Forecasting the solar cycle 25 using a multistep Bayesian neural network
MONTHLY NOTICES OF THE ROYAL ASTRONOMICAL SOCIETY(2022)
摘要
Predicting the solar activity of upcoming cycles is crucial nowadays to anticipate potentially adverse space weather effects on the Earth's environment produced by coronal transients and traveling interplanetary disturbances. The latest advances in deep learning techniques provide new paradigms to obtain effective prediction models that allow to forecast in detail the evolution of cosmogeophysical time series. Because of the underlying complexity of the dynamo mechanism in the solar interior that is at the origin of the solar cycle phenomenon, the predictions offered by state-of-the-art machine learning algorithms represent valuable tools for our understanding of the cycle progression. As a plus, Bayesian deep learning is particularly compelling thanks to recent advances in the field that provide improvements in both accuracy and uncertainty quantification compared to classical techniques. In this work, a deep learning long short-term memory model is employed to predict the complete profile of Solar Cycle 25, thus forecasting also the advent of the next solar minimum. A rigorous uncertainty estimation of the predicted sunspot number is obtained by applying a Bayesian approach. Two different model validation techniques, namely the Train-Test split and the time series k-fold cross-validation, have been implemented and compared, giving compatible results. The forecasted peak amplitude is lower than that of the preceding cycle. Solar Cycle 25 will last 10.6 +/- 0.7 yr, reaching its maximum in the middle of the year 2024. The next solar minimum is predicted in 2030 and will be as deep as the previous one.
更多查看译文
关键词
methods: numerical, methods: statistical, Sun: activity, sunspots
AI 理解论文
溯源树
样例
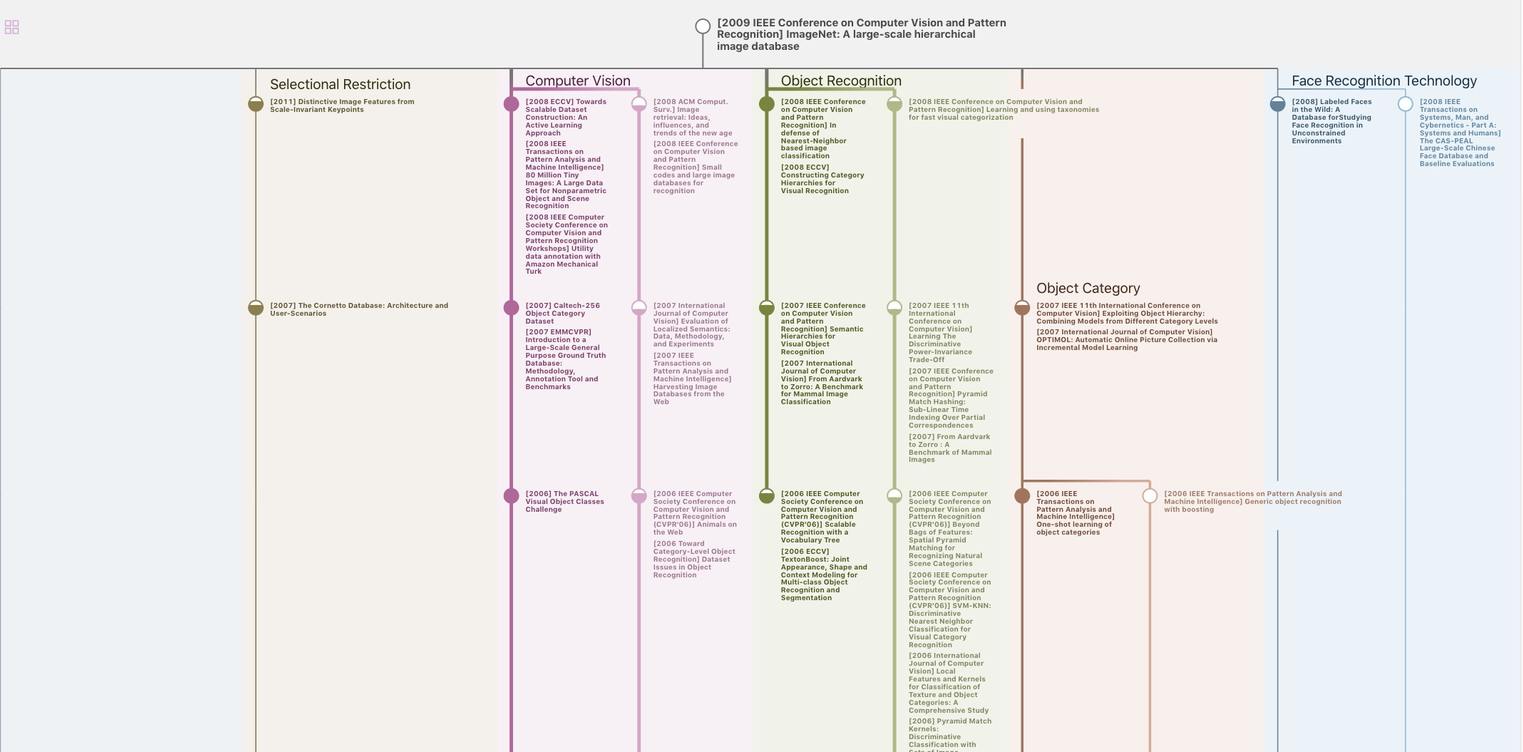
生成溯源树,研究论文发展脉络
Chat Paper
正在生成论文摘要