Fundus Image Screening for Diabetic Retinopathy br
CHINESE JOURNAL OF LASERS-ZHONGGUO JIGUANG(2022)
Abstract
ObjectiveDiabetesisaworldwidechronicdiseasewhichcancausechangesinvascularperformanceandresultincomplicationssuchasdiabeticretinopathyDRIfapatienthasDRanditgoesundetectedthepatient????seyesightmaybelostThereforeearlydetectionandearlytreatmentofDRisimportantforreducingtheblindnessrateinvariouscountriesAtpresenttheacquisitionoffundusimagesmainlydependsonnonmydriasiscolorfundusphotographywhichcanclearlycapturethesoftandhardexudatesbleedingpointsmicrovesselsandsoonCurrentlytheophthalmologistswhoobservefundusimageshavetoendureheavyworkloadsandmassivejob-relatedstressowingtoproblemssuchasinsufficientexpertresourcesToreducetheirworkloaditisnecessarytousecomputer-aideddiagnosisMoreovercomputer-aideddiagnosisismoreaccurateInthefieldofmedicalimagingusingclassicalmachinelearninganddeeplearningtoclassifymedicalimageshasbecomekeyresearchareasandgraduallythesubfieldofretinalfundusimageclassificationhasdevelopedHoweverthereisaseriousproblemwithmachinelearninganddeeplearningalgorithmsforfundusimageclassificationdevelopedbymanyresearchersthatistheycontinuouslyincreasethecomplexityofthemodeltopursuehighaccuracyThisresultsinacorrespondingincreaseincomputationalcomplexityintermsofthenumberoffloating-pointoperationsandthesizeoftheparametersofthenetworkmodelthusreducingthespeedandincreasingthememoryutilizationAninefficientclassificationmodelislesslikelytobeusedinclinicalpracticeThepurposeofthispaperistoproposeasimplenetworkmodelComparedwiththemostadvancedmodelourmodelhasnotonlyhighaccuracyprecisionandsensitivitybutalsohighspeedMoreimportantlyithasthepotentialtobeusedinclinicalpracticeMethodsWeimprovedthenetworkarchitectureoftheRepVGGmodelproposedbytheKuangshi????sgroupandproposedanovelmodelChannelAttention-RepVGGCA-RepVGGTheRepVGGwithsimplestructurewasusedtoreplacethecomplexmoduleasthemainpartoftheclassificationmodelandefficientchannelattentionwasselectedtoreplacethesqueeze-and-excitationSEforagoodclassificationofimagesrelatedtoDRThenCA-RepVGGwastestedonthenewdatasetofDRimagesThemainresearchincludesthefollowingFirstthemultibrancharchitectureemployedduringtrainingandthesingle-brancharchitectureemployedduringinferenceweredecoupledusingthestructuralreparameterizationmethodwhichgreatlyreducedthecomplexityofthemodelandmettherequirementsofsimplicityinstructureSecondanewlightweightattentionmodulewasusedtoimprovetheperformanceoftheconvolutionalneuralnetworkandenhancetheabilityoffeatureextractionfromretinalfundusimagesFinallytheparametersspeedprecisionaccuracyandsensitivityofseveralclassicalnetworksinimageclassificationwerecomparedResultsandDiscussionsTheproposedmodelistestedon1096picturesofdataset1and500picturesofdataset2Theaccuracyofdataset1is924%theprecisionis916%andthesensitivityis965%Fordataset2theaccuracyis939%theprecisionis963%andthesensitivityis938%Theconfusionmatrixofdataset1isshowninFig6andthatofdataset2isshowninFig7ThetwofiguresshowtheclassificationresultsofthepicturesthevaluesonthediagonalpresentthenumberofpatientscorrectlyclassifiedbythemodelOnlyafewimageshaveerrorstheoverdiagnosisdistributesonthelowerleftofthediagonalintheimageandthemisseddiagnosisdistributesontheupperrightofthediagonalTheconfusionmatricesshowthesuperiorityoftheproposedmodelFurthermoreCA-RepVGGhasthefastestspeedalthoughithasthelargestparametersinthecomparedmodelsOwingtothesingle-brancharchitectureand3x3convolutionourmodelisnotcomplexTheCA-RepVGGcanprocess415picturespersecondwhichis153%higherthanthosebyResNet-50ConclusionsCA-RepVGGcanbeusedinclinicalpracticeThesimplicityofthemodelandthesmallamountof calculationensurethefeasibilityandreliabilityofCA-RepVGGInthispaperCA-RepVGGisusedtotest andevaluatetheclassificationeffectofDRi magesintwodatasetsAtthesametimeVGG-16Inception-V3ResNet-50andResNext-50arecomparedwithourmodelandtheaccuracyprecisionandsensitivityofthenetworkdemonstratetheadvancednatureofourmodelTheexperimentalresultsshowthatthemodelisnotonlyfeasiblebutalsosuperiorinclassificationInthefutureifourproposedmodelisappliedtoclinicalpracticeitcanenhancethediagnosticefficiencyofprofessionalophthalmologistsregardingophthalmicdiseasesespeciallyinremoteandpoorareasensuringthatmorepatientscanbetreatedintimeandavoidlosingtheireyesightIfmoredatasetscanbeusedtotrainthemodelinthefuturetheaccuracyofautomaticclassificationcanbefurtherenhancedandbetterresultscanbeachievedinclinicalpractice
MoreTranslated text
Key words
medical optics, ophthalmology, grading of diabetic retinopathy, fundus camera, deep learning, fundus image, automatic detection
AI Read Science
Must-Reading Tree
Example
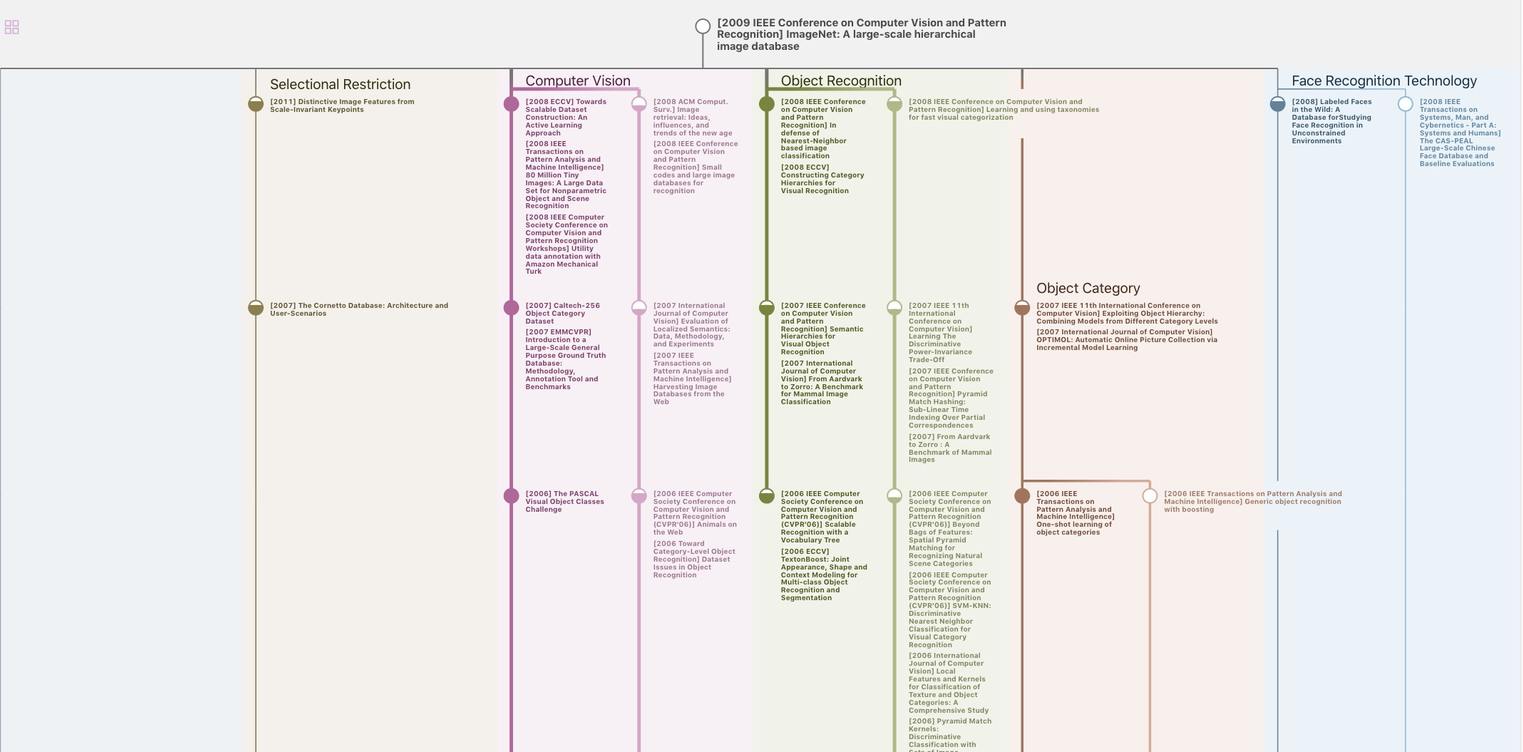
Generate MRT to find the research sequence of this paper
Chat Paper
Summary is being generated by the instructions you defined