Detecting COVID-19 from Respiratory Sound Recordings with Transformers
MEDICAL IMAGING 2022: COMPUTER-AIDED DIAGNOSIS(2022)
摘要
Auscultation is an established technique in clinical assessment of symptoms for respiratory disorders. Auscultation is safe and inexpensive, but requires expertise to diagnose a disease using a stethoscope during hospital or office visits. However, some clinical scenarios require continuous monitoring and automated analysis of respiratory sounds to pre-screen and monitor diseases, such as the rapidly spreading COVID-19. Recent studies suggest that audio recordings of bodily sounds captured by mobile devices might carry features helpful to distinguish patients with COVID-19 from healthy controls. Here, we propose a novel deep learning technique to automatically detect COVID-19 patients based on brief audio recordings of their cough and breathing sounds. The proposed technique first extracts spectrogram features of respiratory recordings, and then classifies disease state via a hierarchical vision transformer architecture. Demonstrations are provided on a crowdsourced database of respiratory sounds from COVID-19 patients and healthy controls. The proposed transformer model is compared against alternative methods based on state-of-the-art convolutional and transformer architectures, as well as traditional machine-learning classifiers. Our results indicate that the proposed model achieves on par or superior performance to competing methods. In particular, the proposed technique can distinguish COVID-19 patients from healthy subjects with over 94% AUC.
更多查看译文
关键词
COVID-19,respiratory,sound,breathing,cough,transformer
AI 理解论文
溯源树
样例
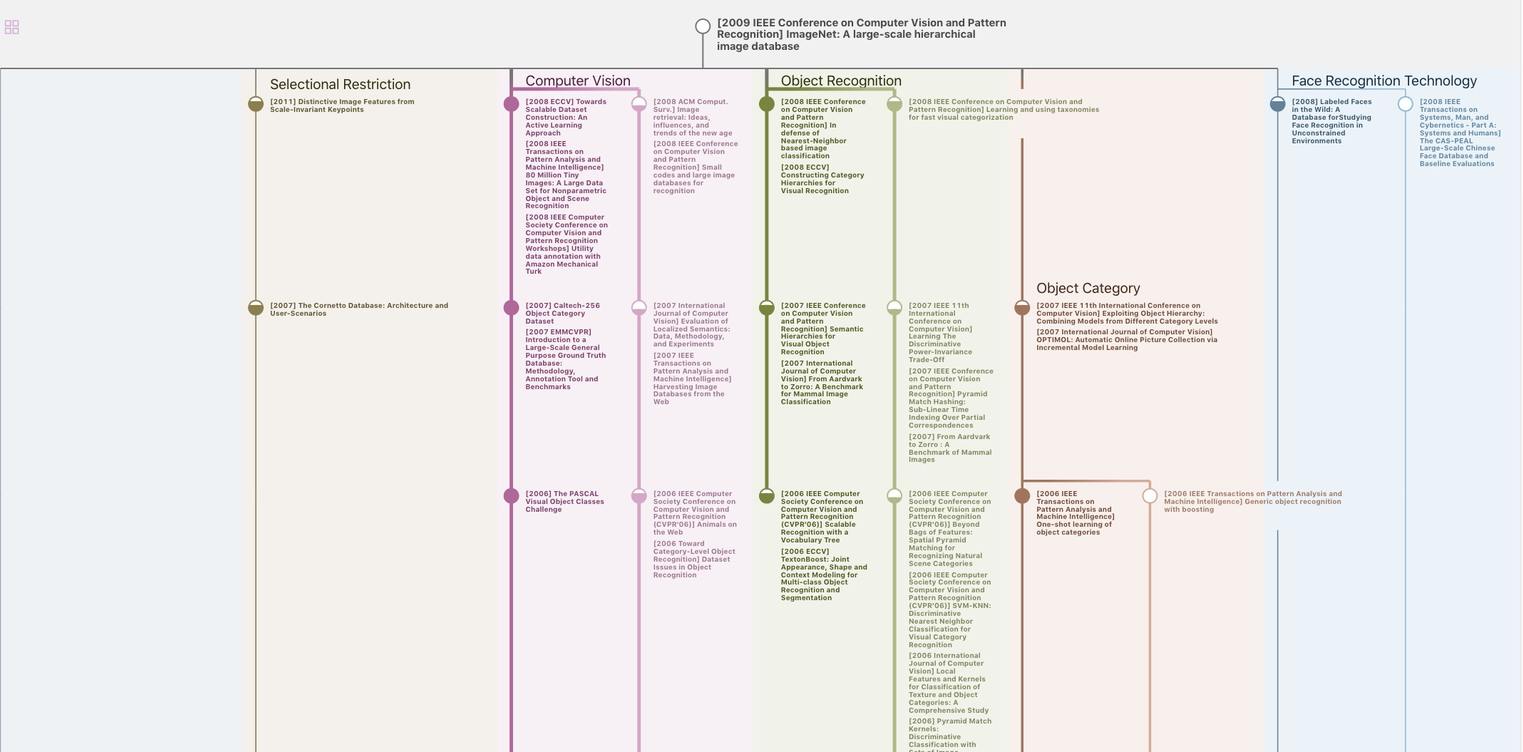
生成溯源树,研究论文发展脉络
Chat Paper
正在生成论文摘要