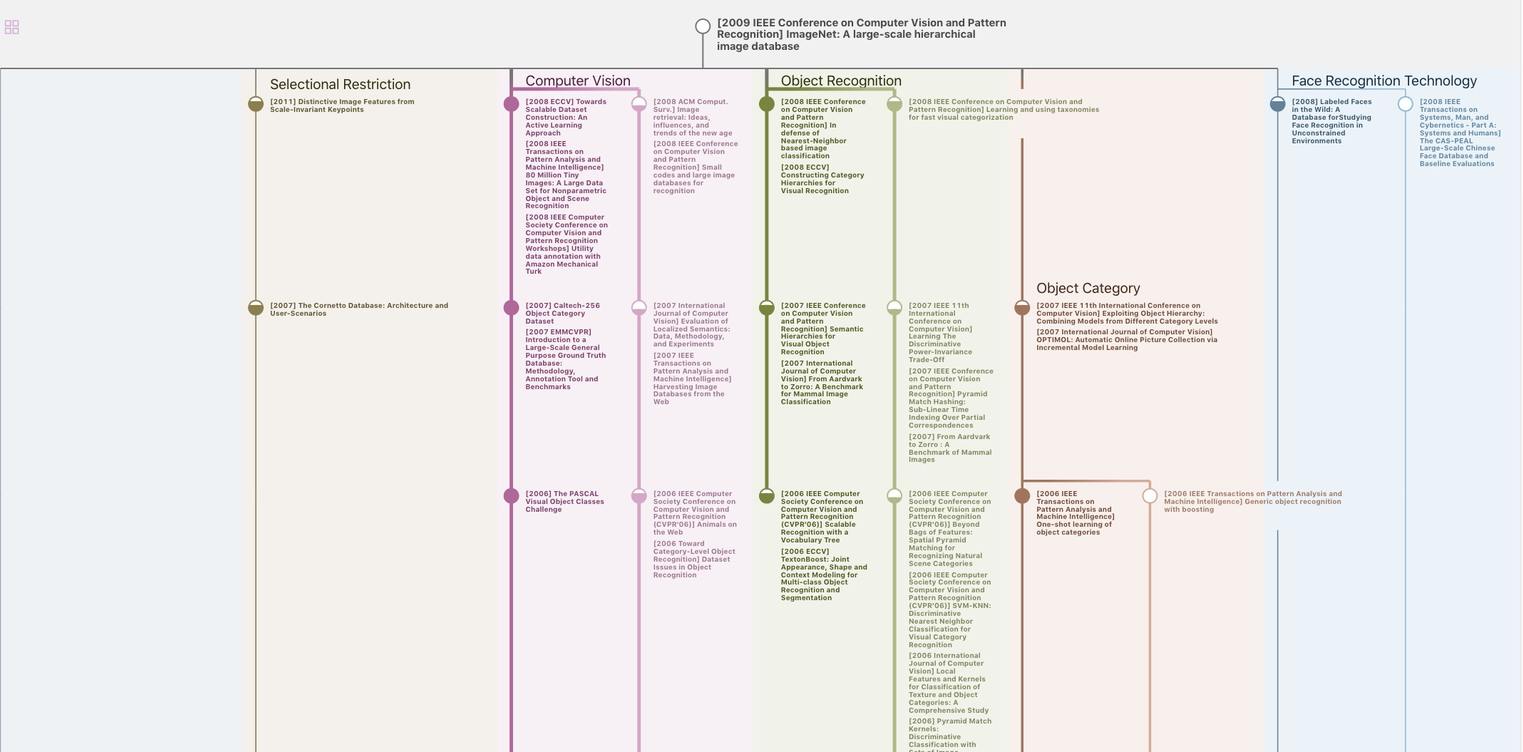
生成溯源树,研究论文发展脉络
Chat Paper
正在生成论文摘要