Res-attention net: an unsupervised PET - MRI brain image fusion model
MULTIMEDIA TOOLS AND APPLICATIONS(2022)
摘要
PET-MRI fusion images are of great significance in improving diagnostic accuracy and nursing quality. However, existing fusion methods usually subjectively define fusion rules to retain specific fusion regions, which will lead to information distortion. Complex processes or network design can also significantly slow down fusion speed. The fusion results of unsupervised models without artificially setting fusion rules are usually poor. To address these limitations, we designed an unsupervised model to accomplish the fusion of MRI-PET brain images. We use the attention modules CBAM (convolutional block attention module) and AG (attention gate) to dynamically highlight the salient features, and the ASPP (arous spatial pyramid pooling) module and residual structure are used to maximize the preservation of source image information. The unique design of each module saves us a lot of computational costs. In addition, we designed a fusion strategy for separating the bone density information and the fusion region, which solves the problem of MRI bone density information retention during image fusion. We have done lots of experiments to verify the superiority of the model. In comparison with the state-of-the-art methods, our model has a higher Q G than FusionDN at 68.9% and a Q s than U2Fusion at 120.49%, and the fusion efficiency has also reached a high level.
更多查看译文
关键词
Image fusion,Convolutional block attention module,Attention gate,Atrous spatial pyramid pooling
AI 理解论文
溯源树
样例
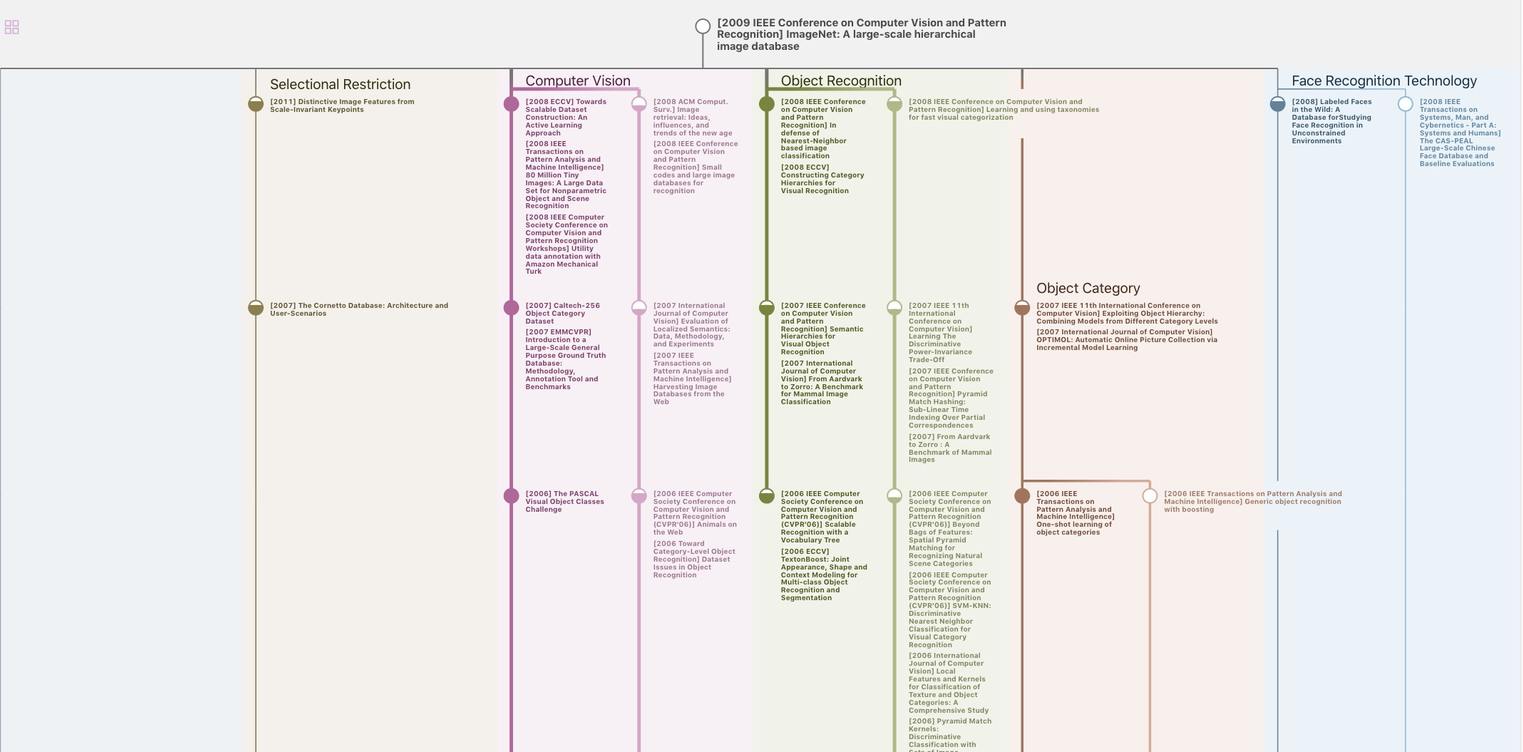
生成溯源树,研究论文发展脉络
Chat Paper
正在生成论文摘要