Weighted contrastive learning using pseudo labels for facial expression recognition
VISUAL COMPUTER(2022)
Abstract
Supervised learning style requires a large amount of manually annotated data which are not readily available for facial attributes. Particularly annotations for emotion images are subjective and inconsistent, thus labeled facial expression data are insufficient, which cannot satisfy the demand for large-scale Facial Expression Recognition in deep learning era. In this paper, we propose a new self-supervised pretext task, called Weighted Contrastive Learning to make full use of extensive non-curated facial data, and learn the discriminative representation in a self-supervised way. Specifically, WeiCL learns the discriminative representation by two steps: (1) a pre-classification module is designed to build a specific batch for weighted contrastive learning by obtaining pseudo labels of the pre-training unlabeled facial data, and (2) a novel weighted contrastive objective function is proposed to reduce the intra-class variation and enlarge the distance among different instances by weighting the intermediate samples. Experiments on benchmark FER datasets validate the effect of our method. Results demonstrate that our approach outperforms current leading methods with 86.96% on RAF-DB and 71.42% on FER2013, under a rigorous evaluation protocol.
MoreTranslated text
Key words
Facial expression recognition,Representation learning,Contrastive learning,Self-supervised learning,Pseudo labels
AI Read Science
Must-Reading Tree
Example
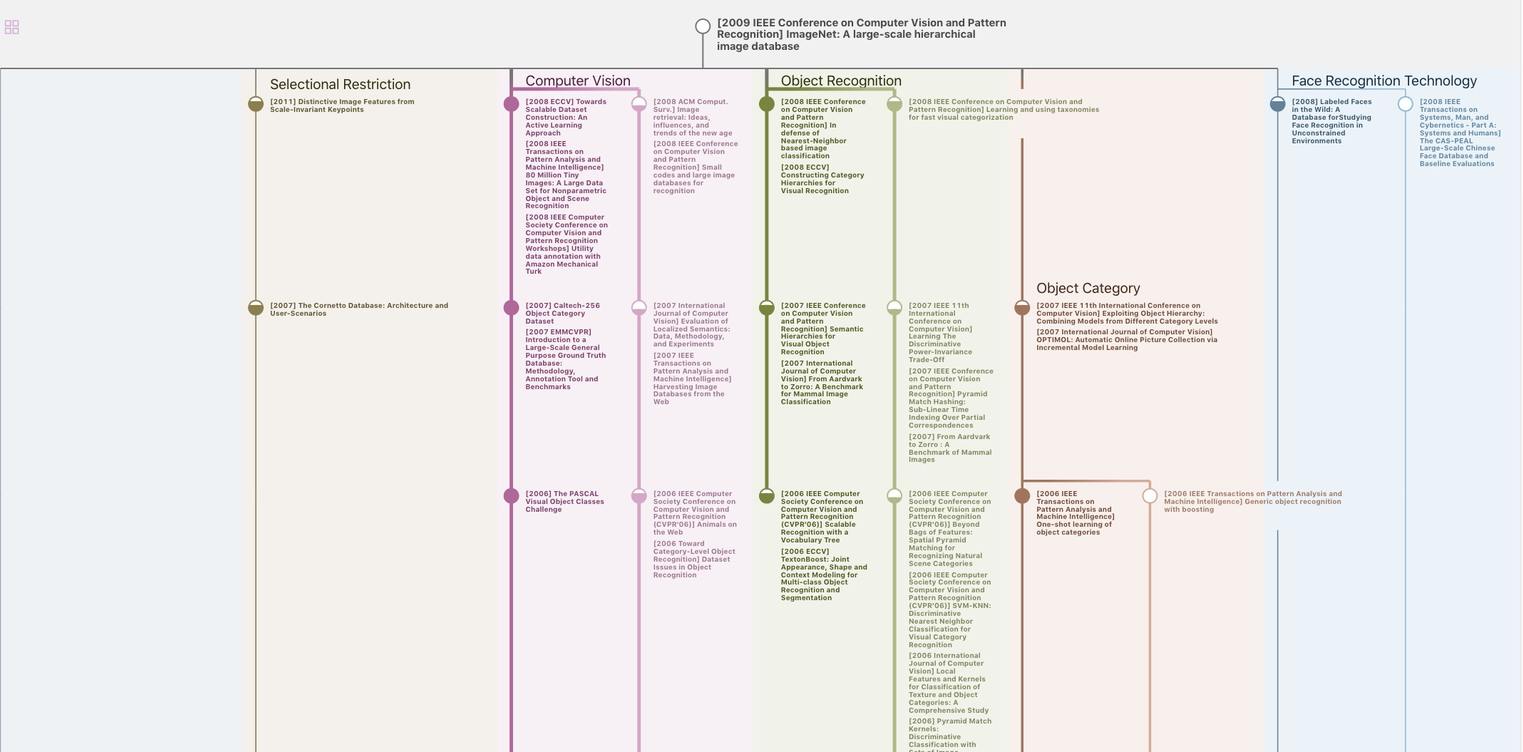
Generate MRT to find the research sequence of this paper
Chat Paper
Summary is being generated by the instructions you defined