Imperceptible Image Steganography Using Symmetry-Adapted Deep Learning Techniques
SYMMETRY-BASEL(2022)
摘要
Digital image steganography is the process of embedding information within a cover image in a secure, imperceptible, and recoverable way. This research extends a symmetry-adapted deep-learning approach to identify hidden patterns of images using two-dimensional convolutional neural networks (CNN). The proposed method (SteGuz) uses three CNNs to implement the different phases of the steganography process on digital image covers. SteGuz introduced a gain function, based on several image similarity metrics, to maximize the imperceptibility of the hiding process. Using 10 different pairs of cover-secret images, the performance of the proposed method was measured in terms of standard metrics such as peak signal to noise ratio (PSNR) and structured similarity index measurement (SSIM). The results showed that the proposed methodology outperformed the original method in terms of both imperceptibility and recoverability. In addition, when compared with some existing methods, SteGuz proved the outstanding performance of achieving a very high PSNR value while maintaining high accuracy of the extracted image.
更多查看译文
关键词
deep learning, hiding, extraction, steganography, convolutional neural network, image, PSNR, SSIM
AI 理解论文
溯源树
样例
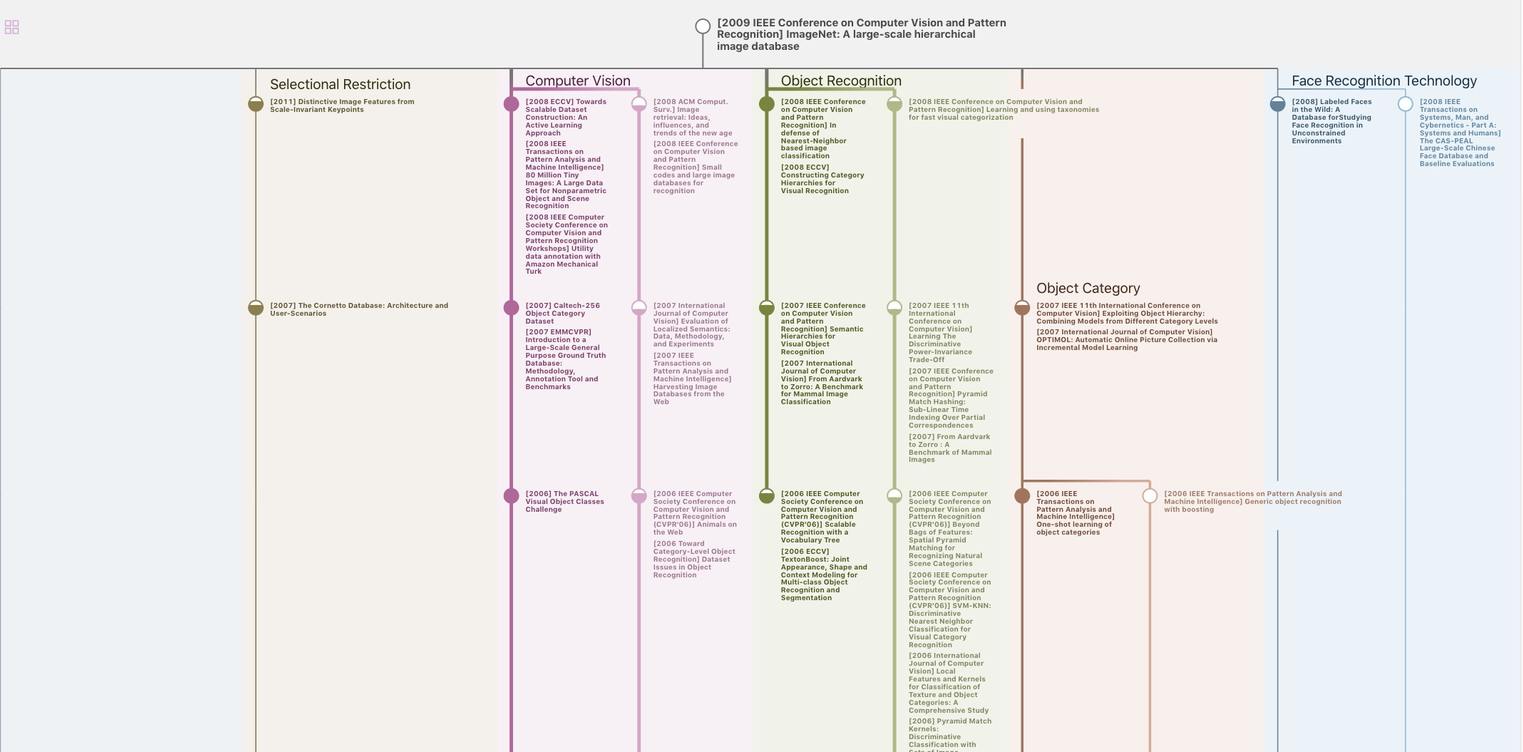
生成溯源树,研究论文发展脉络
Chat Paper
正在生成论文摘要