Stratiform and Convective Rain Classification Using Machine Learning Models and Micro Rain Radar
REMOTE SENSING(2022)
摘要
Rain type classification into convective and stratiform is an essential step required to improve quantitative precipitation estimations by remote sensing instruments. Previous studies with Micro Rain Radar (MRR) measurements and subjective rules have been performed to classify rain events. However, automating this process by using machine learning (ML) models provides the advantages of fast and reliable classification with the possibility to classify rain minute by minute. A total of 20,979 min of rain data measured by an MRR at Das in northeast Spain were used to build seven types of ML models for stratiform and convective rain type classification. The proposed classification models use a set of 22 parameters that summarize the reflectivity, the Doppler velocity, and the spectral width (SW) above and below the so-called separation level (SL). This level is defined as the level with the highest increase in Doppler velocity and corresponds with the bright band in stratiform rain. A pre-classification of the rain type for each minute based on the rain microstructure provided by the collocated disdrometer was performed. Our results indicate that complex ML models, particularly tree-based ensembles such as xgboost and random forest which capture the interactions of different features, perform better than simpler models. Applying methods from the field of interpretable ML, we identified reflectivity at the lowest layer and the average spectral width in the layers below SL as the most important features. High reflectivity and low SW values indicate a higher probability of convective rain.
更多查看译文
关键词
Micro Rain Radar (MRR),disdrometer,convective,stratiform,classification,machine learning,random forest,xgboost,decision trees,support vector machines,naive Bayes
AI 理解论文
溯源树
样例
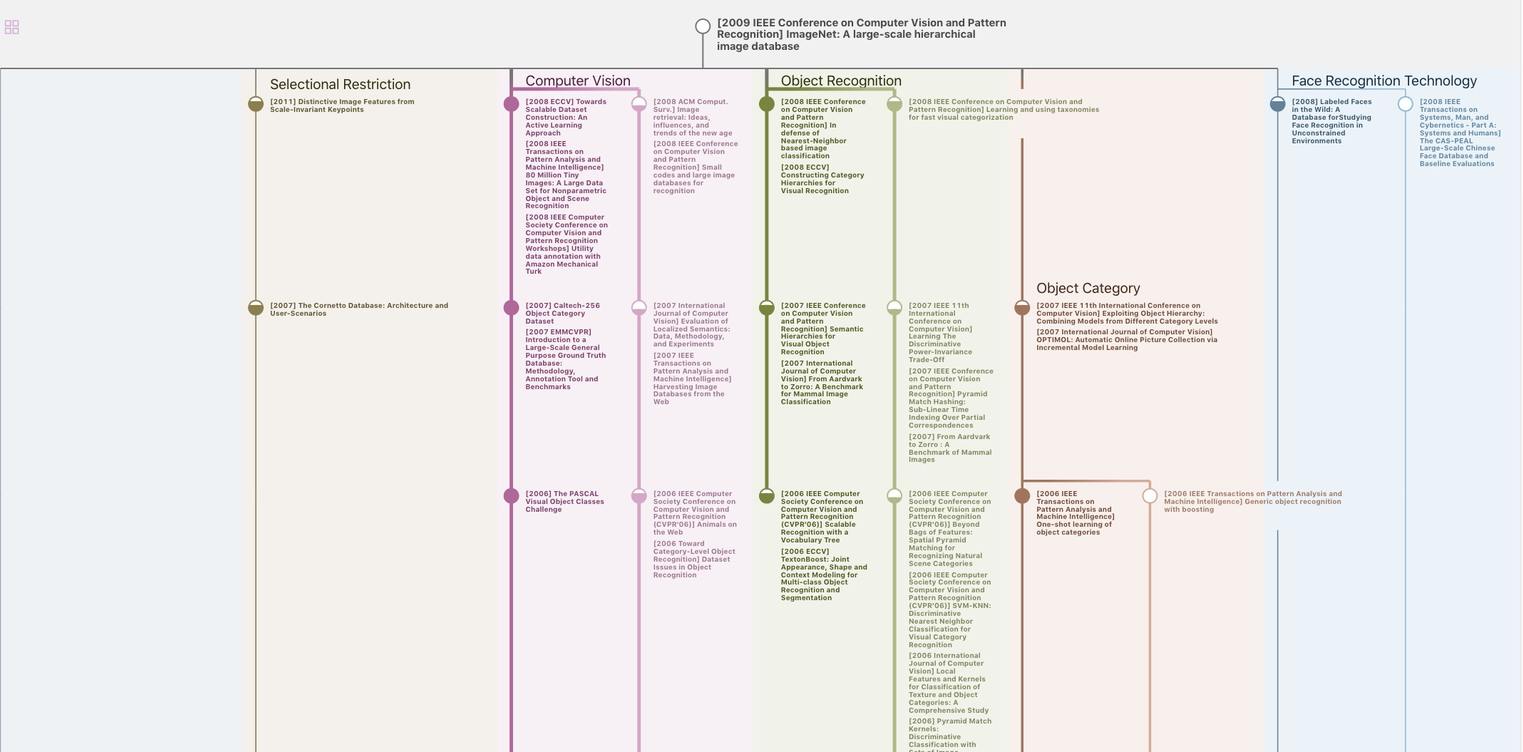
生成溯源树,研究论文发展脉络
Chat Paper
正在生成论文摘要