AGE ESTIMATION FROM SLEEP USING DEEP LEARNING PREDICTS LIFE EXPECTANCY
SLEEP(2022)
摘要
Abstract Introduction Sleep disturbances increase with age and are predictors of mortality. However, summary metrics typically derived in sleep clinics from gold standard clinical analysis of polysomnograms (PSGs) only represent a very small fraction of data collected. In this study, we designed deep neural networks that estimate age as a proxy for overall health using full PSG signals. Age estimation was next used to evaluate association to mortality risk. Methods Aging was modeled using 2,500 PSGs and tested in 10,808 PSGs from men and women in 7 different cohorts aged between 20 and 90. The deep neural network was trained using as a regression model of age in the 2,500 PSGs roughly uniformly distributed across 6 to 90 years. The estimates of the network were interpreted using Gradient SHAP, which attributes relevance scores to the input in terms of the age estimate. The association between age estimate error (AEE), which is the residual of the estimate, and mortality risk was investigated with Cox proportional hazards models that adjusted for demographics, sleep, and health covariates. Results Ages were estimated with a mean absolute error of 5.81 ± 1.18 years, while a linear regression model using basic sleep scoring measures had an error of 15.10 ± 6.48 years. Interpretation of the network revealed that patterns such as arousal, sleep apnea, and sleep stage transitions contribute to the age estimate. Each 10-year increment in AEE was associated with increased all-cause mortality rate of 28 % (95% confidence interval: 19–38 %) and cardiovascular mortality rate of 38 % (95% confidence interval: 19 – 59 %). An increase from -10 to +10 years in AEE translates to an estimated decreased life expectancy of 6.21 years (95% confidence interval: 4.31–8.21 years). Conclusion Greater AEE was mostly reflected in increased sleep fragmentation, suggesting this is an important biomarker of future health independent of sleep apnea. Support (If Any) The Klarman Family Foundation and grants HL46380, M01 RR00080-39, T32-HL07567, RO1-46380, U01HL53916, U01HL53931, U01HL53934, U01HL53937, U01HL64360, U01HL53938, U01HL53940, U01HL53941, U01HL63463, 38-PM-07, R01HL62252, R01AG036838, R01AG058680, 1UL1RR025011, U01AG027810, U01AG042124, U01AG042139, U01AG042140, U01AG042143, U01AG042145, U01AG042168, U01AR066160, UL1TR000128, R01HL071194, R01HL070848, R01HL070847, R01HL070842, R01HL070841, R01HL070837, R01HL070838, R01HL070839, R24HL114473, and 75N92019R002.
更多查看译文
AI 理解论文
溯源树
样例
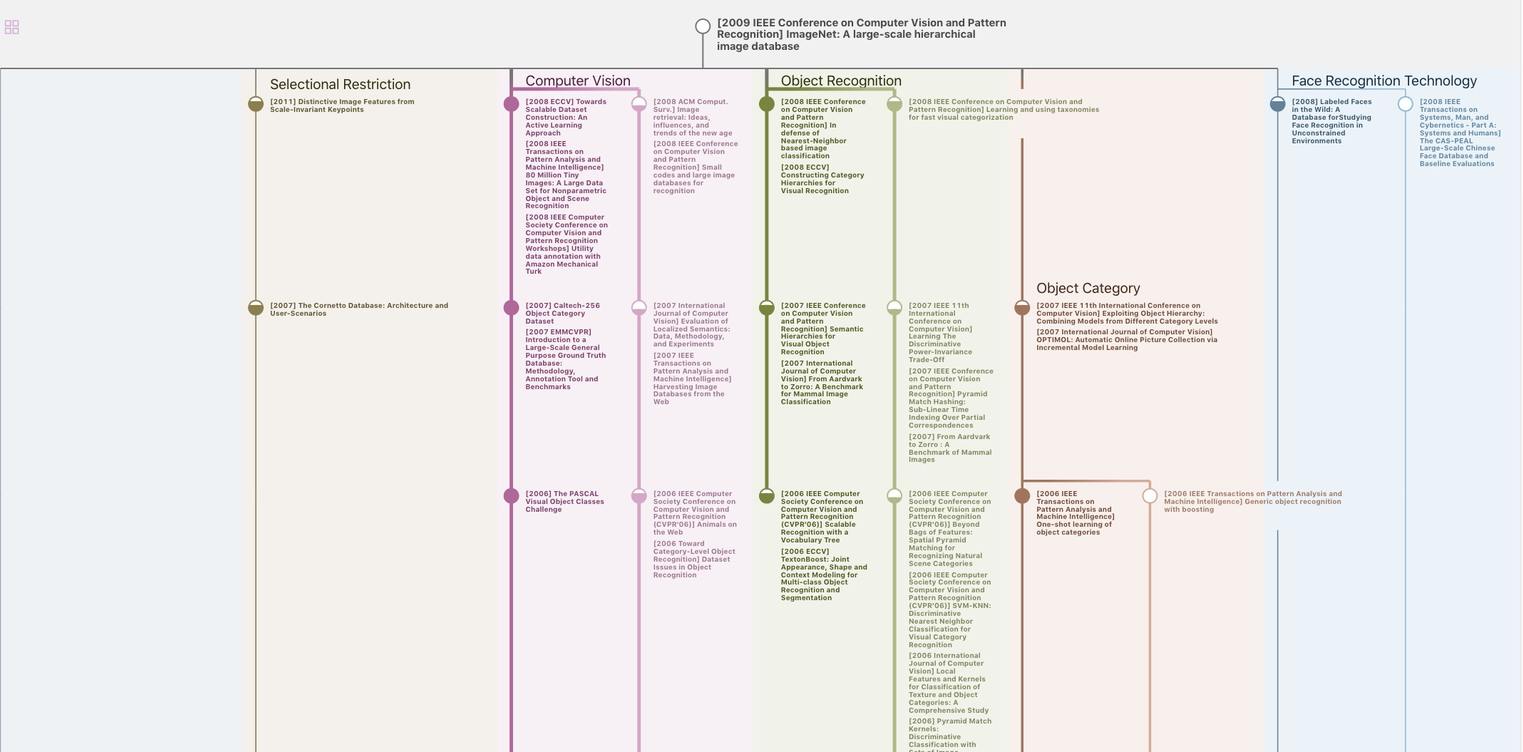
生成溯源树,研究论文发展脉络
Chat Paper
正在生成论文摘要