Statistical shape model of the spine fitting study: impact of clipping the latent representation
MEDICAL IMAGING 2022: IMAGE PROCESSING(2022)
摘要
Statistical shape models (SSMs) represent the distribution of labeled points across a training set of shapes. The standard practice for SSMs based on principal component analysis (PCA) is to use clipping, thresholding the latent representation so that all shapes lie within 3 standard deviations of the mean. This practice precludes the representation of shapes that are not well represented by the training set, constraining the model to realistic solutions, but making it impossible to work with shapes at the edges of the statistical population. In this study, we investigate the impact of clipping in a PCA-based SSM and whether using L2 regularization is a good replacement for clipping in the context of the automatic 2D to 3D reconstruction of the spine. We first show that using L2 regularization is equivalent to using a probabilistic PCA with two error variables, accounting for the suppression of the least important principal components and for the fact that the training set cannot perfectly represent all shapes at test time. Secondly, we use two data sets of 1746 and 768 patients with adolescent idiopathic scoliosis to study the effect of regularization, for different regularization weights and with or without clipping, for removing landmark detection errors using a simulated noise or a reconstruction pipeline. In both sets of experiments, we show that regularization removes noise in a way similar to clipping without preventing the reconstruction of out-of-distribution shapes, leading to outputs closer to ground truth, demonstrating that using a regularized SSM should be preferred to clipping.
更多查看译文
关键词
Satistical shape model,principal component analysis,clipping,scoliosis,spine,vertebrae,automatic reconstruction
AI 理解论文
溯源树
样例
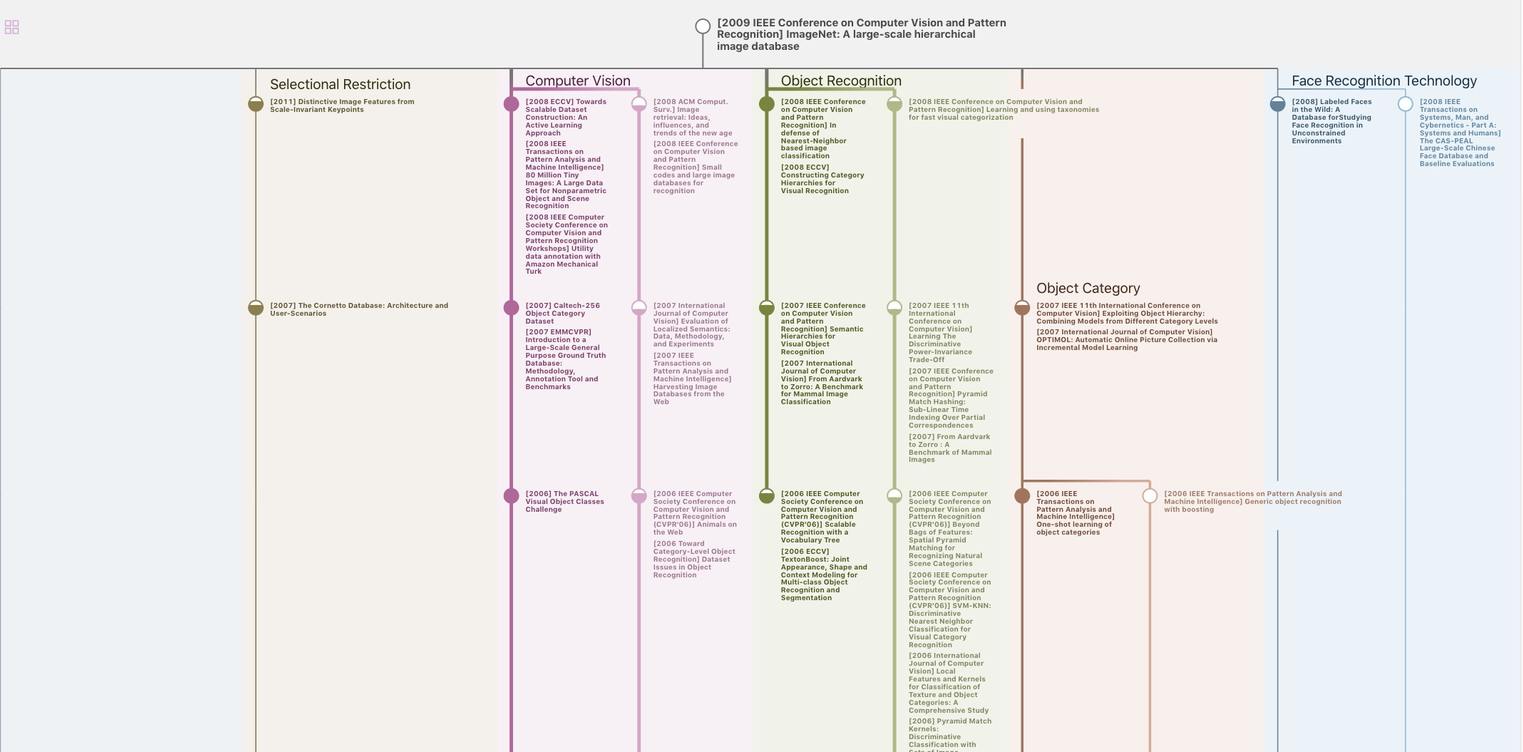
生成溯源树,研究论文发展脉络
Chat Paper
正在生成论文摘要