Lossy Image Compression with Conditional Diffusion Models
arXiv (Cornell University)(2022)
摘要
Diffusion models are a new class of generative models that mark a milestone in high-quality image generation while relying on solid probabilistic principles. This makes them promising candidate models for neural image compression. This paper outlines an end-to-end optimized framework based on a conditional diffusion model for image compression. Besides latent variables inherent to the diffusion process, the model introduces an additional per-instance "content" latent variable to condition the denoising process. Upon decoding, the diffusion process conditionally generates/reconstructs an image using ancestral sampling. Our experiments show that this approach outperforms one of the best-performing conventional image codecs (BPG) and one neural codec on two compression benchmarks, where we focus on rate-perception tradeoffs. Qualitatively, our approach shows fewer decompression artifacts than the classical approach.
更多查看译文
关键词
conditional diffusion models,image
AI 理解论文
溯源树
样例
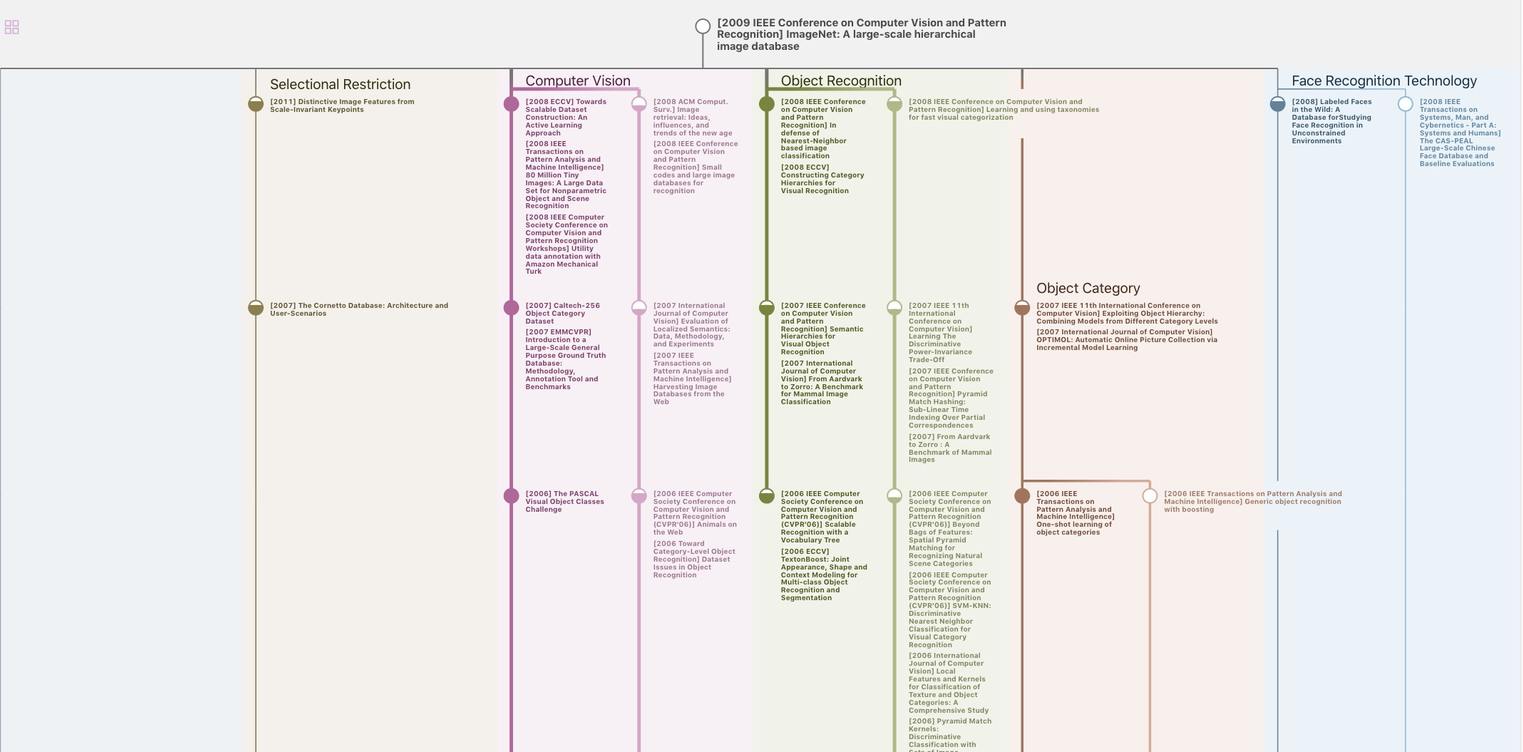
生成溯源树,研究论文发展脉络
Chat Paper
正在生成论文摘要