Accelerating Hydraulic Fracture Imaging by Deep Transfer Learning
IEEE TRANSACTIONS ON ANTENNAS AND PROPAGATION(2022)
摘要
Deep transfer learning has a great success story in computer vision (CV), natural language processing, and many other fields. In this communication, we are going to push forward the deep transfer learning to the hydraulic fracture imaging problem by proposing a two-step approach: 1) train a convolutional neural network (CNN) to reconstruct target geometries by a relatively large amount of approximated field patterns generated from a simplified model and 2) fine-tune the top layers of transferred CNN by a small amount of true field patterns generated through a full model. The advantages include the rapid generation of large amount of data through the simplified model and the high reconstruction accuracy through a careful design of the deep transfer learning. The CNN trained through the deep transfer learning can accurately reconstruct the lateral extent and direction of fractures with unseen conductivity and white Gaussian noise, showing a notable acceleration/accuracy enhancement over the previous CNN trained by mixing a small number of true data into the approximated data as data augmentation.
更多查看译文
关键词
Data models, Transfer learning, Computational modeling, Imaging, Convolutional neural networks, Hydraulic systems, Kernel, Convolutional neural networks (CNNs), deep transfer learning, hydraulic fracture imaging
AI 理解论文
溯源树
样例
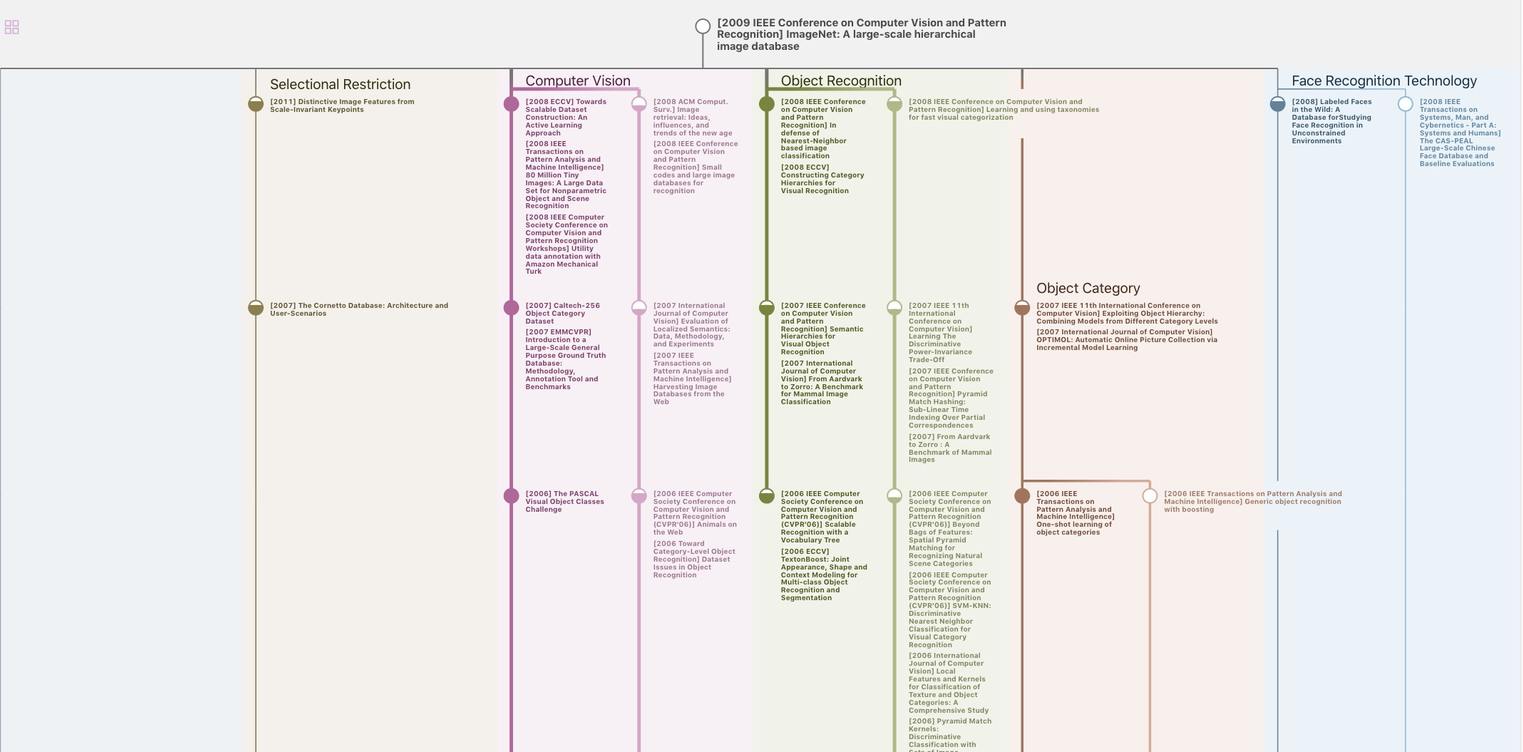
生成溯源树,研究论文发展脉络
Chat Paper
正在生成论文摘要