On the Effectiveness of Pre-Trained Language Models for Legal Natural Language Processing: An Empirical Study
IEEE ACCESS(2022)
摘要
We present the first comprehensive empirical evaluation of pre-trained language models (PLMs) for legal natural language processing (NLP) in order to examine their effectiveness in this domain. Our study covers eight representative and challenging legal datasets, ranging from 900 to 57K samples, across five NLP tasks: binary classification, multi-label classification, multiple choice question answering, summarization and information retrieval. We first run unsupervised, classical machine learning and/or non-PLM based deep learning methods on these datasets, and show that baseline systems' performance can be 4%similar to 35% lower than that of PLM-based methods. Next, we compare general-domain PLMs and those specifically pre-trained for the legal domain, and find that domain-specific PLMs demonstrate 1%similar to 5% higher performance than general-domain models, but only when the datasets are extremely close to the pre-training corpora. Finally, we evaluate six general-domain state-of-the-art systems, and show that they have limited generalizability to legal data, with performance gains from 0.1% to 1.2% over other PLM-based methods. Our experiments suggest that both general-domain and domain-specific PLM-based methods generally achieve better results than simpler methods on most tasks, with the exception of the retrieval task, where the best-performing baseline outperformed all PLM-based methods by at least 5%. Our findings can help legal NLP practitioners choose the appropriate methods for different tasks, and also shed light on potential future directions for legal NLP research.
更多查看译文
关键词
Legal natural language processing,pre-trained language model,deep learning,machine learning
AI 理解论文
溯源树
样例
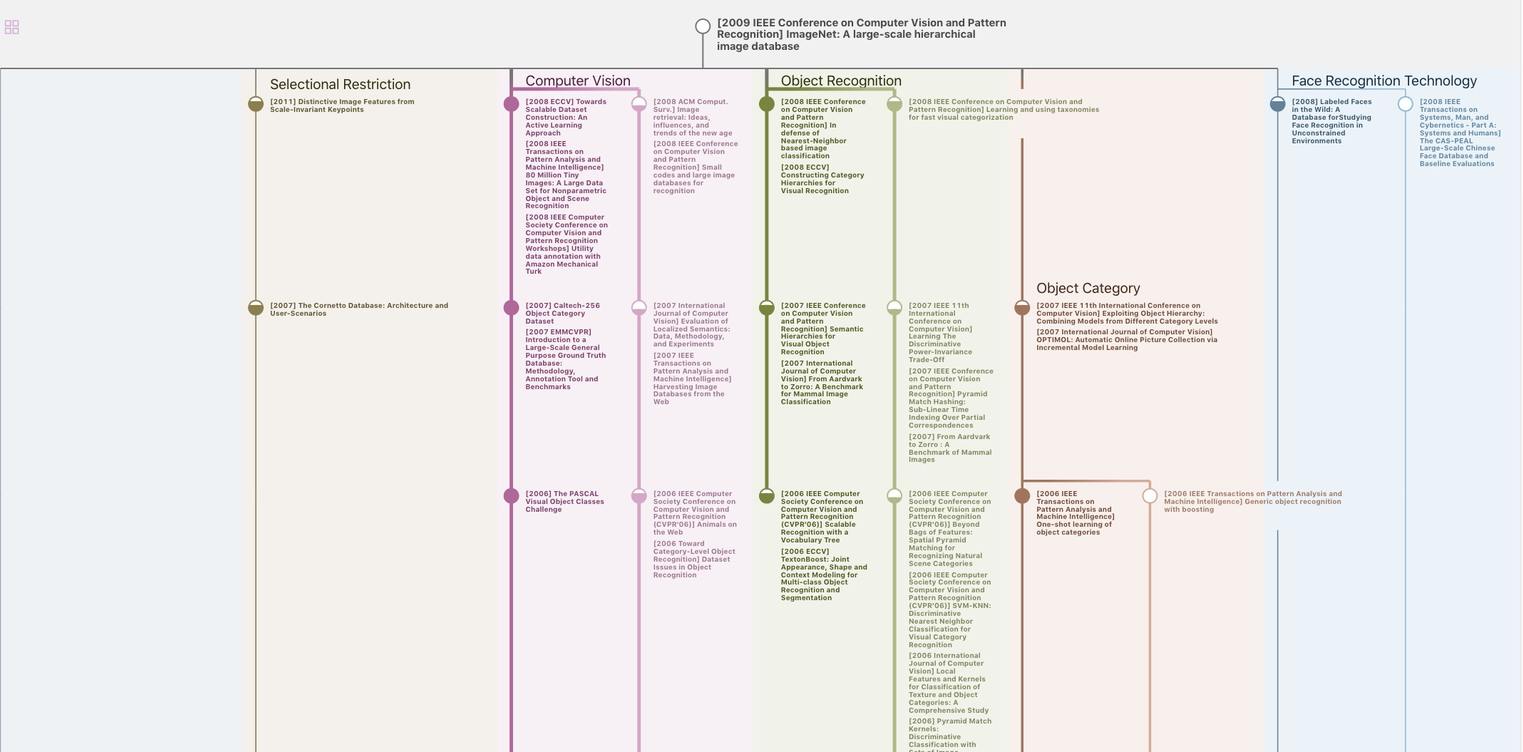
生成溯源树,研究论文发展脉络
Chat Paper
正在生成论文摘要