Meta-Learning-Based Proactive Online Planning for UAVs Under Degraded Conditions
IEEE ROBOTICS AND AUTOMATION LETTERS(2022)
摘要
Changes in model dynamics due to factors like actuator faults, platform aging, and unexpected disturbances can challenge an autonomous robot during real-world operations affecting its intended behavior and safety. Under such circumstances, it becomes critical to improve tracking performance, predict future states of the system, and replan to maintain safety and liveness conditions. In this letter, we propose a meta-learning-based framework to learn a model to predict the future system's states and their uncertainties under unforeseen and untrained conditions. Meta-learning is considered for this problem thanks to its ability to easily adapt to new tasks with a few data points gathered at runtime. We use the predictions from the meta-learned model to detect unsafe situations and proactively replan the system's trajectory when an unsafe situation is detected (e.g., a collision with an object). The proposed framework is applied and validated with both simulations and experiments on a faulty UAV performing an infrastructure inspection mission, demonstrating safety improvements.
更多查看译文
关键词
Planning under uncertainty,failure detection and recovery,aerial systems
AI 理解论文
溯源树
样例
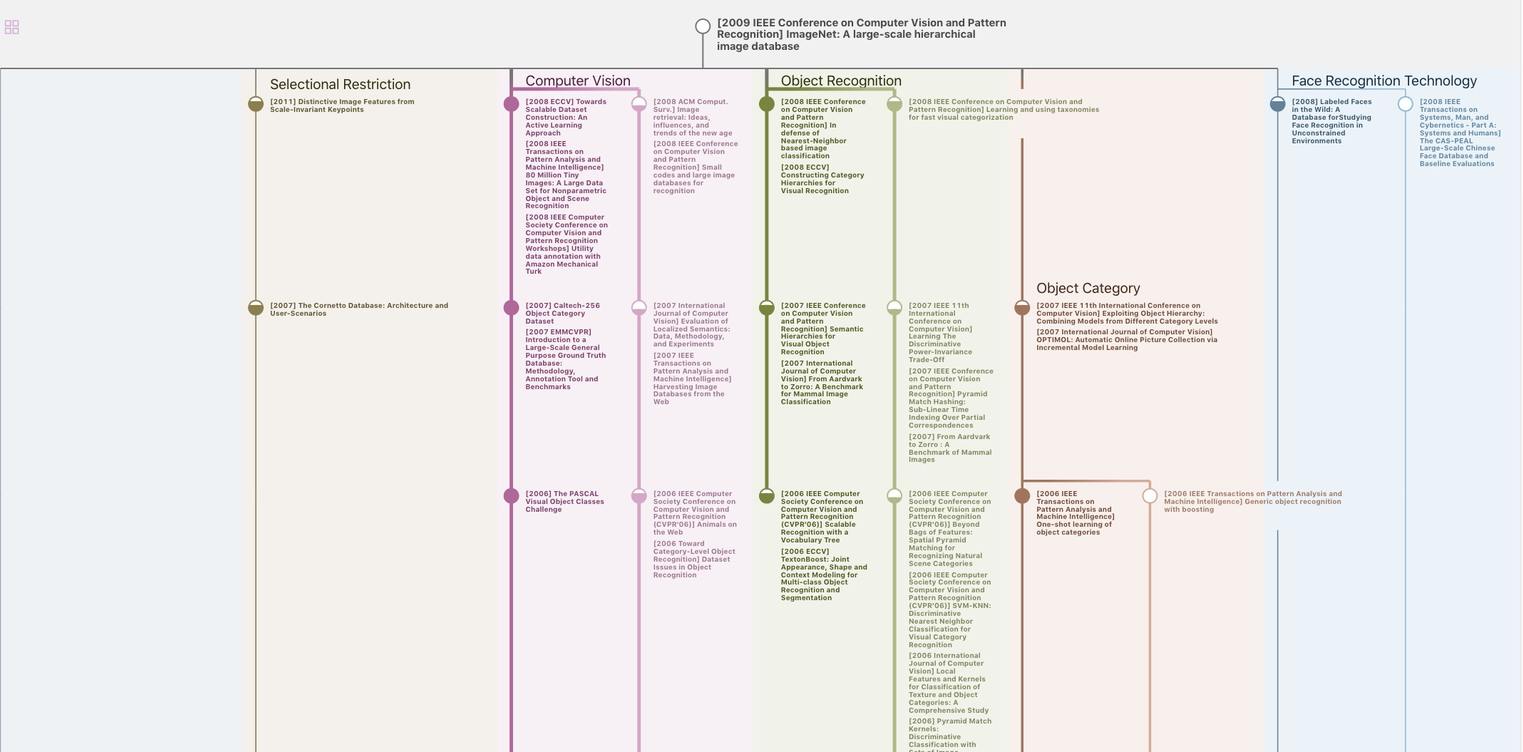
生成溯源树,研究论文发展脉络
Chat Paper
正在生成论文摘要