Entity Tagging: Extracting Entities in Text Without Mention Supervision
arXiv (Cornell University)(2022)
摘要
Detection and disambiguation of all entities in text is a crucial task for a wide range of applications. The typical formulation of the problem involves two stages: detect mention boundaries and link all mentions to a knowledge base. For a long time, mention detection has been considered as a necessary step for extracting all entities in a piece of text, even if the information about mention spans is ignored by some downstream applications that merely focus on the set of extracted entities. In this paper we show that, in such cases, detection of mention boundaries does not bring any considerable performance gain in extracting entities, and therefore can be skipped. To conduct our analysis, we propose an "Entity Tagging" formulation of the problem, where models are evaluated purely on the set of extracted entities without considering mentions. We compare a state-of-the-art mention-aware entity linking solution against GET, a mention-agnostic sequence-to-sequence model that simply outputs a list of disambiguated entities given an input context. We find that these models achieve comparable performance when trained both on a fully and partially annotated dataset across multiple benchmarks, demonstrating that GET can extract disambiguated entities with strong performance without explicit mention boundaries supervision.
更多查看译文
关键词
entity tagging,mention supervision,entities,text
AI 理解论文
溯源树
样例
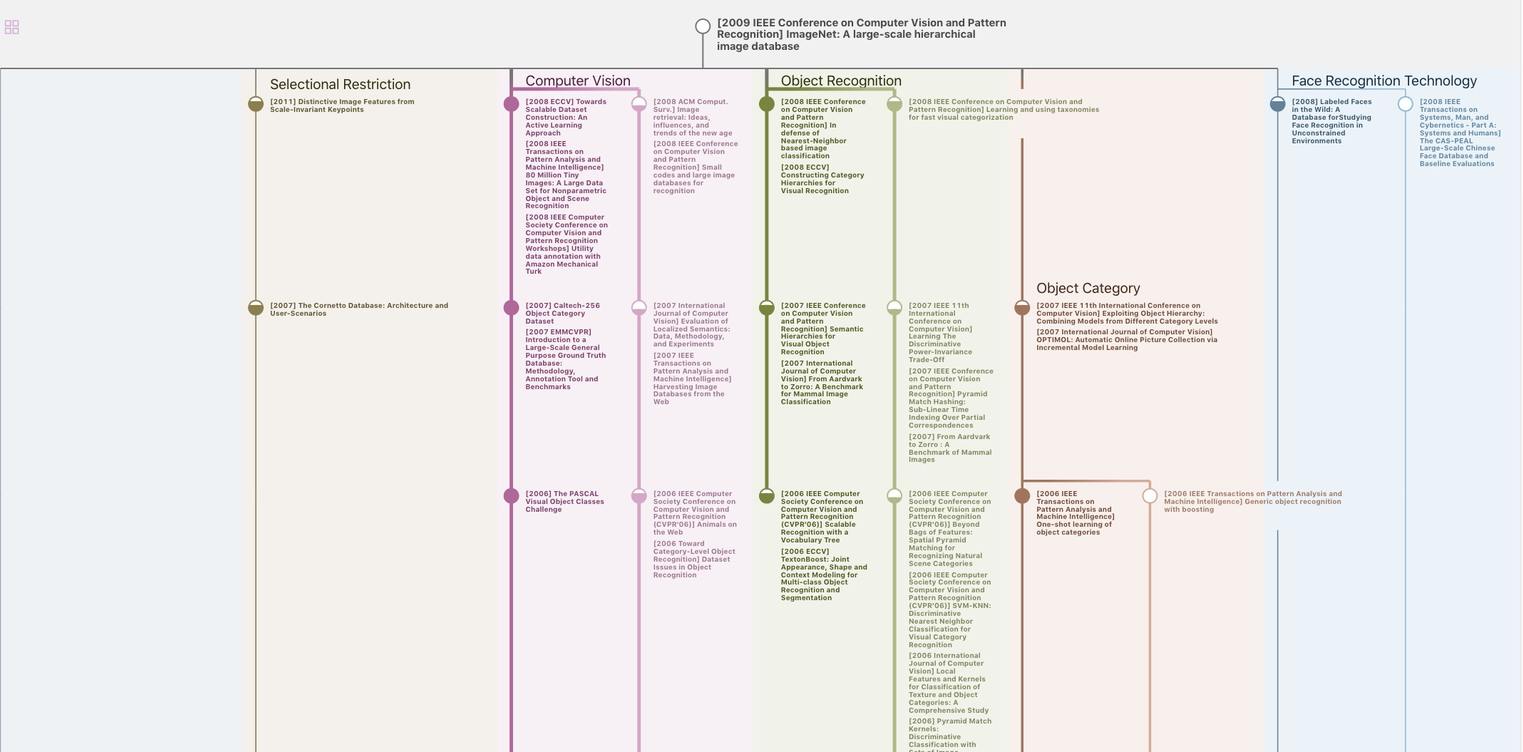
生成溯源树,研究论文发展脉络
Chat Paper
正在生成论文摘要