Different Approaches to Aggregate Stability Estimation in the Semi-Arid Climate Conditions
COMMUNICATIONS IN SOIL SCIENCE AND PLANT ANALYSIS(2023)
摘要
Aggregate stability, which is an important feature among soil physical quality indicators, has a direct or indirect effect on many soil properties. In this study, some physical (sand, silt, and clay), chemical [organic carbon, electrical conductivity (EC), lime (CaCO3), exchangeable calcium + magnesium (Ca + Mg), sodium (Na), free iron and aluminum oxide (Fe2O3 and Al2O3)], and biological (soil respiration, enzyme analysis, microbial biomass carbon) properties of soil were determined. Aggregate stability estimation was performed with (1) multivariate regression equation and (2) artificial neural networks mediated by different activation functions (Sigmoid, Purelin, and Tansig) and algorithms. Properties highly correlated with aggregate stability were EC (r:0.35; p < .01), Urease (r: 0.50; p < .01), Ca + Mg (r: 0.38; p < .01), and clay (r: 0.51; p < .01) was found. Regression equations with an accuracy of 63.6% were obtained by using the clay, CaCO3%, Ca + Mg, EC, and Urease enzyme properties of the soils. With artificial neural networks, the lowest root mean squarer (RMSE) values were obtained in Tansig and Sigmoid activation functions, Levenberg-Marquardt algorithm (LM) and Bayesian correction-based (BR) learning algorithms. The RMSE values ranged from 1.73% to 33.14%, 1.70 to 28.23%, and 5.66 to 29.77% for Tansig, Sigmoid, and Purine functions, respectively. As a result of the study, it was observed that more successful predictions were obtained with artificial neural networks.
更多查看译文
关键词
Artificial neural networks, structure, soil properties, multivariate analysis, algorithm
AI 理解论文
溯源树
样例
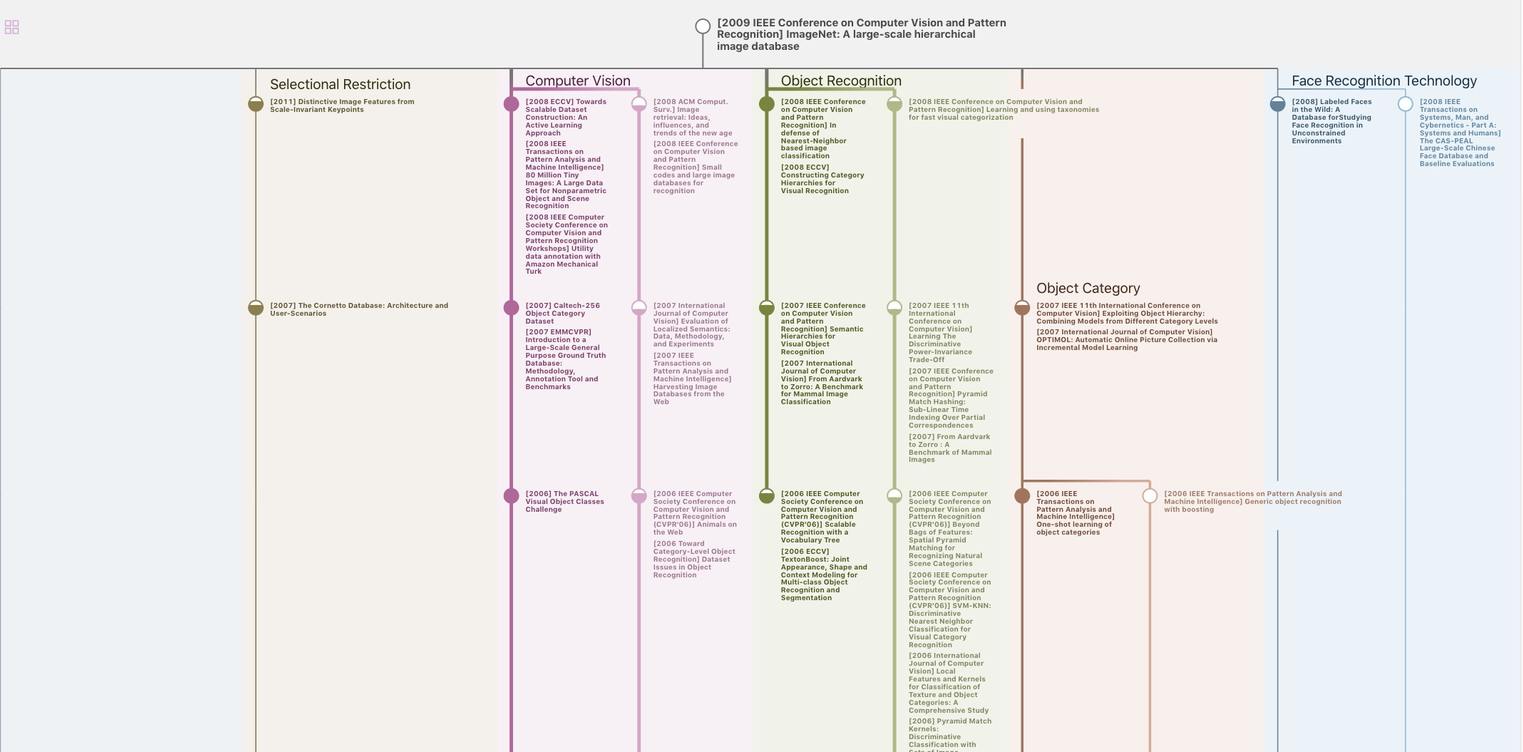
生成溯源树,研究论文发展脉络
Chat Paper
正在生成论文摘要