PCTNet: 3D Point Cloud and Transformer Network for Monocular Depth Estimation
2022 10th International Conference on Information and Education Technology (ICIET)(2022)
摘要
Estimating dense depth map from one image is a challenging task for computer vision. Because the same image can correspond to the infinite variety of 3D spaces. Neural networks have gradually achieved reasonable results on this task with the continuous development of deep learning. But the depth estimation method based on monocular cameras still has a gap in accuracy compared with multi-view or sensor-based methods. Thus, this paper proposes to supplement a limited number of sparse 3D point clouds combined with transformer processing to increase the accuracy of the monocular depth estimation model. The sparse 3D point clouds are used as supplementary geometric information and the 3D point clouds are input into the network with the RGB image. After five times integration, the multi-scale features are extracted, and then the swin transformer block is used to process the output feature map of the main network, further improving the accuracy. Experiments demonstrate that our network achieves better results than the best method on the current most commonly used dataset for monocular depth estimation, NYU Depth V2. However, the qualitative results are also better than the best method.
更多查看译文
关键词
3D point cloud,swin transformer,monocular depth estimation
AI 理解论文
溯源树
样例
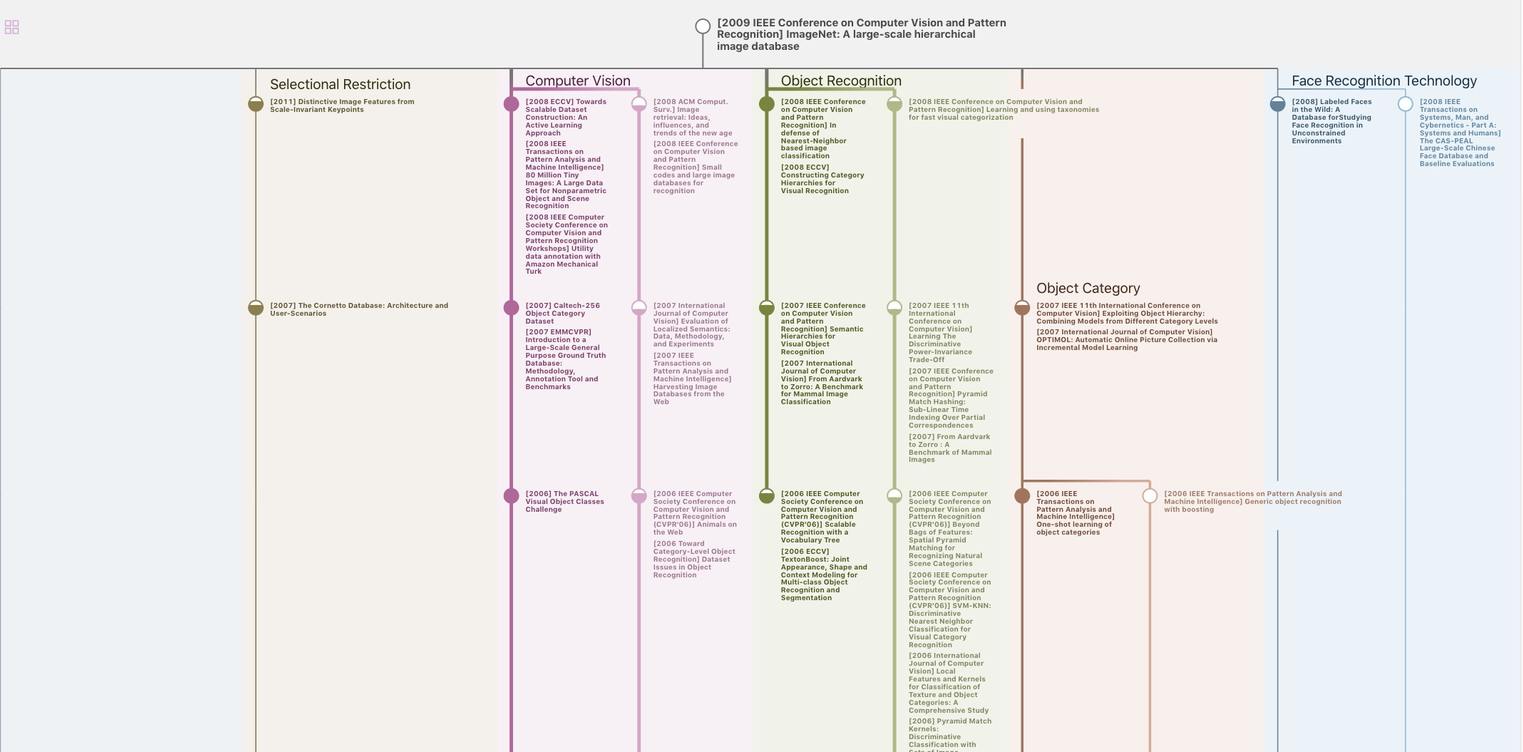
生成溯源树,研究论文发展脉络
Chat Paper
正在生成论文摘要