Multibranch 3D-Dense Attention Network for Hyperspectral Image Classification
IEEE ACCESS(2022)
摘要
The convolutional neural network (CNN) is widely used in the task of hyperspectral image (HSI) classification. However, for the HSI of three-dimensional characteristics, the 2D CNN-based methods will result in losing spatial-spectral information. To solve this problem, this paper proposes a multi-branch 3D-densely connected network for HSI classification. This network is able to reuse features to fully exploit the shallow spatial-spectral information of HSI. Meanwhile, the convolutional kernels of different sizes are used to extract multi-scale spatial-spectral features. Subsequently, spatial attention mechanisms are used to emphasize spatial features and increase the diversity of features. By introducing global average pooling instead of a fully connected layer, the number of parameters in the whole network will be reduced. In order to verify the performance of the proposed method, the experiment results conducted in the Indian Pines, the University of Pavia, Salinas Valley and Houston 2013 datasets show that the proposed method is better than the state-of-the-art methods.
更多查看译文
关键词
Feature extraction, Three-dimensional displays, Convolution, Convolutional neural networks, Kernel, Hyperspectral imaging, Training, Hyperspectral image classification, spatial-spectral feature, spatial attention mechanism, 3D CNN
AI 理解论文
溯源树
样例
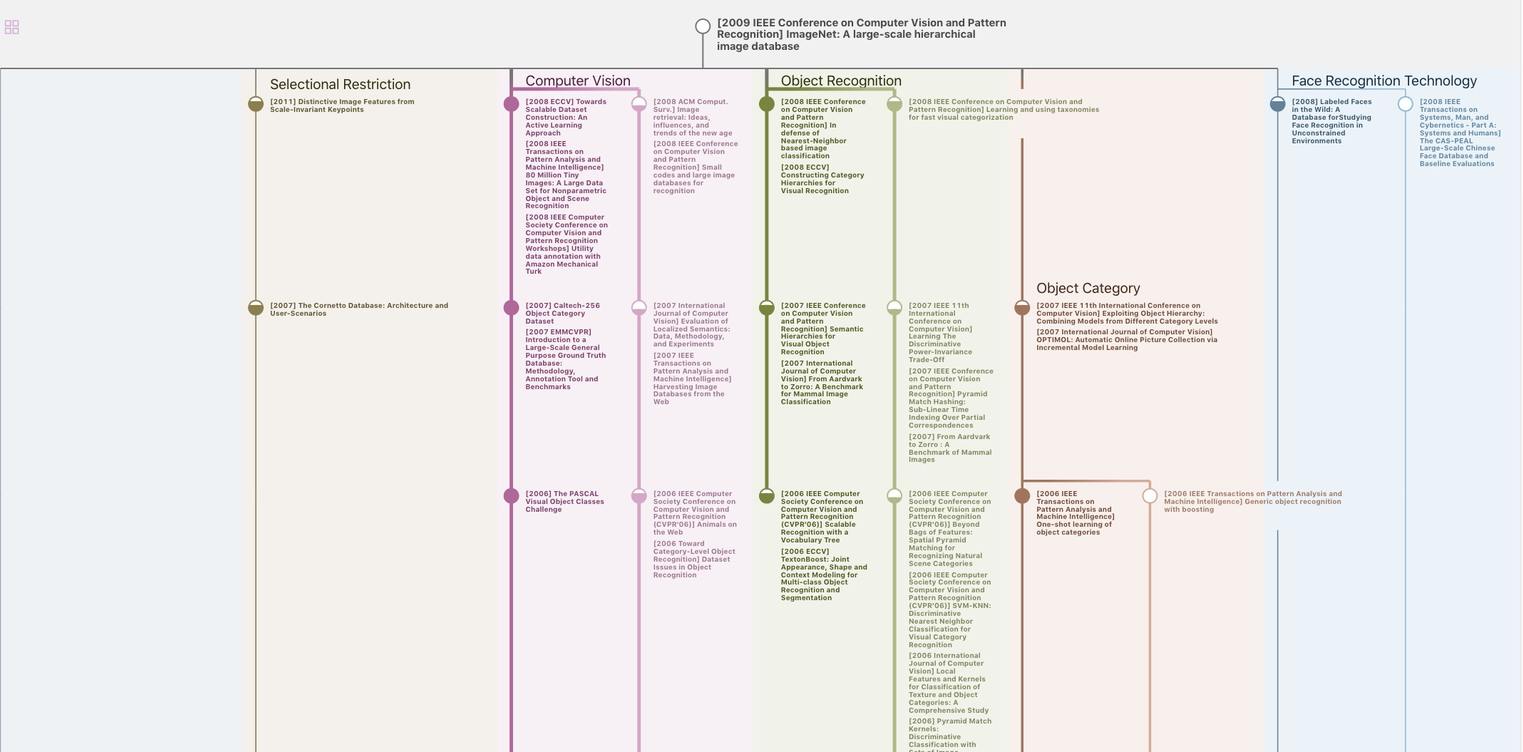
生成溯源树,研究论文发展脉络
Chat Paper
正在生成论文摘要