A radiomics-based study for differentiating parasellar cavernous hemangiomas from meningiomas
SCIENTIFIC REPORTS(2022)
摘要
To investigate the value of the radiomic models for differentiating parasellar cavernous hemangiomas from meningiomas and to compare the classification performance with different MR sequences and classifiers. A total of 96 patients with parasellar tumors (40 cavernous hemangiomas and 56 meningiomas) were enrolled in this retrospective multiple-center study. Univariate and multivariate analyses were performed to identify the clinical factors and semantic features of MRI scans. Radiomics features were extracted from five MRI sequences using radiomics software. Three feature selection methods and six classifiers were evaluated in the training cohort to construct favorable radiomic machine-learning classifiers. The performance of different classifiers was evaluated using the AUC and compared to neuroradiologists. The detection rates of T 1 WI, T 2 WI, and CE-T 1 WI for parasellar cavernous hemangiomas and meningiomas were approximately 100%. In contrast, the ADC maps had the detection rate of 18/22 and 19/25, respectively, (AUC, 0.881) with 2.25 cm as the critical value diameter. Radiomics models with the SVM and KNN classifiers based on T 2 WI and ADC maps had favorable predictive performances (AUC > 0.90 and F-score value > 0.80). These models outperformed MRI model (AUC 0.805) and neuroradiologists (AUC, 0.756 and 0.545, respectively). Radiomic models based on T 2 WI and ADC and combined with SVM and KNN classifiers have the potential to be a viable method for differentiating parasellar hemangiomas from meningiomas. T 2 WI is more universally applicable than ADC values due to its higher detection rate for parasellar tumors.
更多查看译文
关键词
CNS cancer,Clinical trial design,Science,Humanities and Social Sciences,multidisciplinary
AI 理解论文
溯源树
样例
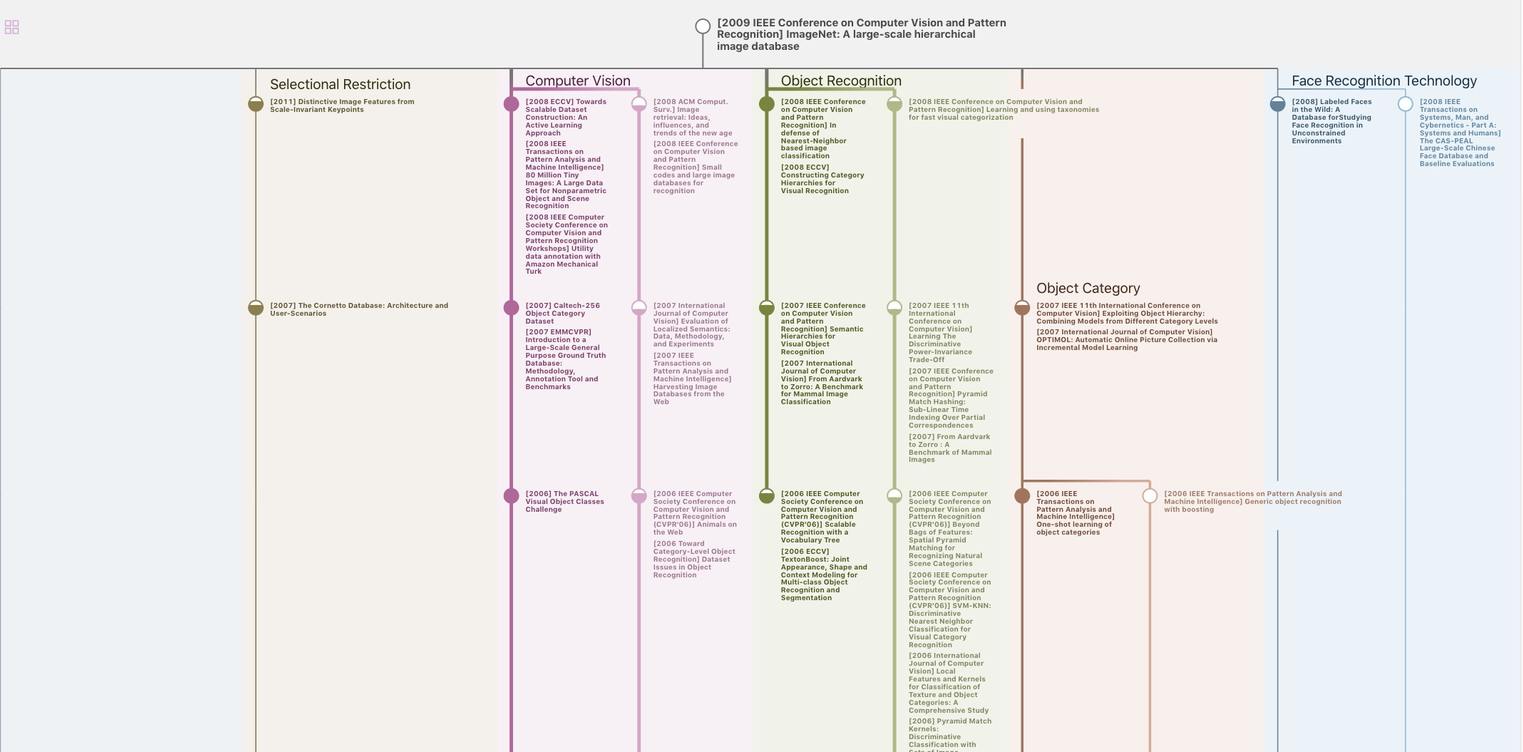
生成溯源树,研究论文发展脉络
Chat Paper
正在生成论文摘要