YOLOv5-Tassel: Detecting Tassels in RGB UAV Imagery With Improved YOLOv5 Based on Transfer Learning
IEEE JOURNAL OF SELECTED TOPICS IN APPLIED EARTH OBSERVATIONS AND REMOTE SENSING(2022)
摘要
Unmanned aerial vehicles (UAVs) equipped with lightweight sensors, such as RGB cameras and LiDAR, have significant potential in precision agriculture, including object detection. Tassel detection in maize is an essential trait given its relevance as the beginning of the reproductive stage of growth and development of the plants. However, compared with general object detection, tassel detection based on RGB imagery acquired by UAVs is more challenging due to the small size, time-dependent variable shape, and complexity of the objects of interest. A novel algorithm referred to as YOLOv5-tassel is proposed to detect tassels in UAV-based RGB imagery. A bidirectional feature pyramid network is adopted for the path-aggregation neck to effectively fuse cross-scale features. The robust attention module of SimAM is introduced to extract the features of interest before each detection head. An additional detection head is also introduced to improve small-size tassel detection based on the original YOLOv5. Annotation is performed with guidance from center points derived from CenterNet to improve the selection of the bounding boxes for tassels. Finally, to address the issue of limited reference data, transfer learning based on the VisDrone dataset is adopted. Testing results for our proposed YOLOv5-tassel method achieved the mAP value of 44.7%, which is better than well-known object detection approaches, such as FCOS, RetinaNet, and YOLOv5.
更多查看译文
关键词
Feature extraction, Object detection, Head, Agriculture, Neck, Deep learning, Transfer learning, CenterNet, SimAM attention module, small tassel detection, transfer learning, YOLOv5
AI 理解论文
溯源树
样例
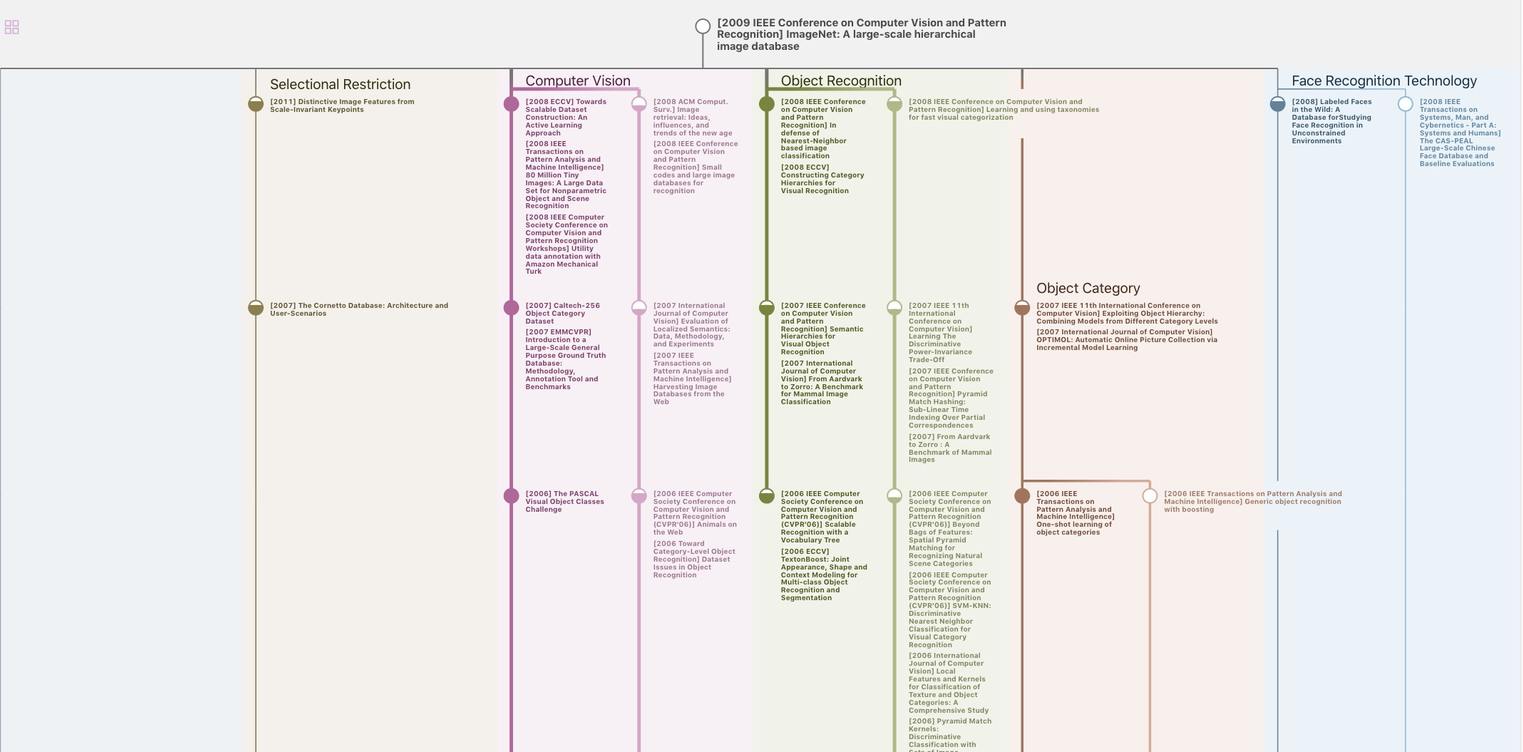
生成溯源树,研究论文发展脉络
Chat Paper
正在生成论文摘要