Deformable residual attention network for defect detection of train wheelset tread
The Visual Computer(2024)
摘要
The wheelset tread has large noise interference and various forms of defects, resulting in the low detection accuracy and poor localization effect. We proposed a new detection model for wheel tread defect detection. First, we proposed a deformable residual attention network that combines deformable residual network modules and Channel-Fusion-Correct-Attention modules. The deformable residual network modules are to strengthen the capability of feature extraction for various forms of defects. While the Channel-Fusion-Correct-Attention modules can weaken noise interference. Second, adopt the path aggregation network feature fusion method combining top-down and bottom-up to reduce the loss of defect feature. Third, a deformable double-branch detection head is constructed to further optimize the overall performance of the model. A dataset of defects in the wheelset treads of trains was formulated for experiments to verify the performance of the model. The experiment results showed that the model could achieve accuracy of 78.2% and Recall of up to 91% when the IOU threshold was 0.5. It could detect 24.1 images per second on a single GPU, with 35.2 M parameters. The performance of the model was higher than classical models, such as Faster-RCNN and YOLO. The proposed method can be applied to the detection of defects on the tread surface of the wheelset.
更多查看译文
关键词
Deep learning,Defect detection,Wheelset tread,Convolution network,Deformable convolution network
AI 理解论文
溯源树
样例
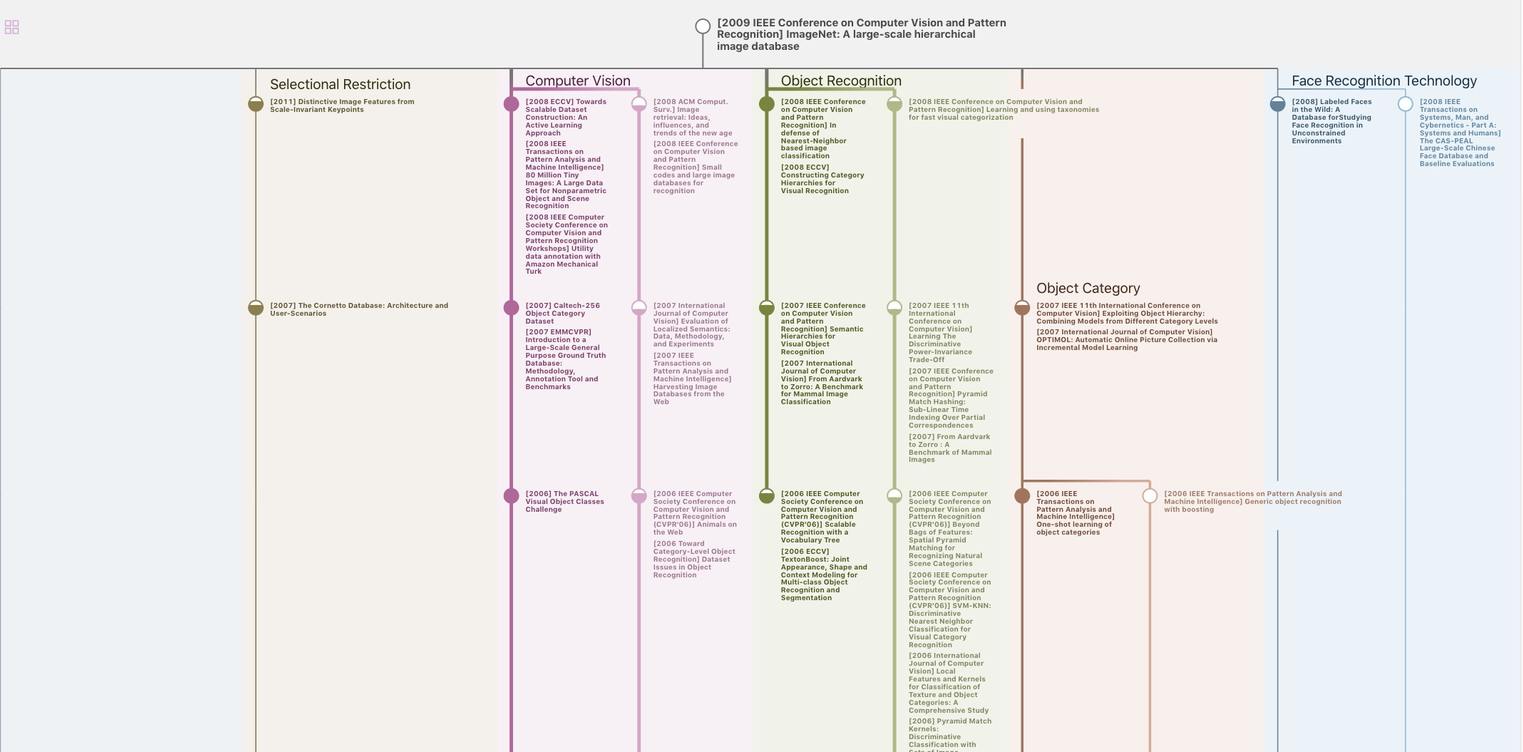
生成溯源树,研究论文发展脉络
Chat Paper
正在生成论文摘要