Upscale versus ‘up-amplitude’ growth of forecast-error spectra
Journal of the Atmospheric Sciences(2022)
摘要
Abstract Atmospheric predictability is measured by the average difference (or ‘error’) within an ensemble of forecasts starting from slightly different initial conditions. The spatial scale of the error field is a fundamental quantity; for meteorological applications, the error field typically varies with latitude and longitude and so requires a two-dimensional (2D) spectral analysis. Statistical predictability theory is based on the theory of homogeneous, isotropic turbulence, in which spectra are circularly symmetric in 2D wavenumber space. One takes advantage of this circular symmetry to reduce 2D spectra to one-dimensional (1D) spectra by integrating around a circle in wavenumber polar coordinates. In recent studies it has become common to reduce 2D error spectra to 1D by computing spectra in the zonal direction and then averaging the results over latitude. It is shown here that such 1D error spectra are generically fairly constant across the low wavenumbers as the amplitude of error spectra grows with time and therefore the error spectrum is said grow ‘up-amplitude’. In contrast computing the 1D error spectra in a manner consistent with statistical predictability theory gives spectra that are peaked at intermediate wavenumbers. In certain cases, this peak wavenumber is decreasing with time as the error at that wavenumber increases and therefore the error spectrum is said to grow ‘upscale’. We show through theory, simple examples and global predictability experiments that comparisons of model error spectra with the predictions of statistical predictability theory are only justified when using a theory-consistent method to transform a 2D error field to a 1D spectrum.
更多查看译文
关键词
Error analysis,Fourier analysis,Numerical weather prediction,forecasting,Diagnostics
AI 理解论文
溯源树
样例
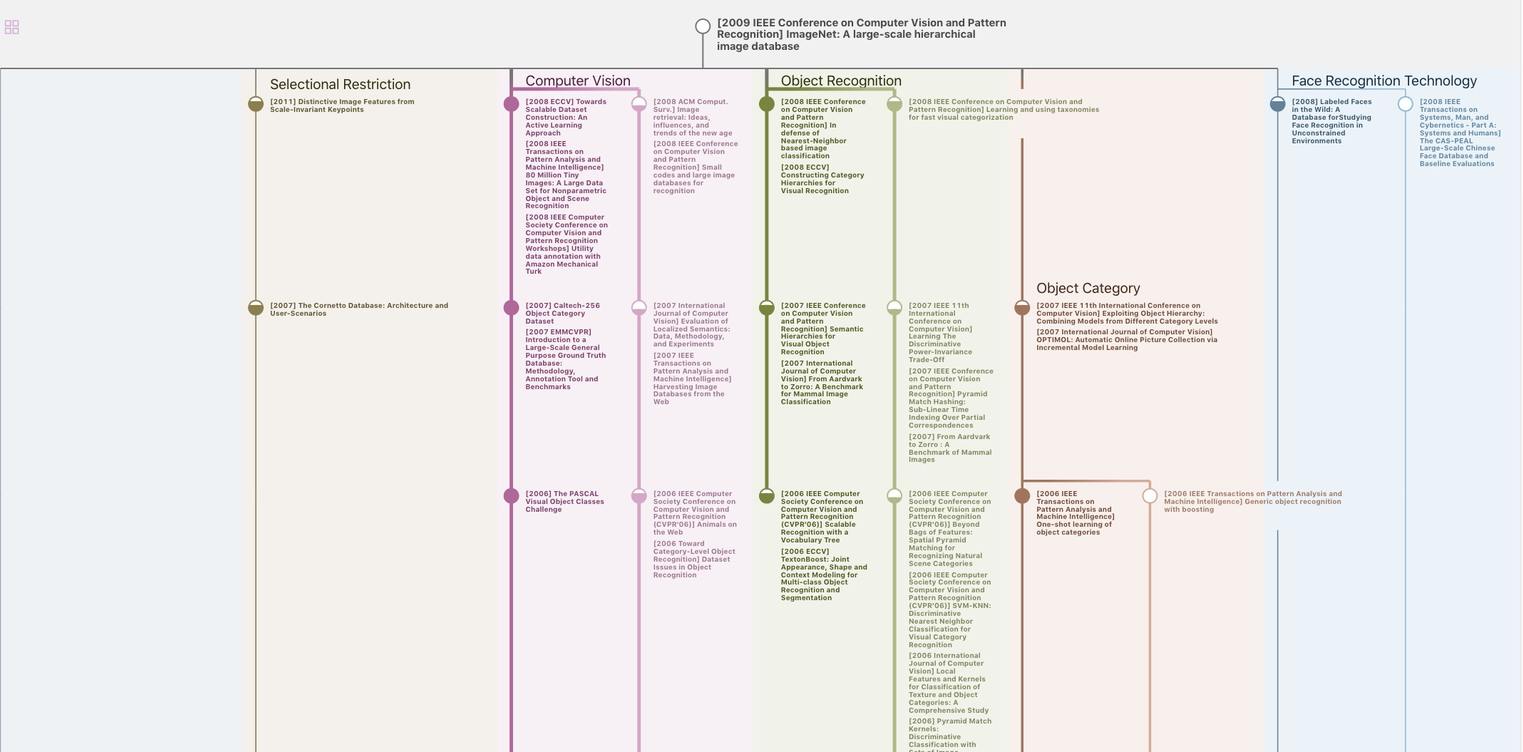
生成溯源树,研究论文发展脉络
Chat Paper
正在生成论文摘要