Fusion of multiple deep convolutional neural networks (DCNNs) for improved segmentation of lung nodules in CT images
MEDICAL IMAGING 2022: COMPUTER-AIDED DIAGNOSIS(2022)
摘要
We are developing DCNN-based methods for the segmentation of lung nodules of a wide variety of sizes, shapes, and margins. In this study, we developed several fusion methods for hybridizing multiple DCNNs with different structures to improve segmentation accuracy. Two groups of fusion methods that combined the output information from our previously designed shallow and deep U-shape based deep learning models (U-DL) were compared. Group-1 denotes the late fusion (LF) methods that concatenated the feature maps output from the last layers (before a sigmoid activation layer) of the two U-DLs. Group-2 denotes early fusion (EF) methods that combined multi-scale output information from the encoders and decoders, or decoders alone, of the two U-DLs. A set of 883 cases from the LIDC-IDRI database which contained lung nodules manually marked by at least two radiologists was selected for this study. We split the data into 683 training and validation and 200 independent test cases. The multiple DCNNs with the fusion method were trained simultaneously and end-to-end. The baseline LF-1 method using pre-defined thresholds achieved an average DICE coefficient of 0.718 +/- 0.159. The newly developed LF method using Squeeze and Excitation Attention Block (SEAB) followed by a sigmoid activation layer (LF-4), and two Convolutional Block Attention Modules (CBAM) based EF methods that combined multiscale information from the decoders alone (EF-2) or from both the encoders and decoders (EF-3) of the two U-DLs, achieved significantly (p<0.05) better performance with average DICE coefficients of 0.745 +/- 0.135, 0.745 +/- 0.142, and 0.747 +/- 0.142, respectively.
更多查看译文
关键词
Computer-aided diagnosis,lung nodule,Deep learning
AI 理解论文
溯源树
样例
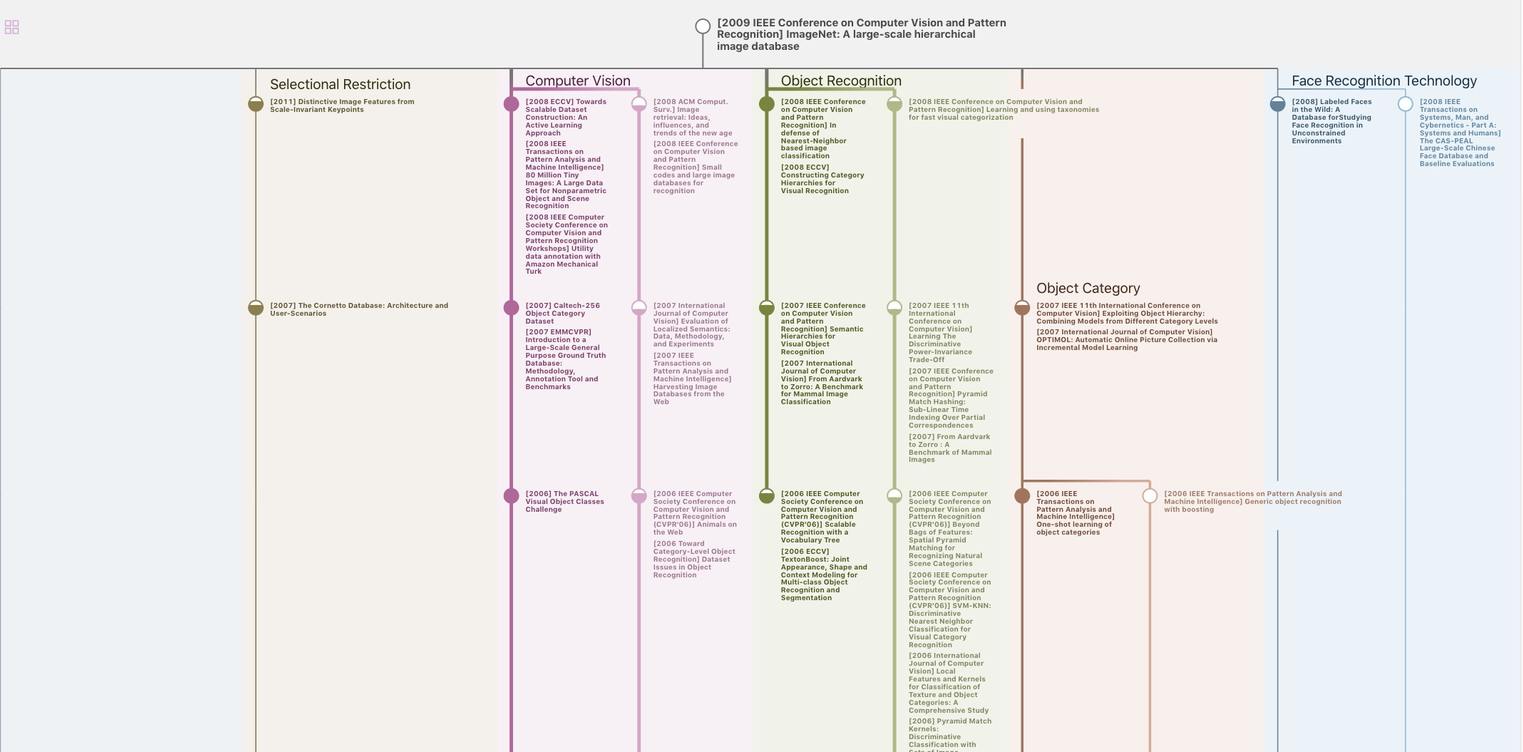
生成溯源树,研究论文发展脉络
Chat Paper
正在生成论文摘要