Viewer-Centred Surface Completion for Unsupervised Domain Adaptation in 3D Object Detection
2023 IEEE INTERNATIONAL CONFERENCE ON ROBOTICS AND AUTOMATION (ICRA 2023)(2023)
关键词
3D object detection,autonomous driving dataset,distinct geographic regions,domain-invariant representation,drastic decrease,lidar scan pattern differences,multiple domain adaptation settings,multitarget domain adaptation approach,novel viewer-centred surface completion network,previous domain adaptation methods,scan patterns,unsupervised domain adaptation framework
AI 理解论文
溯源树
样例
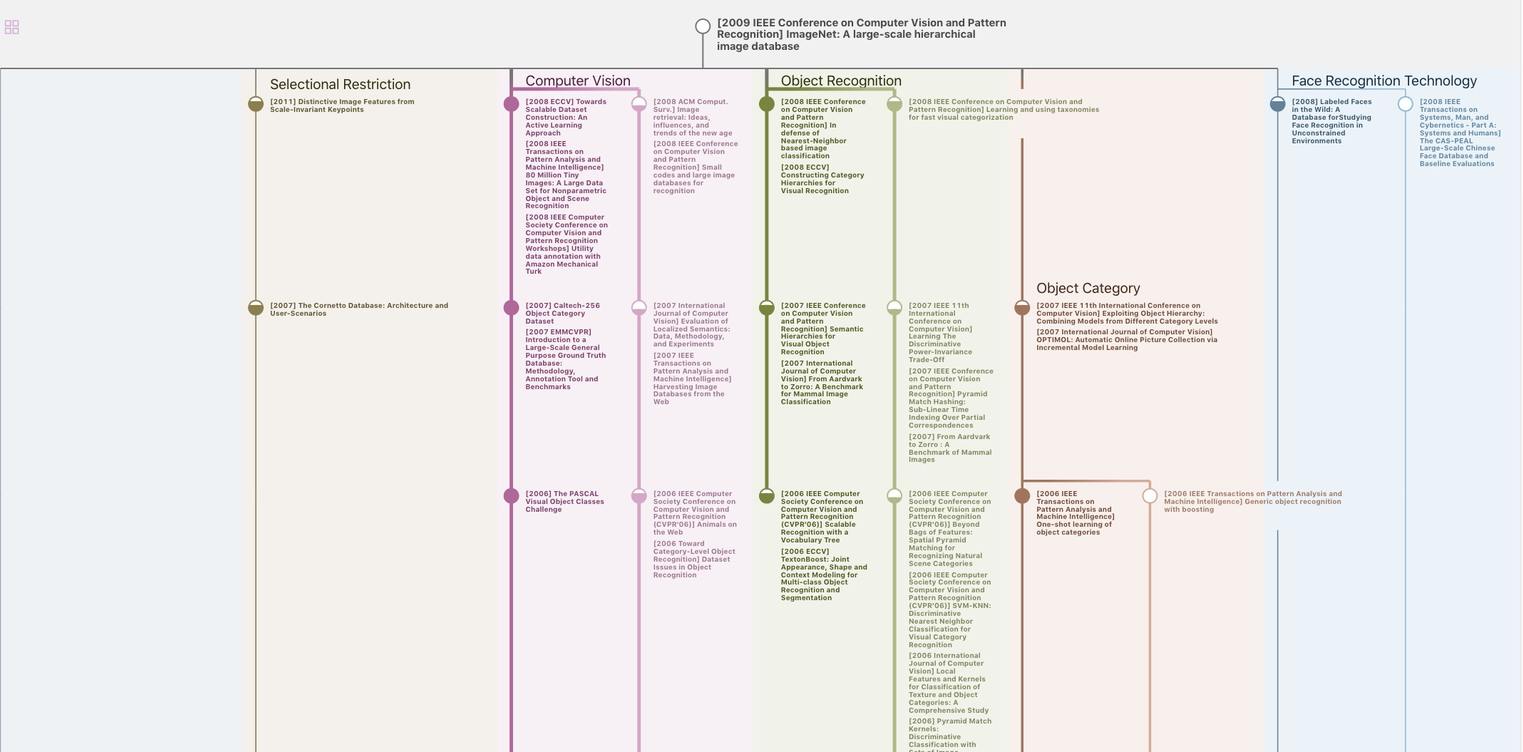
生成溯源树,研究论文发展脉络
Chat Paper
正在生成论文摘要