Generalization and learning rate of multi-class support vector classification and regression
INTERNATIONAL JOURNAL OF WAVELETS MULTIRESOLUTION AND INFORMATION PROCESSING(2022)
Abstract
The generalization is one of the main concerns of machine learning method. k-SVCR is an important multi-class classification algorithm, but there is no theoretical analysis on the generalization of k-SVCR algorithm up to now. Therefore, in this paper, we consider multi-class support vector classification and regression (MSVCR) algorithm. We first establish the generalization bounds of MSVCR based on uniformly ergodic Markovian chain (u.e.M.c.) samples, and obtain its fast learning rate of MSVCR. As applications, we estimate the generalization of MSVCR for independent and identically distributed (i.i.d.) observations and strongly mixing observations, respectively. We also propose a new MSVCR algorithm based on q-times Markovian resampling (MSVCR-Mar-q). The experimental studies indicate that compared to the classical k-SVCR and other multi-class SVM algorithms, the proposed algorithm not only has smaller misclassification rate, but also has less sampling and training total time.
MoreTranslated text
Key words
Multi-class classification, learning rate, k-support vector classification-regression, Markovian resampling
AI Read Science
Must-Reading Tree
Example
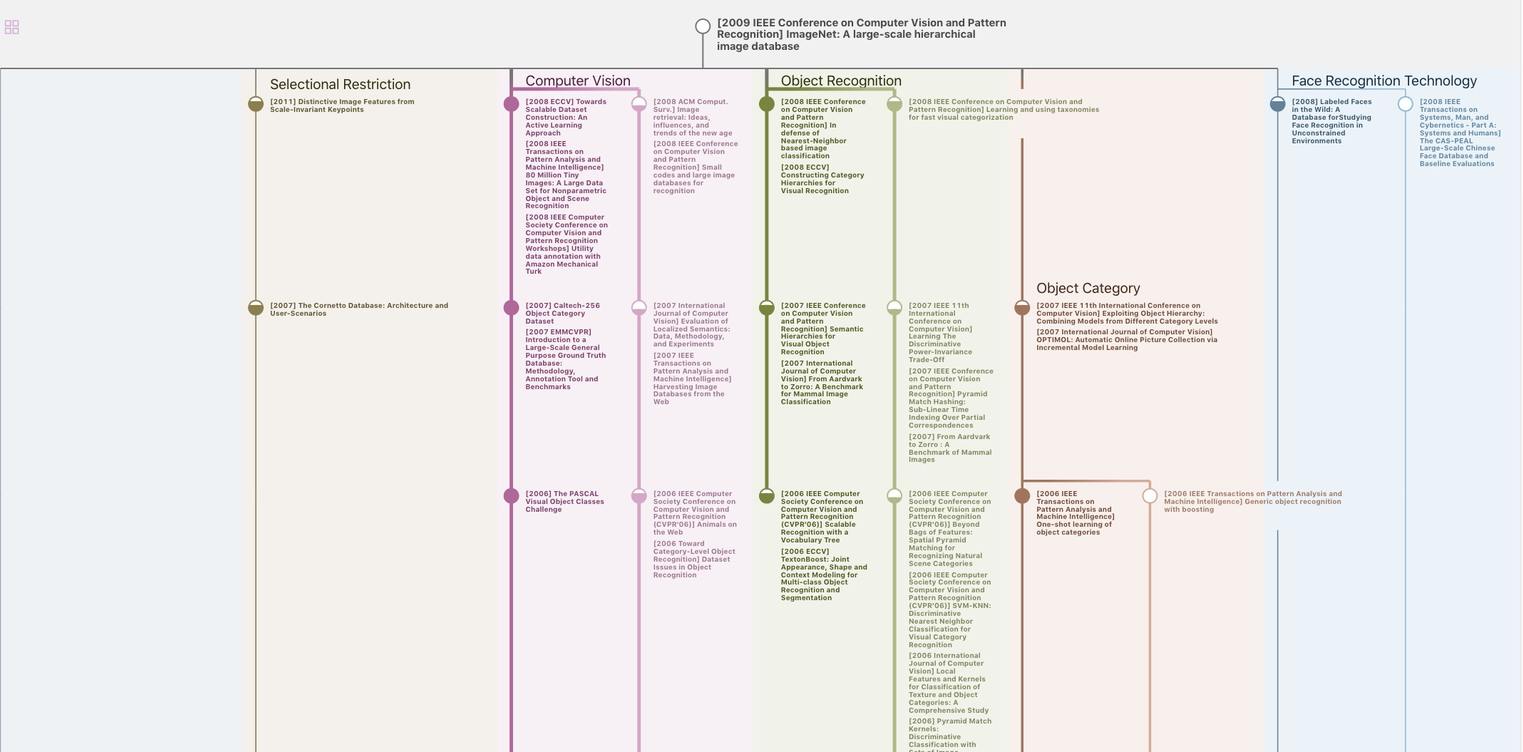
Generate MRT to find the research sequence of this paper
Chat Paper
Summary is being generated by the instructions you defined