Functional Data Analysis for the Detection of Outliers and Study of the Effects of the COVID-19 Pandemic on Air Quality: A Case Study in Gijon, Spain
MATHEMATICS(2022)
Abstract
Air pollution, especially at the ground level, poses a high risk for human health as it can have serious negative effects on the population of certain areas. The high variability of this type of data, which are affected by weather conditions and human activities, makes it difficult for conventional methods to precisely detect anomalous values or outliers. In this paper, classical analysis, statistical process control, and functional data analysis are compared for this purpose. The results obtained motivate the development of a new outlier detector based on the concept of functional directional outlyingness. The validation of this algorithm is perfomed on real air quality data from the city of Gijon, Spain, aiming to detect the proven reduction in NO2 levels during the COVID-19 lockdown in that city. Three more variables (SO2, PM10, and O-3) are studied with this technique. The results demonstrate that functional data analysis outperforms the two other methods, and the proposed outlier detector is well suited for the accurate detection of outliers in data with high variability.
MoreTranslated text
Key words
functional data analysis,air pollution,magnitude outlyingness,shape outlyingness,COVID-19
AI Read Science
Must-Reading Tree
Example
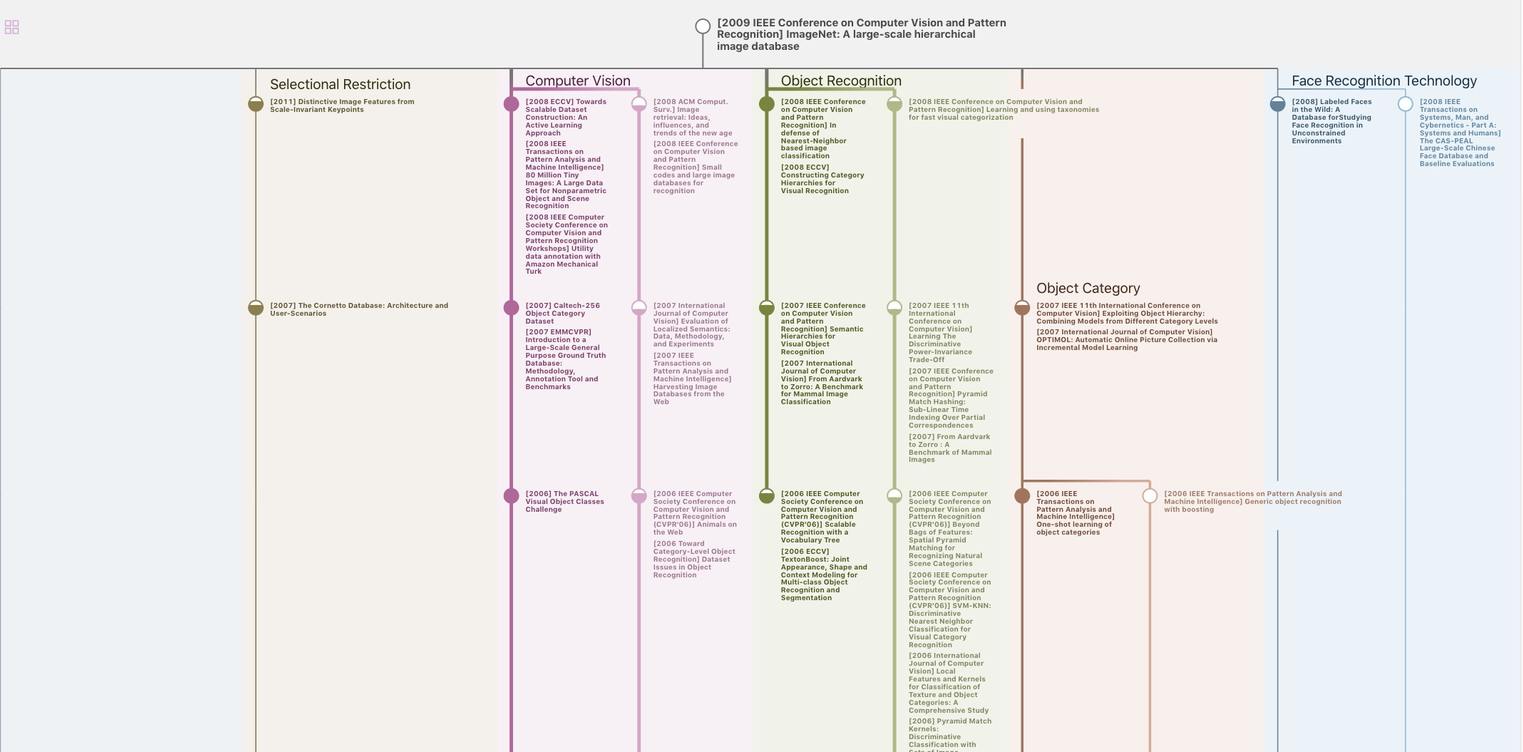
Generate MRT to find the research sequence of this paper
Chat Paper
Summary is being generated by the instructions you defined