Visual Inspection Method for Metal Rolls Based on Multi-Scale Spatial Location Feature
SYMMETRY-BASEL(2022)
摘要
Metal rolls in a non-ferrous-metal manufacturing workshop manifest the characteristics of symmetry, multiple scales and mutual covering, which poses great challenges for metal roll detection. To solve this problem, firstly, an efficient attention mechanism algorithm named ECLAM (efficient capture location attendant model) is proposed for capturing spatial position features efficiently, to obtain complete location information for metal rolls in a complex environment. ECLAM can improve the ability to extract the spatial features of backbone networks and reduce the influence of the non-critical background. In addition, in order to give feature maps a larger receptive field and improve the weight of location information in multi-scale feature maps, a nonlinear feature fusion module named LFFM (location feature fusion module) is used to fuse two adjacent feature images. Finally, a multi-scale object detection network named L-MSNet (location-based multi-scale object detection network) based on the combination of ECLAM and LFFM is proposed and used to accurately detect multi-scale metal rolls. In the experiments, multi-scale metal roll images are collected from an actual non-ferrous-metal manufacturing workshop. On this basis, a pixel-level image dataset is constructed. Comparative experiments show that, compared with other object detection methods, L-MSNet can detect multi-scale metal rolls more accurately. The average accuracy is improved by 2% to 5%, and the average accuracy of small and medium-sized objects is also significantly improved by 3% to 6%.
更多查看译文
关键词
warehouse management, deep learning, object detection, attention mechanisms, feature fusion
AI 理解论文
溯源树
样例
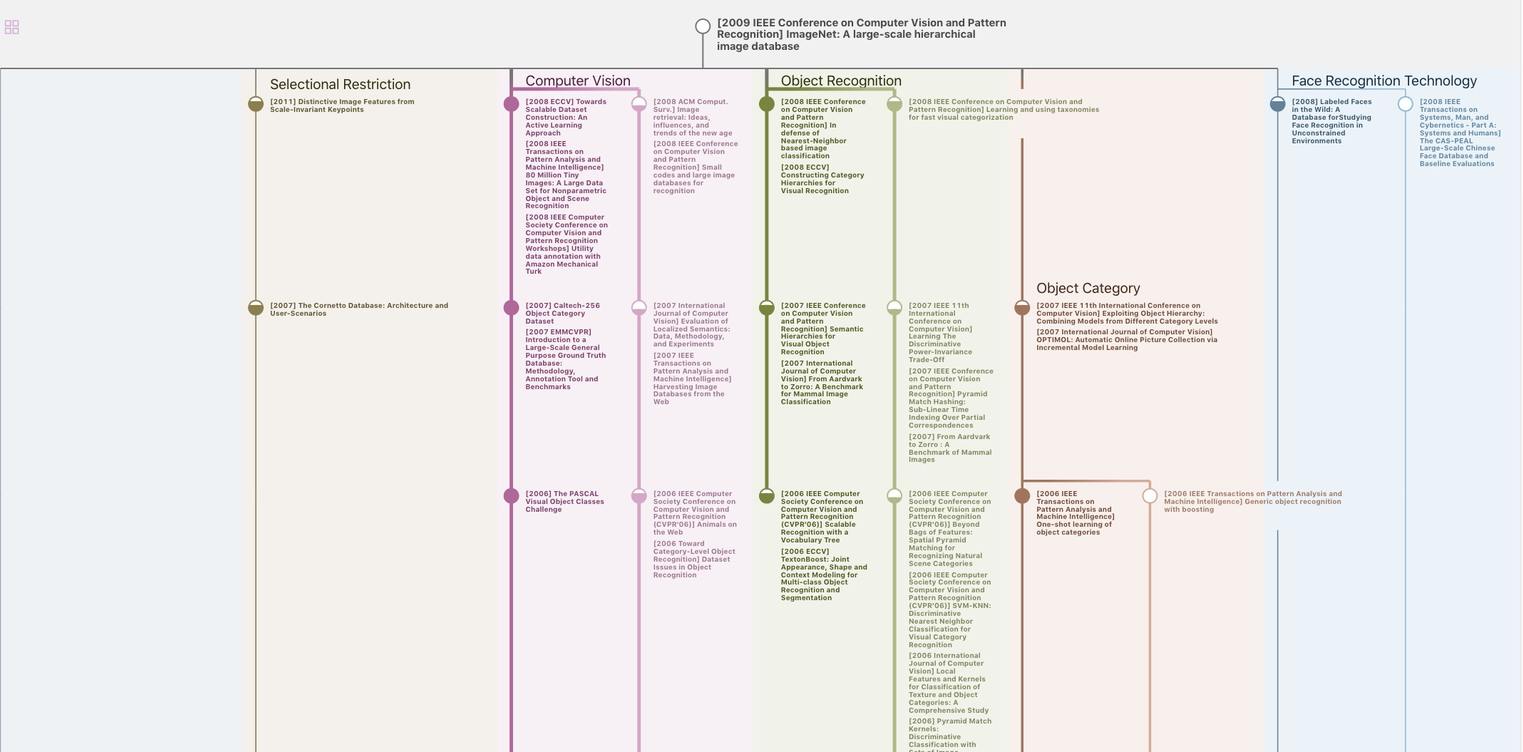
生成溯源树,研究论文发展脉络
Chat Paper
正在生成论文摘要