Inference and Local Influence Assessment in a Multifactor Skew-Normal Linear Mixed Model
MATHEMATICS(2022)
摘要
This work considers a multifactor linear mixed model under heteroscedasticity in random-effect factors and the skew-normal errors for modeling the correlated datasets. We implement an expectation-maximization (EM) algorithm to achieve the maximum likelihood estimates using conditional distributions of the skew-normal distribution. The EM algorithm is also implemented to extend the local influence approach under three model perturbation schemes in this model. Furthermore, a Monte Carlo simulation is conducted to evaluate the efficiency of the estimators. Finally, a real data set is used to make an illustrative comparison among the following four scenarios: normal/skew-normal errors and heteroscedasticity/homoscedasticity in random-effect factors. The empirical studies show our methodology can improve the estimates when the model errors follow from a skew-normal distribution. In addition, the local influence analysis indicates that our model can decrease the effects of anomalous observations in comparison to normal ones.
更多查看译文
关键词
EM algorithm, expectation-maximization algorithm, heteroscedasticity, Monte Carlo simulation, random effects, skew-normal, variance components, applied mathematics, Linear mixed models
AI 理解论文
溯源树
样例
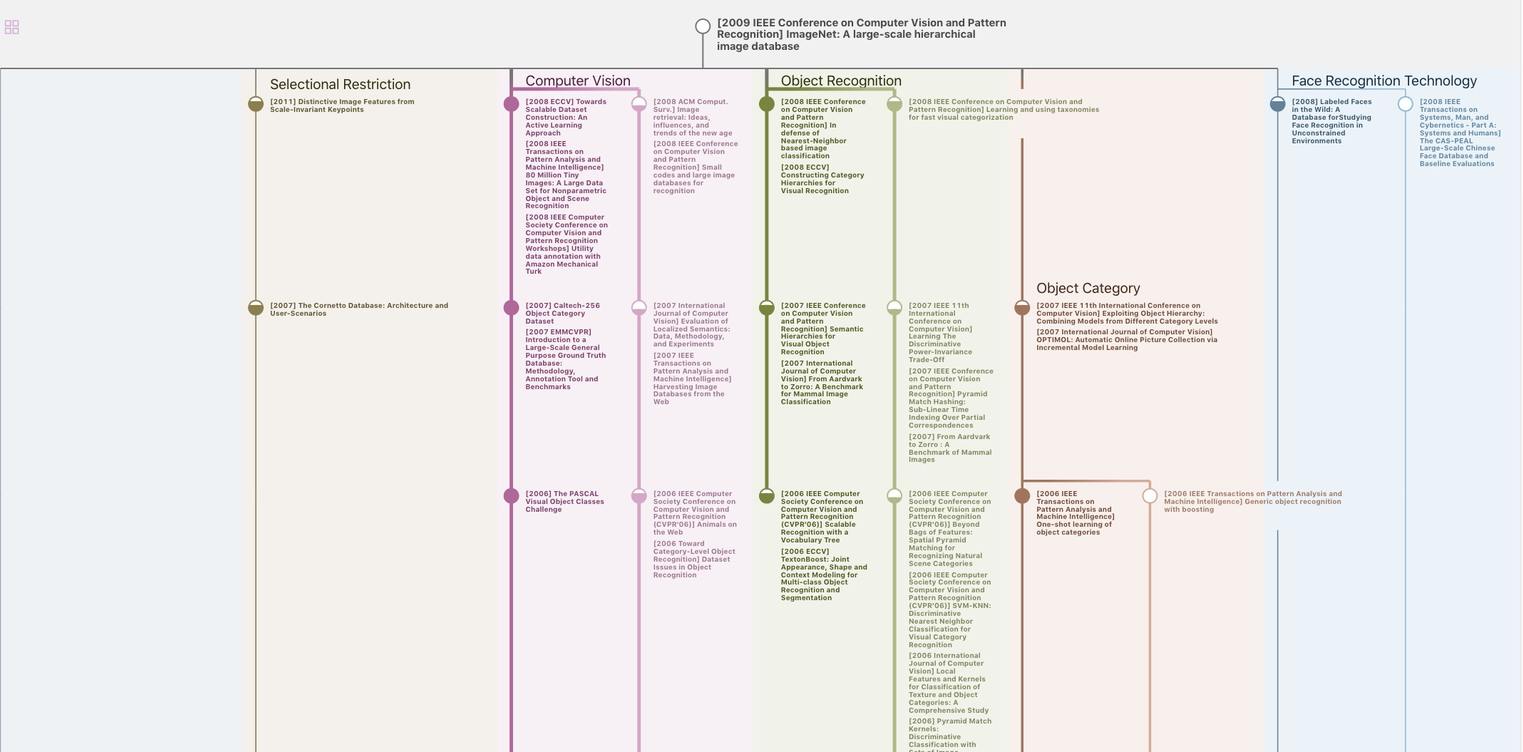
生成溯源树,研究论文发展脉络
Chat Paper
正在生成论文摘要