A Comparison of Ensemble and Deep Learning Algorithms to Model Groundwater Levels in a Data-Scarce Aquifer of Southern Africa
HYDROLOGY(2022)
摘要
Machine learning and deep learning have demonstrated usefulness in modelling various groundwater phenomena. However, these techniques require large amounts of data to develop reliable models. In the Southern African Development Community, groundwater datasets are generally poorly developed. Hence, the question arises as to whether machine learning can be a reliable tool to support groundwater management in the data-scarce environments of Southern Africa. This study tests two machine learning algorithms, a gradient-boosted decision tree (GBDT) and a long short-term memory neural network (LSTM-NN), to model groundwater level (GWL) changes in the Shire Valley Alluvial Aquifer. Using data from two boreholes, Ngabu (sample size = 96) and Nsanje (sample size = 45), we model two predictive scenarios: (I) predicting the change in the current month's groundwater level, and (II) predicting the change in the following month's groundwater level. For the Ngabu borehole, GBDT achieved R-2 scores of 0.19 and 0.14, while LSTM achieved R-2 scores of 0.30 and 0.30, in experiments I and II, respectively. For the Nsanje borehole, GBDT achieved R-2 of -0.04 and -0.21, while LSTM achieved R-2 scores of 0.03 and -0.15, in experiments I and II, respectively. The results illustrate that LSTM performs better than the GBDT model, especially regarding slightly greater time series and extreme GWL changes. However, closer inspection reveals that where datasets are relatively small (e.g., Nsanje), the GBDT model may be more efficient, considering the cost required to tune, train, and test the LSTM model. Assessing the full spectrum of results, we concluded that these small sample sizes might not be sufficient to develop generalised and reliable machine learning models.
更多查看译文
关键词
groundwater levels, machine learning, gradient boosting decision trees, long short-term memory neural networks, Southern Africa
AI 理解论文
溯源树
样例
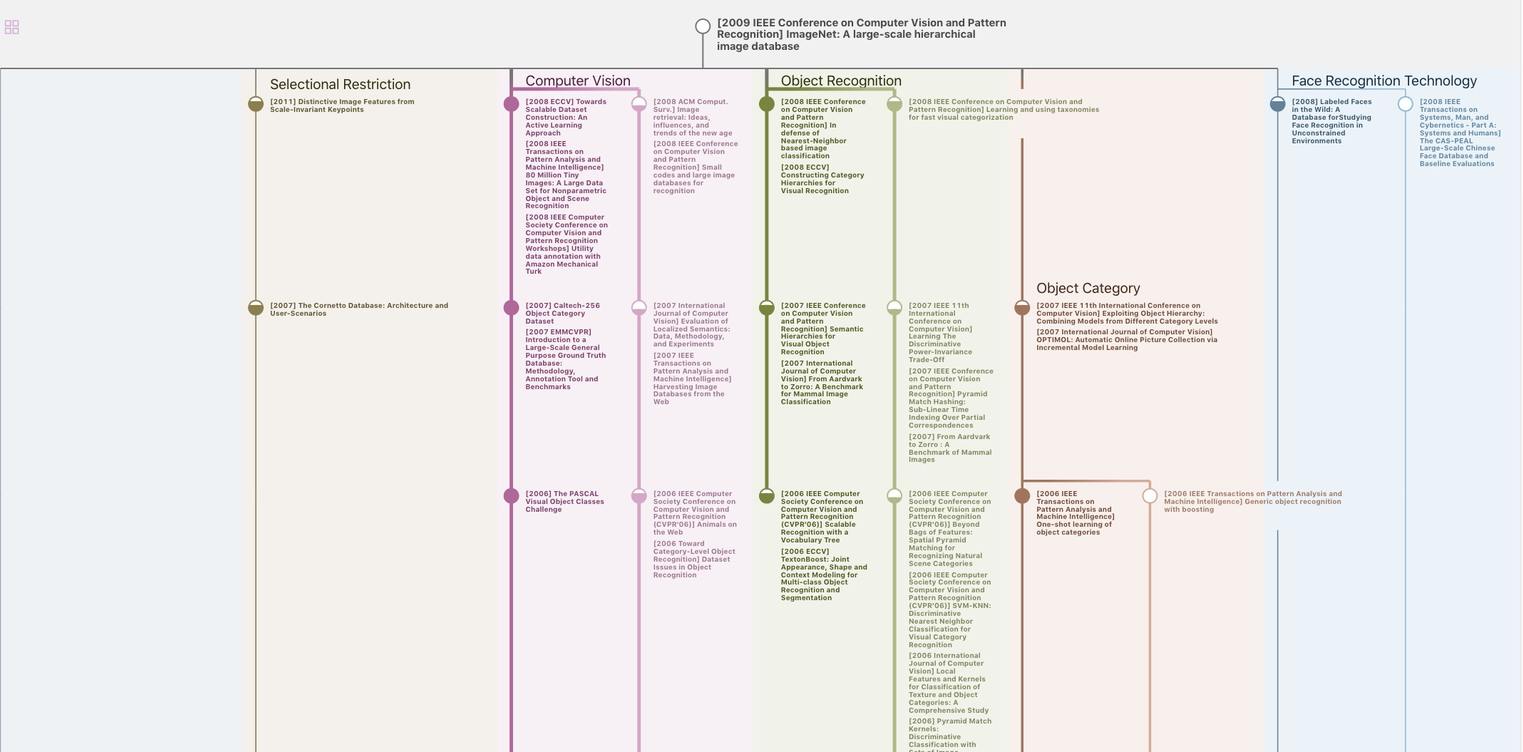
生成溯源树,研究论文发展脉络
Chat Paper
正在生成论文摘要