Automated chondrocyte viability analysis of articular cartilage based on deep learning segmentation and classification of two-photon microscopic images
THREE-DIMENSIONAL AND MULTIDIMENSIONAL MICROSCOPY: IMAGE ACQUISITION AND PROCESSING XXIX(2022)
摘要
Chondrocyte viability is an important measure to consider when assessing cartilage health. Dye-based cell viability assays are not suitable for in vivo or long-term studies. We have introduced a non-labeling viability assay based on the assessment of high-resolution images of cells and collagen structure using two-photon stimulated autofluorescence and second harmonic generation microscopy. By either the visual or quantitative assessment, we were able to differentiate living from dead chondrocytes in those images. However, both techniques require human participation and have limited throughputs. Throughput can be increased by using methods for automated cell-based image processing. Due to the poor image contrast, traditional image processing methods are ineffective on autofluorescence images produced by nonlinear microscopes. In this work, we examined chondrocyte segmentation and classification using Mask R-CNN, a deep learning approach to implement automated viability analysis. It has been demonstrated an 85% accuracy in chondrocyte viability assessment with proper training. This study demonstrates that automated and highly accurate image analysis is achievable with the use of deep learning methods. This image processing approach can be helpful to other imaging applications in clinical medicine and biological research.
更多查看译文
关键词
nonlinear optical microscopy, autofluorescence, second harmonic generation, deep learning, viability
AI 理解论文
溯源树
样例
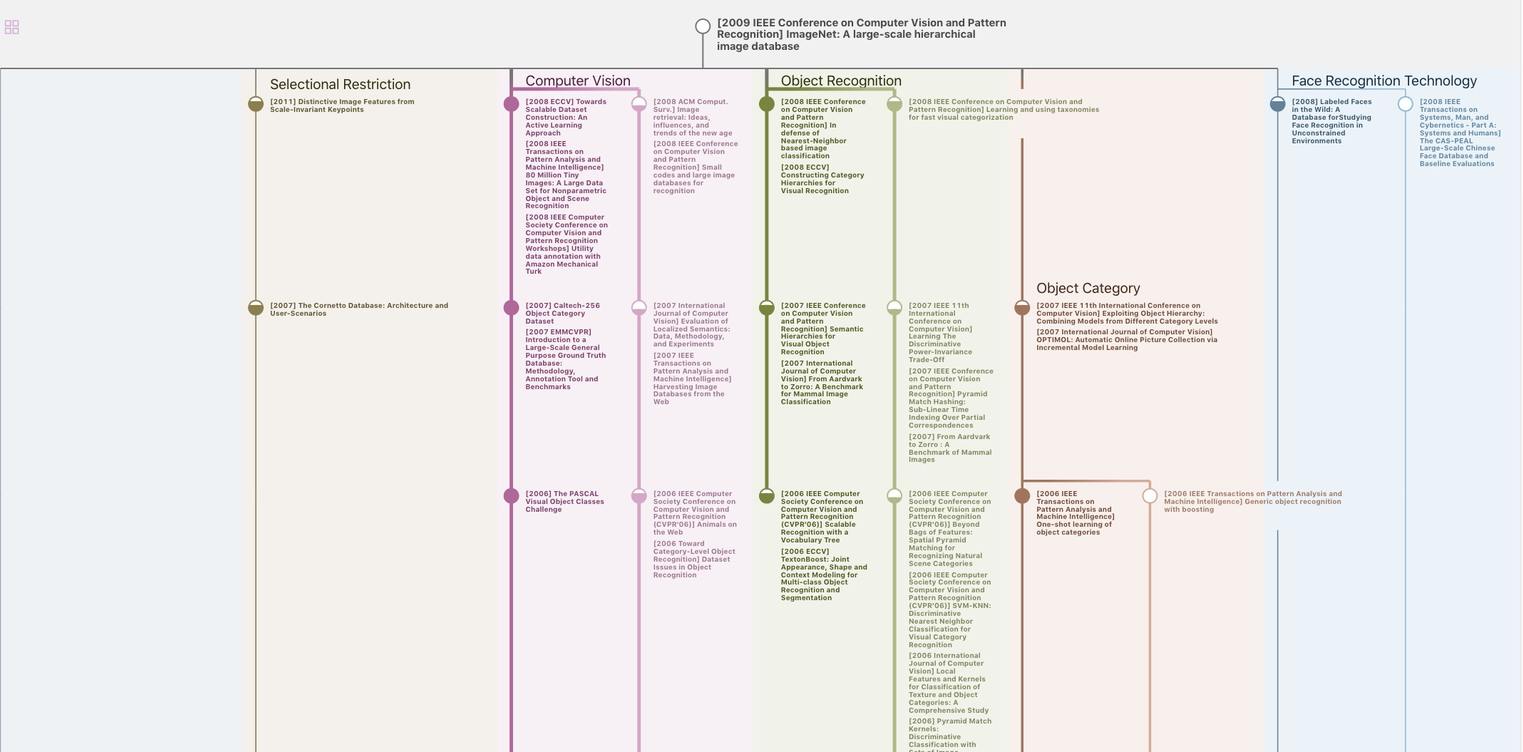
生成溯源树,研究论文发展脉络
Chat Paper
正在生成论文摘要