Selecting Training Samples for Ovarian Cancer Classification via a Semi-supervised Clustering Approach
MEDICAL IMAGING 2022: DIGITAL AND COMPUTATIONAL PATHOLOGY(2022)
摘要
Machine learning techniques have shown great promise in digital pathology. However, a major bottleneck is the difficulty of annotating necessary amount of tissue to deal with several variability factors, namely chemical fixation, sample slicing, or staining. Usually, models are trained using sets of annotated small image patches, but then, the number of required patches may increase exponentially and yet they must represent such variability. This paper presents a method for automatic sample selection to train a classifier for ovarian cancer by integrating a novel soft clustering strategy. The method starts by classifying a large set of patches with a previously trained classifier and divide patches from the cancer class as highly and moderately confident. An unsupervised selection of moderately confident patches by a Probabilistic Latent Semantic Analysis (PLSA), picks samples from relevant and meaningful groups with maximum within-group variance. A new model is re-trained using the highly confident patches together with patches obtained from the associated PLSA. This strategy outperforms a model trained with a larger set of annotated patches while the training times and the number of samples are much more smaller. The strategy was evaluated in a set of patches from 18 patients with Serous Ovarian Cancer, obtaining a reduction of 54.62% in the training time and 73.66% in the number of samples, while recall rate improved from 0.69 to 0.73.
更多查看译文
关键词
Pathologist navigation, Decision Support, Probabilistic Latent Semantic Analysis, Serous ovarian Cancer
AI 理解论文
溯源树
样例
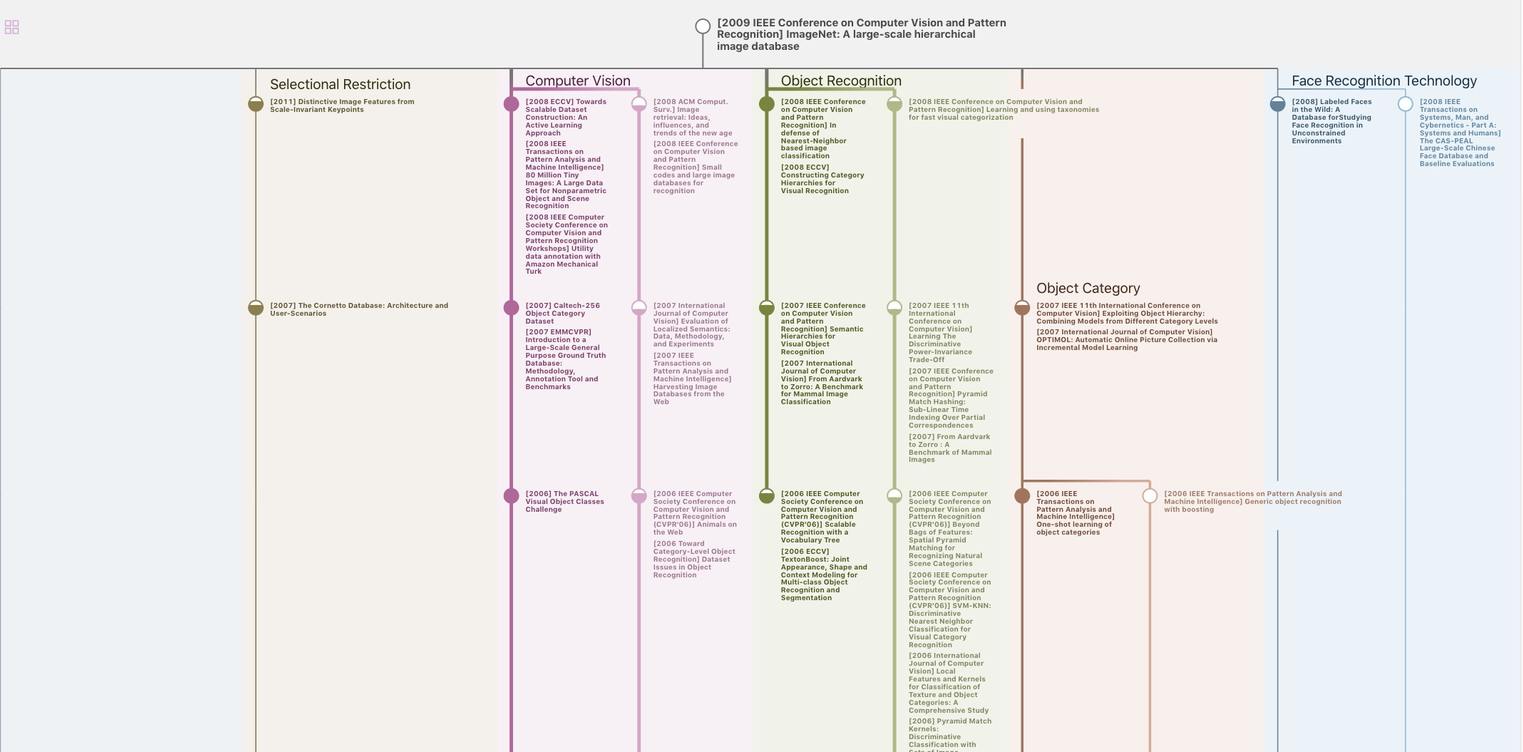
生成溯源树,研究论文发展脉络
Chat Paper
正在生成论文摘要